Constrained Adversarial Learning and its applicability to Automated Software Testing: a systematic review
arxiv(2023)
摘要
Every novel technology adds hidden vulnerabilities ready to be exploited by a growing number of cyber-attacks. Automated software testing can be a promising solution to quickly analyze thousands of lines of code by generating and slightly modifying function-specific testing data to encounter a multitude of vulnerabilities and attack vectors. This process draws similarities to the constrained adversarial examples generated by adversarial learning methods, so there could be significant benefits to the integration of these methods in automated testing tools. Therefore, this systematic review is focused on the current state-of-the-art of constrained data generation methods applied for adversarial learning and software testing, aiming to guide researchers and developers to enhance testing tools with adversarial learning methods and improve the resilience and robustness of their digital systems. The found constrained data generation applications for adversarial machine learning were systematized, and the advantages and limitations of approaches specific for software testing were thoroughly analyzed, identifying research gaps and opportunities to improve testing tools with adversarial attack methods.
更多查看译文
关键词
software testing,automated
AI 理解论文
溯源树
样例
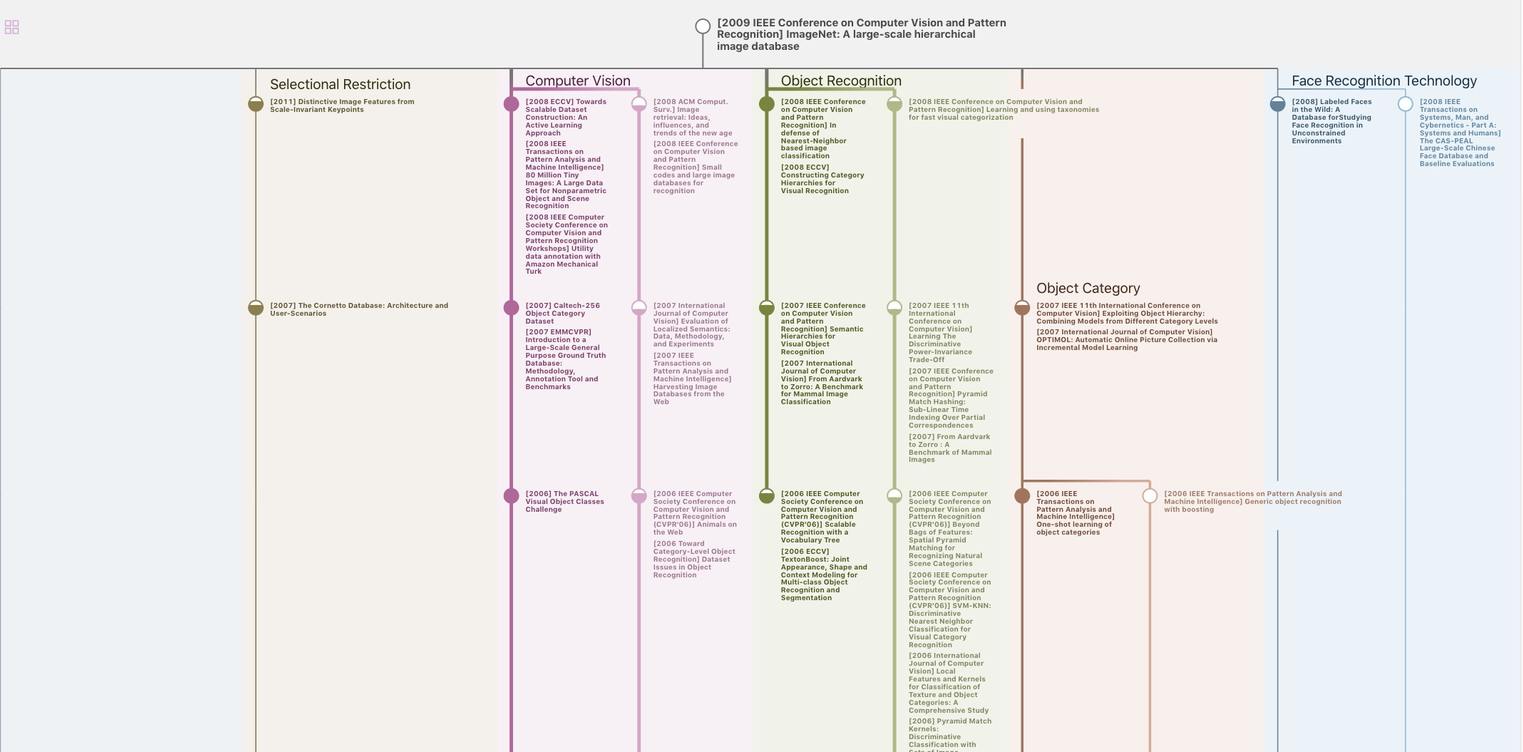
生成溯源树,研究论文发展脉络
Chat Paper
正在生成论文摘要