Sensor fault detection of vehicle suspension systems based on transmissibility operators and Neyman–Pearson test
Reliability Engineering & System Safety(2023)
摘要
As sensors become increasingly important and popular in vehicle suspension systems for control and monitoring purposes, the sensor faults endanger the reliability and safety of suspension systems and have raised broad concerns. However, due to the complex structures and unknown inputs of suspension systems, it is difficult to detect sensor faults using purely physics-based or data-driven approaches. This paper proposes a Physics-Informed Machine Learning (PIML) method, combining a residual generation procedure based on the transmissibility operators and a residual evaluation procedure based on the Neyman–Pearson test, to detect sensor faults in suspension systems as early as possible: First, transmissibilities are derived from the physical models to describe the relationship between the outputs of an underlying system; then, noncausal Finite Impulse Response (FIR) models are used to approximate the transmissibilities to generate the model residuals; finally, the generated residuals from noncausal FIR models are evaluated using the Neyman–Pearson test to detect the sensor faults, along with the analysis of the fault detectability and sensitivity. The feasibility and effectiveness of the proposed method are validated using a numerical example and a real-world application, and good detection performance can be obtained in terms of Receiver Operating Characteristic (ROC) curves.
更多查看译文
关键词
Sensor fault detection,Transmissibility,FIR model,Neyman–Pearson test
AI 理解论文
溯源树
样例
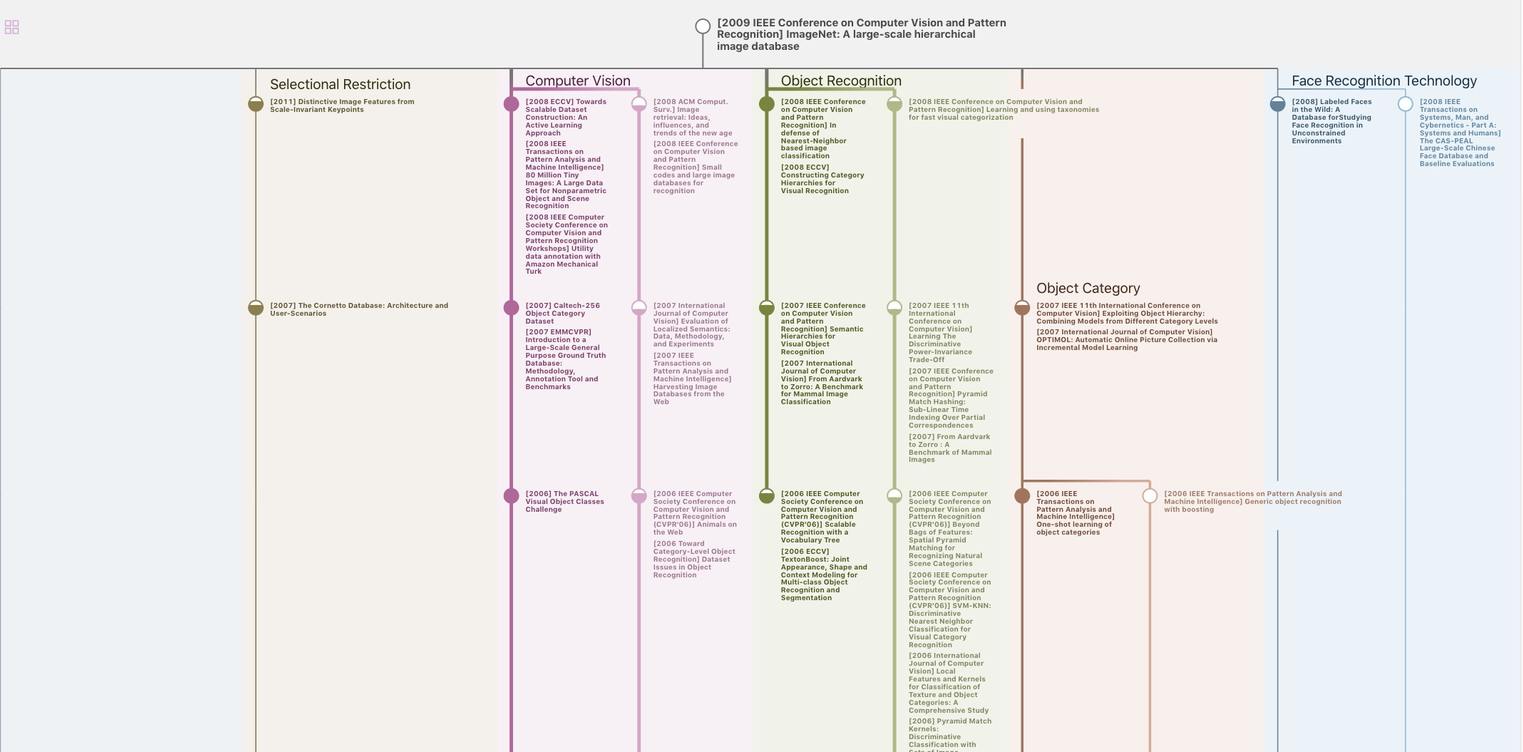
生成溯源树,研究论文发展脉络
Chat Paper
正在生成论文摘要