First passage time analysis of spatial mutation patterns reveals sub-clonal evolutionary dynamics in colorectal cancer
PLoS computational biology(2023)
摘要
Author summaryTumours consist of a mosaic of cell sub-populations, which may differ in ways such as growth rate and their response to anti-cancer treatment. Understanding how sub-populations emerge and evolve is therefore central to predicting future behaviour of tumours and improving treatment regimes. Traditional methods of studying tumour heterogeneity, however, are often ignorant to the spatial context of cells, or preserve only low-resolution spatial data, yet spatial information may contain important clues about the evolution of tumour sub-populations. To exploit this fact, new methods of describing spatial patterns of tumour cells are needed in order to relate experimental observations to the underlying evolution of the tumour. In this study, we present a new methodology for spatial analysis of tumour tissue, utilising random walks to quantify clustering and heterogeneity within high-resolution spatial maps of tumour sub-population mixing. We apply our method to maps of sub-population mixing in colorectal cancer samples and, using spatial computational modelling, estimate the relative growth rate and age of mutated sub-populations, along with the strength of cell pushing within these samples. Our work demonstrates the potential of spatial information to inform about cancer evolution, and establishes a foundation for future research into spatial analysis of tumour data. The signature of early cancer dynamics on the spatial arrangement of tumour cells is poorly understood, and yet could encode information about how sub-clones grew within the expanding tumour. Novel methods of quantifying spatial tumour data at the cellular scale are required to link evolutionary dynamics to the resulting spatial architecture of the tumour. Here, we propose a framework using first passage times of random walks to quantify the complex spatial patterns of tumour cell population mixing. First, using a simple model of cell mixing we demonstrate how first passage time statistics can distinguish between different pattern structures. We then apply our method to simulated patterns of mutated and non-mutated tumour cell population mixing, generated using an agent-based model of expanding tumours, to explore how first passage times reflect mutant cell replicative advantage, time of emergence and strength of cell pushing. Finally, we explore applications to experimentally measured human colorectal cancer, and estimate parameters of early sub-clonal dynamics using our spatial computational model. We infer a wide range of sub-clonal dynamics, with mutant cell division rates varying between 1 and 4 times the rate of non-mutated cells across our sample set. Some mutated sub-clones emerged after as few as 100 non-mutant cell divisions, and others only after 50,000 divisions. The majority were consistent with boundary driven growth or short-range cell pushing. By analysing multiple sub-sampled regions in a small number of samples, we explore how the distribution of inferred dynamics could inform about the initial mutational event. Our results demonstrate the efficacy of first passage time analysis as a new methodology in spatial analysis of solid tumour tissue, and suggest that patterns of sub-clonal mixing can provide insights into early cancer dynamics.
更多查看译文
关键词
spatial mutation patterns,colorectal cancer,sub-clonal
AI 理解论文
溯源树
样例
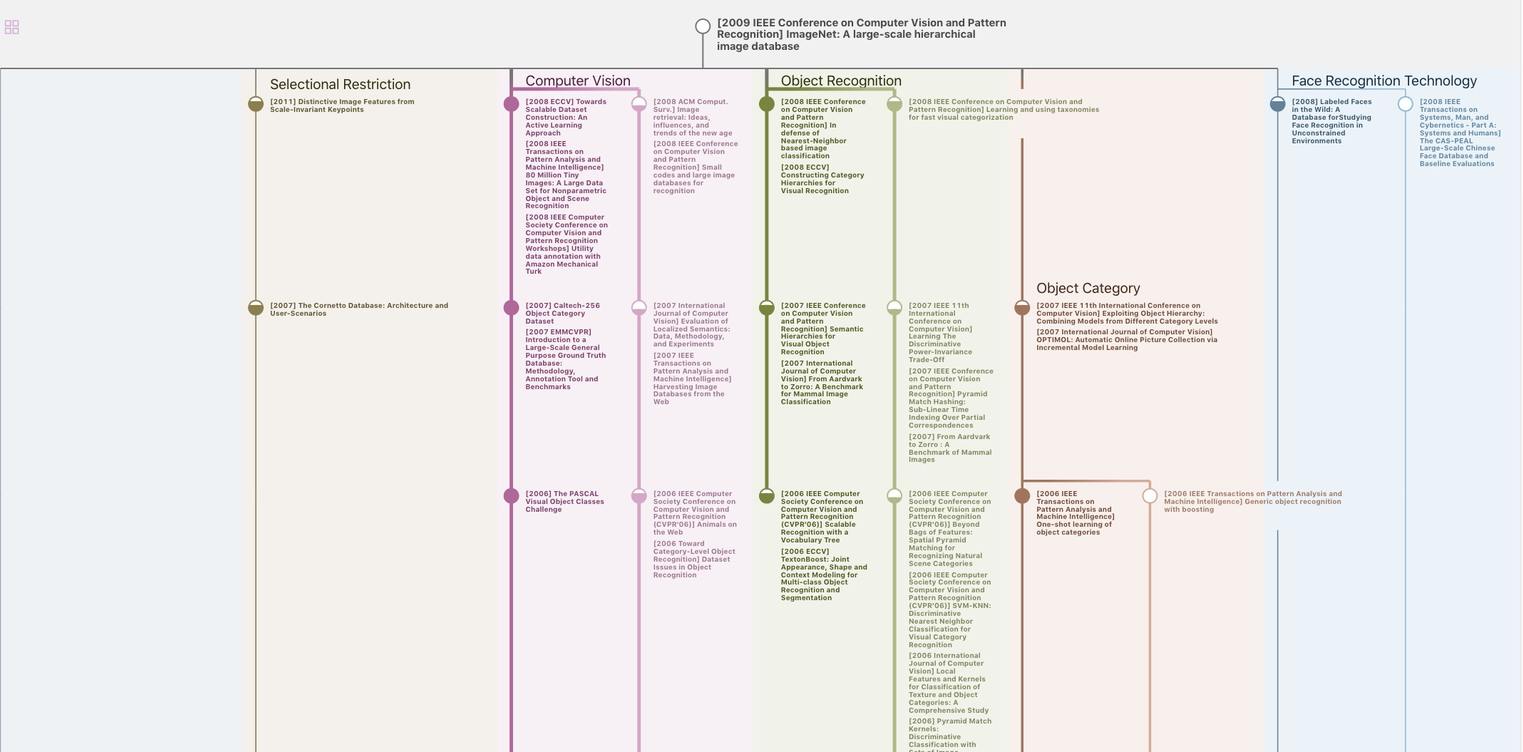
生成溯源树,研究论文发展脉络
Chat Paper
正在生成论文摘要