A systematic review of Generative Adversarial Networks (GANs) in Plastic Surgery
Journal of Plastic, Reconstructive & Aesthetic Surgery(2024)
摘要
Introduction
Generative Adversarial Networks (GANs) are a form of deep learning architecture based on zero-sum game theory, which uses real data to generate realistic fake data. GANs use two opposing neural networks working, a generator and discriminator. They represent a powerful tool for generation of realistic synthetic patient data sets and have the potential to revolutionize research. This systematic literature review evaluates the scale and scope of GANs within Plastic Surgery, constructing a framework for its use and evaluation within subspecialties.
Methods
Following PRISMA guidelines, a systematic review was performed for applications of GANs in Plastic Surgery from 2014 to 2022. Three independent reviewers screened from databases including PubMed, Embase, PsychInfo, Scopus, and Google Scholar.
Results
A total of 70 studies were captured by the search, of which seven studies met our criteria. The most common subspecialty was craniofacial (n=4). Proposed uses of GANs ranged from facial recognition, burn estimation, scar prediction, and post-breast cancer reconstruction anomaly scoring. The GANs were conditional, trained on datasets averaging 54,652±112,180 samples, with some sourced publicly and others being primary.
Conclusion
GANs hold promise for advancing plastic surgery, backed by diverse applications in literature. Studies should follow a standardised reporting structure for consistency and transparency, as outlined, especially regarding the datasets used to ensure appropriate representation from an ethnic and cultural diversity perspective. Whilst GANs require specialist computational expertise to create, it is important for surgeons to understand their development leveraging GANs’ full potential within the emerging field of computational Plastic Surgery and beyond.
更多查看译文
关键词
Generative Adversarial Networks,Plastic Surgery,Medical Imaging
AI 理解论文
溯源树
样例
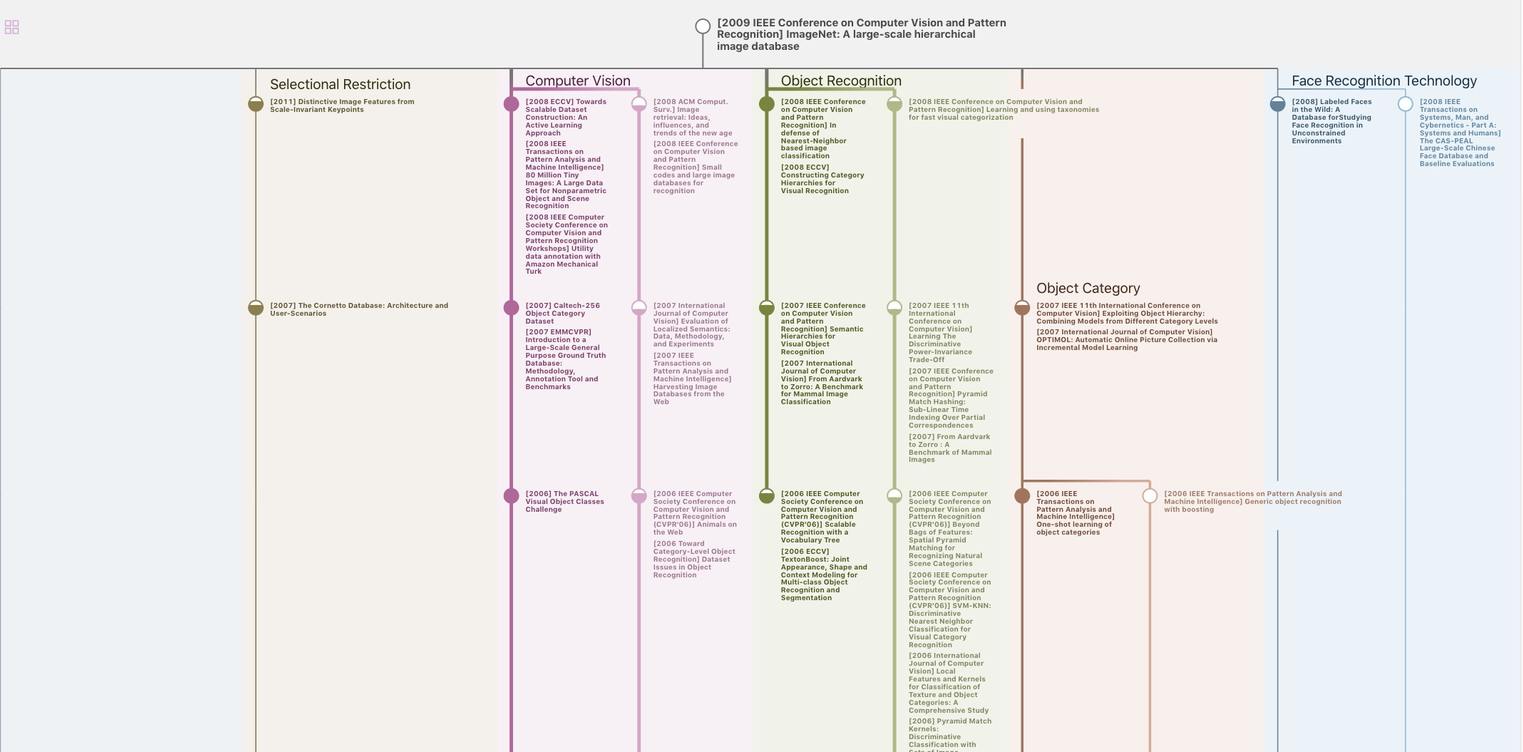
生成溯源树,研究论文发展脉络
Chat Paper
正在生成论文摘要