Fungal identification in peanuts seeds through multispectral images: Technological advances to enhance sanitary quality.
Frontiers in plant science(2023)
摘要
The sanitary quality of seed is essential in agriculture. This is because pathogenic fungi compromise seed physiological quality and prevent the formation of plants in the field, which causes losses to farmers. Multispectral images technologies coupled with machine learning algorithms can optimize the identification of healthy peanut seeds, greatly improving the sanitary quality. The objective was to verify whether multispectral images technologies and artificial intelligence tools are effective for discriminating pathogenic fungi in tropical peanut seeds. For this purpose, dry peanut seeds infected by fungi (, , sp., and sp.) were used to acquire images at different wavelengths (365 to 970 nm). Multispectral markers of peanut seed health quality were found. The incubation period of 216 h was the one that most contributed to discriminating healthy seeds from those containing fungi through multispectral images. Texture (Percent Run), color (CIELab *) and reflectance (490 nm) were highly effective in discriminating the sanitary quality of peanut seeds. Machine learning algorithms (LDA, MLP, RF, and SVM) demonstrated high accuracy in autonomous detection of seed health status (90 to 100%). Thus, multispectral images coupled with machine learning algorithms are effective for screening peanut seeds with superior sanitary quality.
更多查看译文
关键词
Arachis hypogaea L.,Aspergillus spp.,machine learning,seed health,support vector machine
AI 理解论文
溯源树
样例
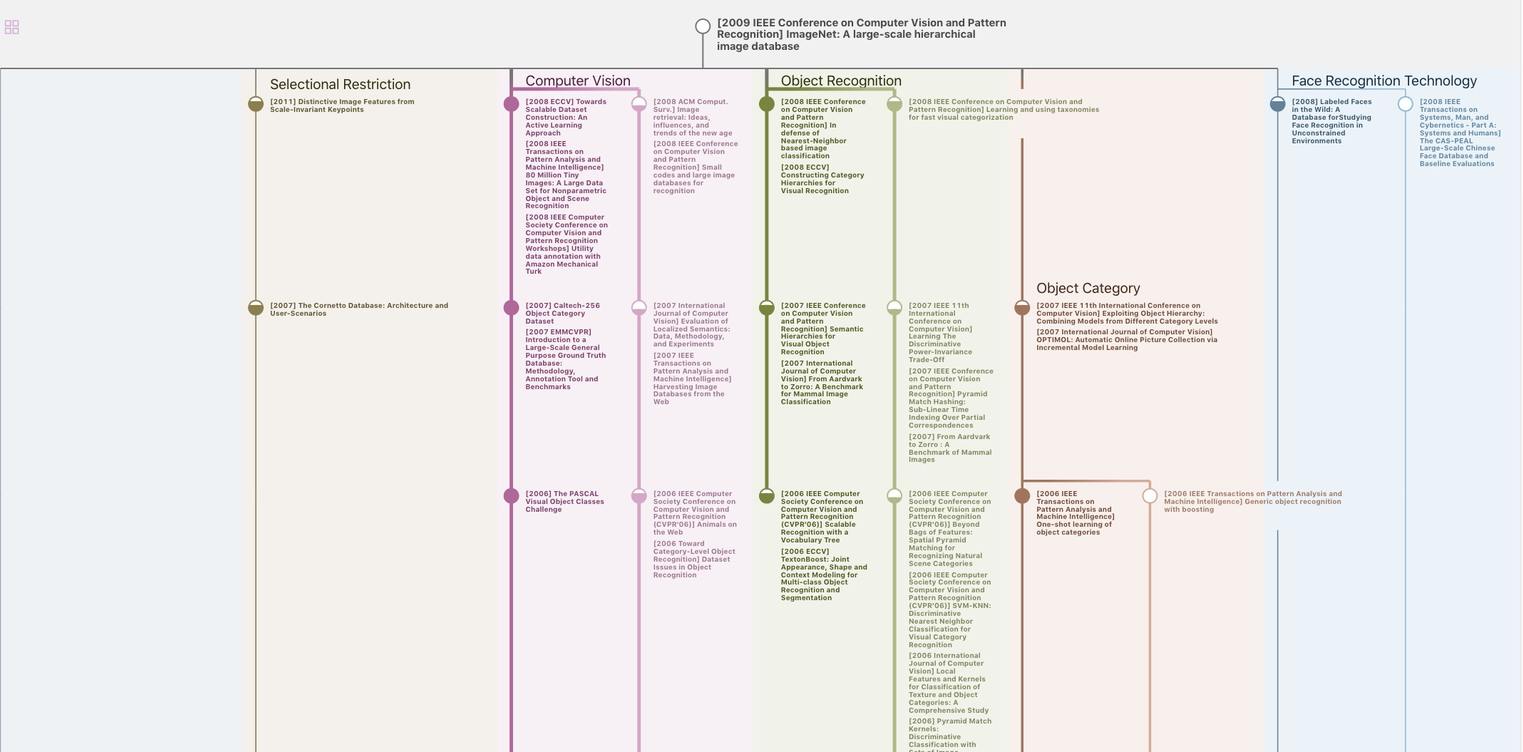
生成溯源树,研究论文发展脉络
Chat Paper
正在生成论文摘要