MetaUE: Model-based Meta-learning for Underwater Image Enhancement
CoRR(2023)
摘要
The challenges in recovering underwater images are the presence of diverse degradation factors and the lack of ground truth images. Although synthetic underwater image pairs can be used to overcome the problem of inadequately observing data, it may result in over-fitting and enhancement degradation. This paper proposes a model-based deep learning method for restoring clean images under various underwater scenarios, which exhibits good interpretability and generalization ability. More specifically, we build up a multi-variable convolutional neural network model to estimate the clean image, background light and transmission map, respectively. An efficient loss function is also designed to closely integrate the variables based on the underwater image model. The meta-learning strategy is used to obtain a pre-trained model on the synthetic underwater dataset, which contains different types of degradation to cover the various underwater environments. The pre-trained model is then fine-tuned on real underwater datasets to obtain a reliable underwater image enhancement model, called MetaUE. Numerical experiments demonstrate that the pre-trained model has good generalization ability, allowing it to remove the color degradation for various underwater attenuation images such as blue, green and yellow, etc. The fine-tuning makes the model able to adapt to different underwater datasets, the enhancement results of which outperform the state-of-the-art underwater image restoration methods. All our codes and data are available at \url{https://github.com/Duanlab123/MetaUE}.
更多查看译文
关键词
underwater,enhancement,model-based,meta-learning
AI 理解论文
溯源树
样例
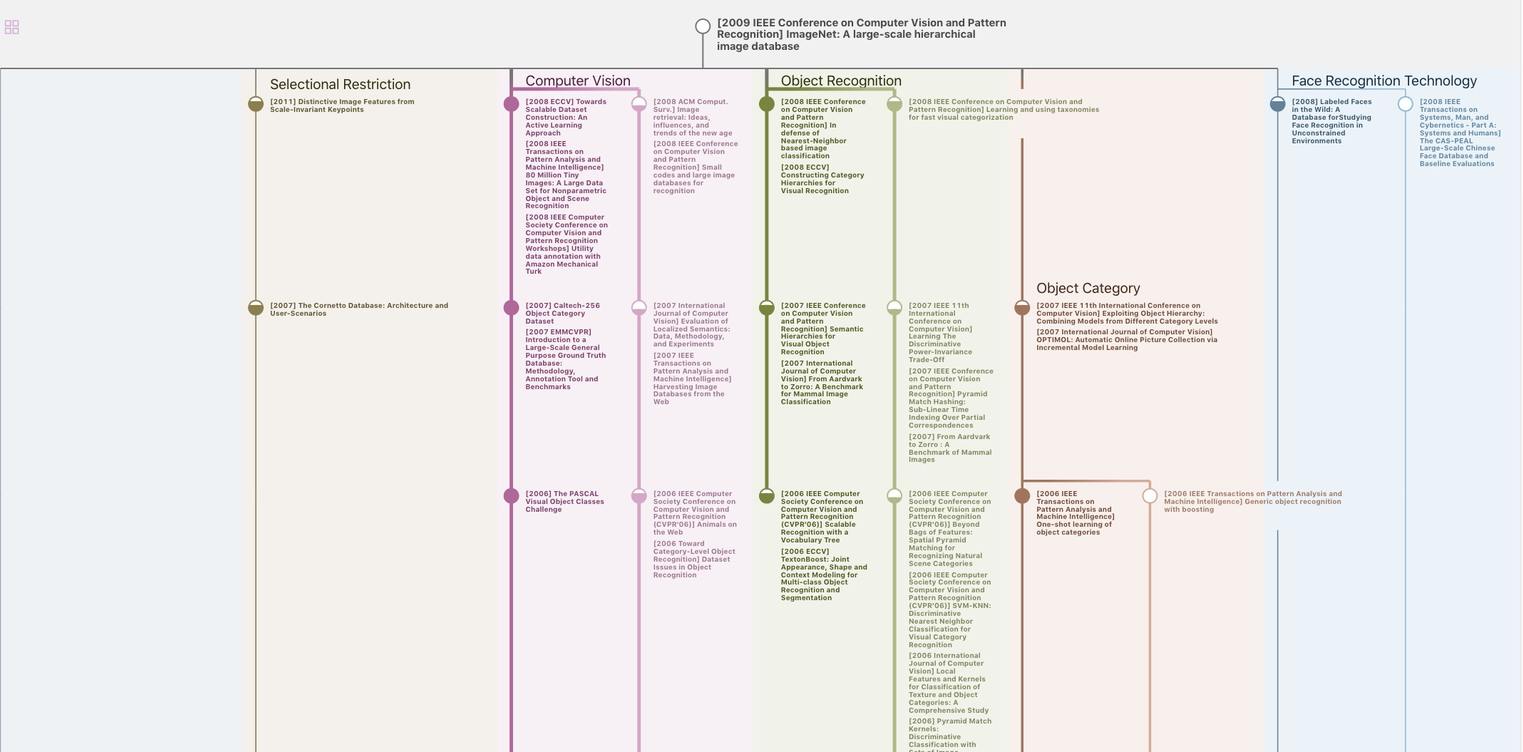
生成溯源树,研究论文发展脉络
Chat Paper
正在生成论文摘要