PiSL: Physics-informed Spline Learning for data-driven identification of nonlinear dynamical systems
Mechanical Systems and Signal Processing(2023)
摘要
Nonlinear dynamics is ubiquitous in nature and commonly seen in many science and engineering disciplines. Distilling analytical expressions that govern the behavior of nonlinear dynamical systems from limited data is vital but remains challenging. To tackle this fundamental issue, we propose a novel Physics-informed Spline Learning (PiSL) framework to discover parsimonious governing equations for nonlinear dynamics, based on sparsely sampled noisy data. Specifically, splines are employed to locally interpolate the dynamics and perform analytical differentiation, feeding the discovery of underlying equations in form of either a linear interpolation of candidate terms or a symbolic-activated neural network model. The physics residual in turn informs the spline learning. The synergy between splines and discovered governing equations produces great robustness against high-level data sparsity and noise. Subsequently, a hybrid sparsity-promoting alternating direction optimization strategy is developed for fine-tuning the coefficients with a sparsity enforcement approach to obtain a parsimonious structure of discovered governing equations. The effectiveness and supremacy of the proposed PiSL architectures have been demonstrated by several numerical and experimental examples, in comparison with two state-of-the-art methods serving as baselines.
更多查看译文
关键词
Spline learning,System discovery,Sparse identification,Neural networks,Dynamical systems
AI 理解论文
溯源树
样例
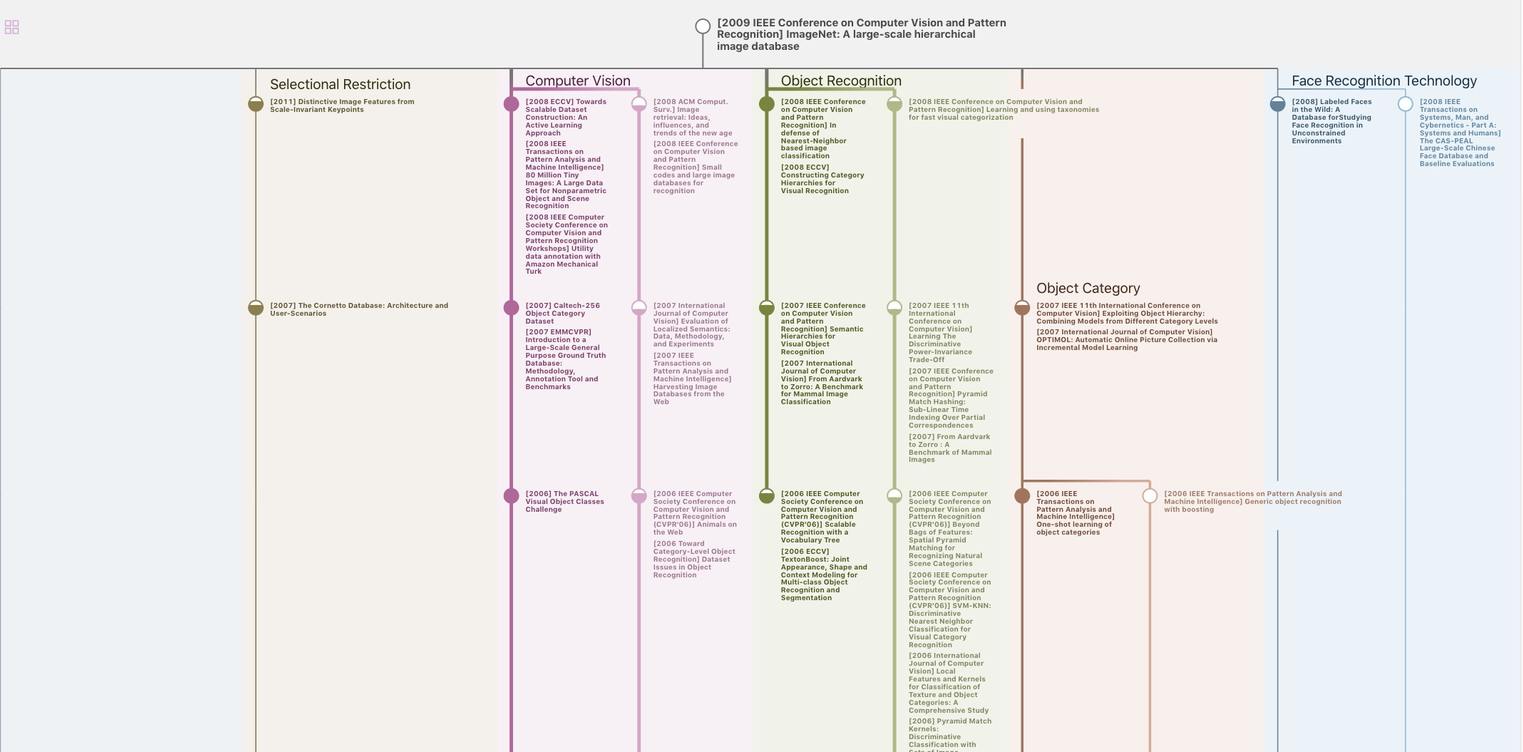
生成溯源树,研究论文发展脉络
Chat Paper
正在生成论文摘要