Loss of Plasticity in Continual Deep Reinforcement Learning
arxiv(2023)
摘要
The ability to learn continually is essential in a complex and changing world. In this paper, we characterize the behavior of canonical value-based deep reinforcement learning (RL) approaches under varying degrees of non-stationarity. In particular, we demonstrate that deep RL agents lose their ability to learn good policies when they cycle through a sequence of Atari 2600 games. This phenomenon is alluded to in prior work under various guises -- e.g., loss of plasticity, implicit under-parameterization, primacy bias, and capacity loss. We investigate this phenomenon closely at scale and analyze how the weights, gradients, and activations change over time in several experiments with varying dimensions (e.g., similarity between games, number of games, number of frames per game), with some experiments spanning 50 days and 2 billion environment interactions. Our analysis shows that the activation footprint of the network becomes sparser, contributing to the diminishing gradients. We investigate a remarkably simple mitigation strategy -- Concatenated ReLUs (CReLUs) activation function -- and demonstrate its effectiveness in facilitating continual learning in a changing environment.
更多查看译文
关键词
deep reinforcement learning,reinforcement learning,plasticity
AI 理解论文
溯源树
样例
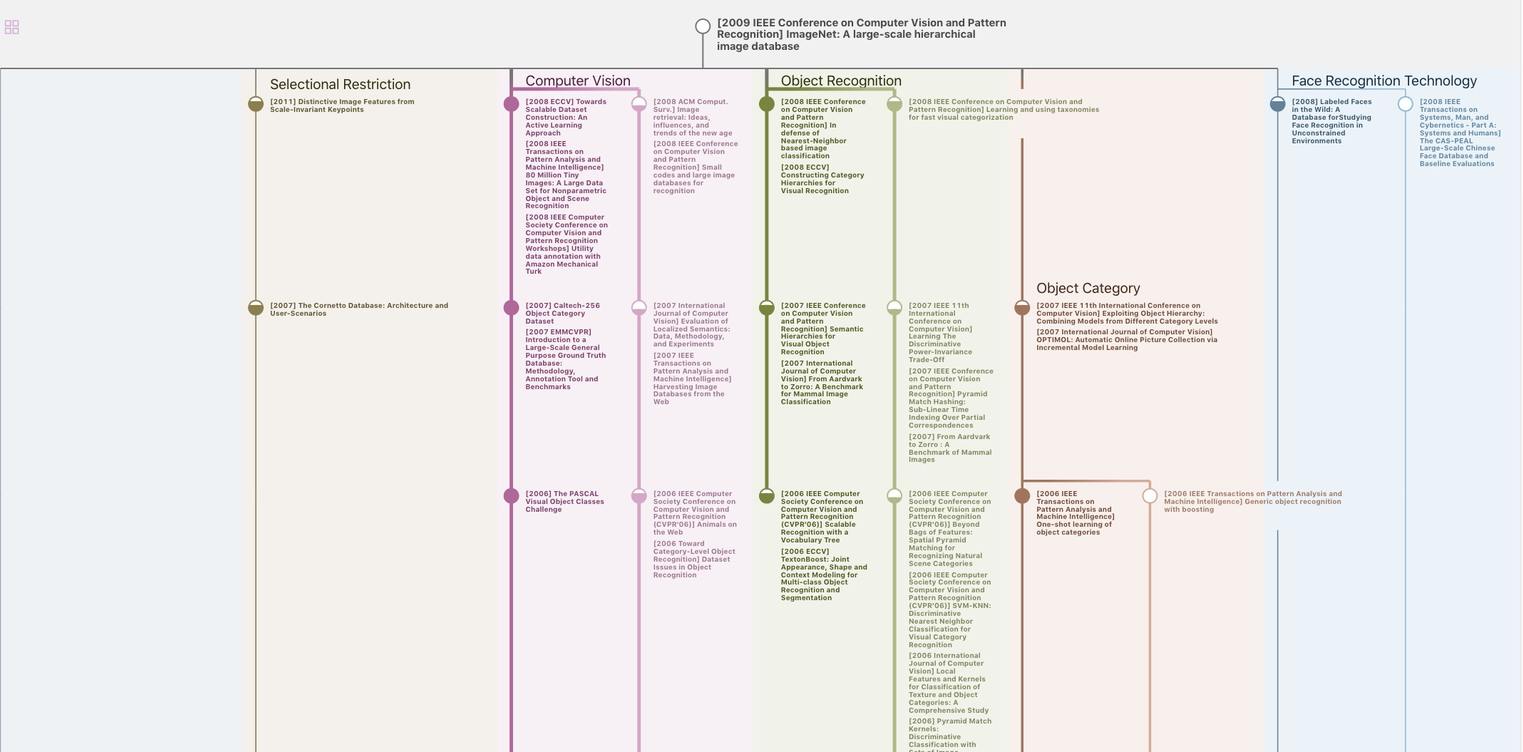
生成溯源树,研究论文发展脉络
Chat Paper
正在生成论文摘要