Deep probabilistic model for lossless scalable point cloud attribute compression
arxiv(2023)
摘要
In recent years, several point cloud geometry compression methods that utilize advanced deep learning techniques have been proposed, but there are limited works on attribute compression, especially lossless compression. In this work, we build an end-to-end multiscale point cloud attribute coding method (MNeT) that progressively projects the attributes onto multiscale latent spaces. The multiscale architecture provides an accurate context for the attribute probability modeling and thus minimizes the coding bitrate with a single network prediction. Besides, our method allows scalable coding that lower quality versions can be easily extracted from the losslessly compressed bitstream. We validate our method on a set of point clouds from MVUB and MPEG and show that our method outperforms recently proposed methods and on par with the latest G-PCC version 14. Besides, our coding time is substantially faster than G-PCC.
更多查看译文
关键词
Point Cloud,Sparse Convolution,Deep Learning,G-PCC,MNeT
AI 理解论文
溯源树
样例
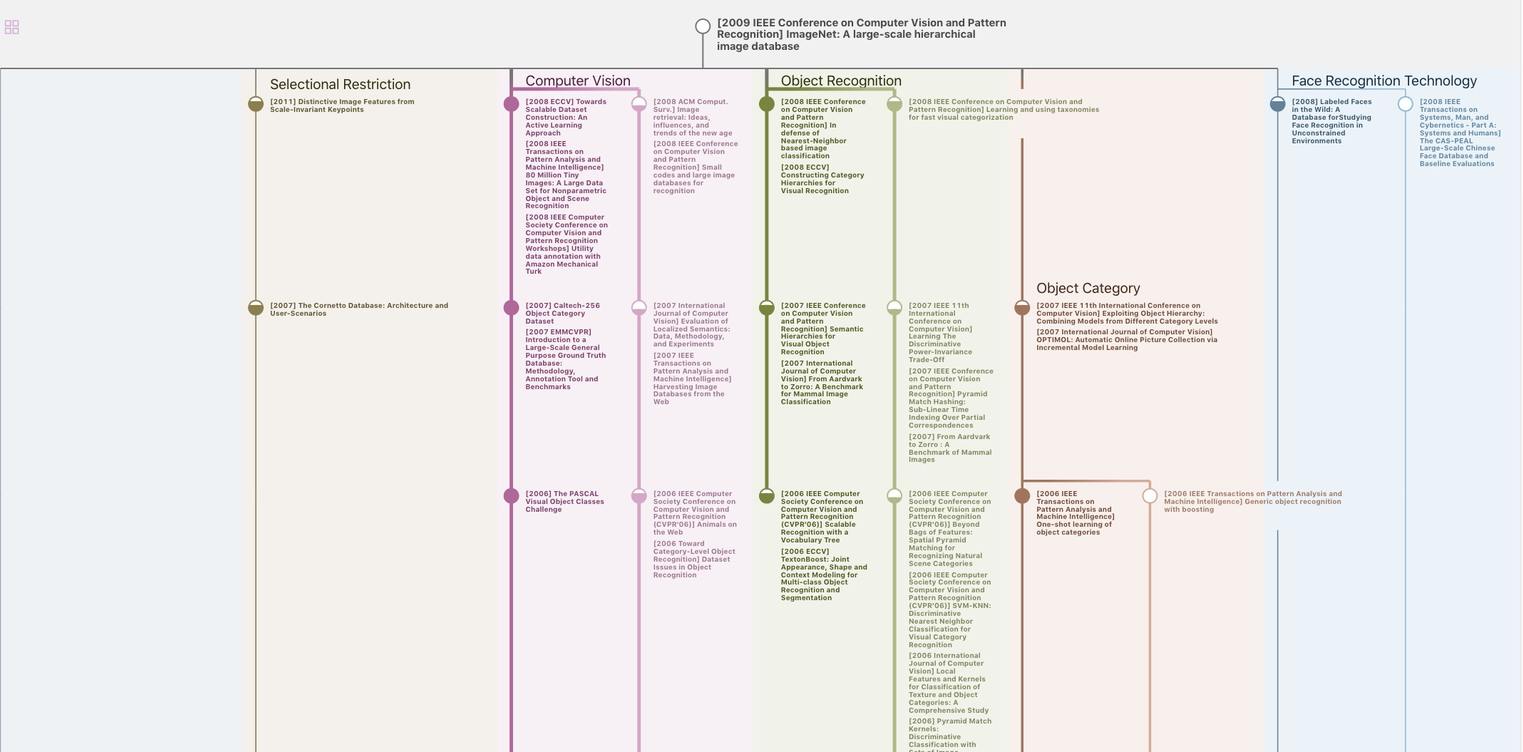
生成溯源树,研究论文发展脉络
Chat Paper
正在生成论文摘要