A Sparse Autoencoder Based Adversarial Open Set Domain Adaptation Model for Fault Diagnosis of Rotating Machinery
2022 International Conference on Sensing, Measurement & Data Analytics in the era of Artificial Intelligence (ICSMD)(2022)
摘要
Rotating machinery is an integral part of many industrial systems. Domain adaptation technique provides a powerful tool to detect faults under different working conditions. However, there is still a challenge: conventional domain adaptation approach only works under the ‘closed set’ assumption that all test classes are known at training time. In practice, a more realistic situation is ‘open set’, i.e., knowledge is incomplete in the training process, resulting in unknown classes during the testing. In this paper, a sparse autoencoder based adversarial open set domain adaptation (SAOSDA) model is proposed for rotating machinery fault diagnosis under open set scenarios, which can recognize the unknown faults and detect the known faults under different working conditions. This model utilizes adversarial learning to reduce the discrepancies between source samples and known target samples and reject the unknown target samples simultaneously. Experimental results of the actual bearing dataset verify the superiority and effectiveness of this method.
更多查看译文
关键词
adversarial learning,deep learning,domain adaptation,fault diagnosis,open set recognition,rotating machinery,unknown faults,sparse autoencoder
AI 理解论文
溯源树
样例
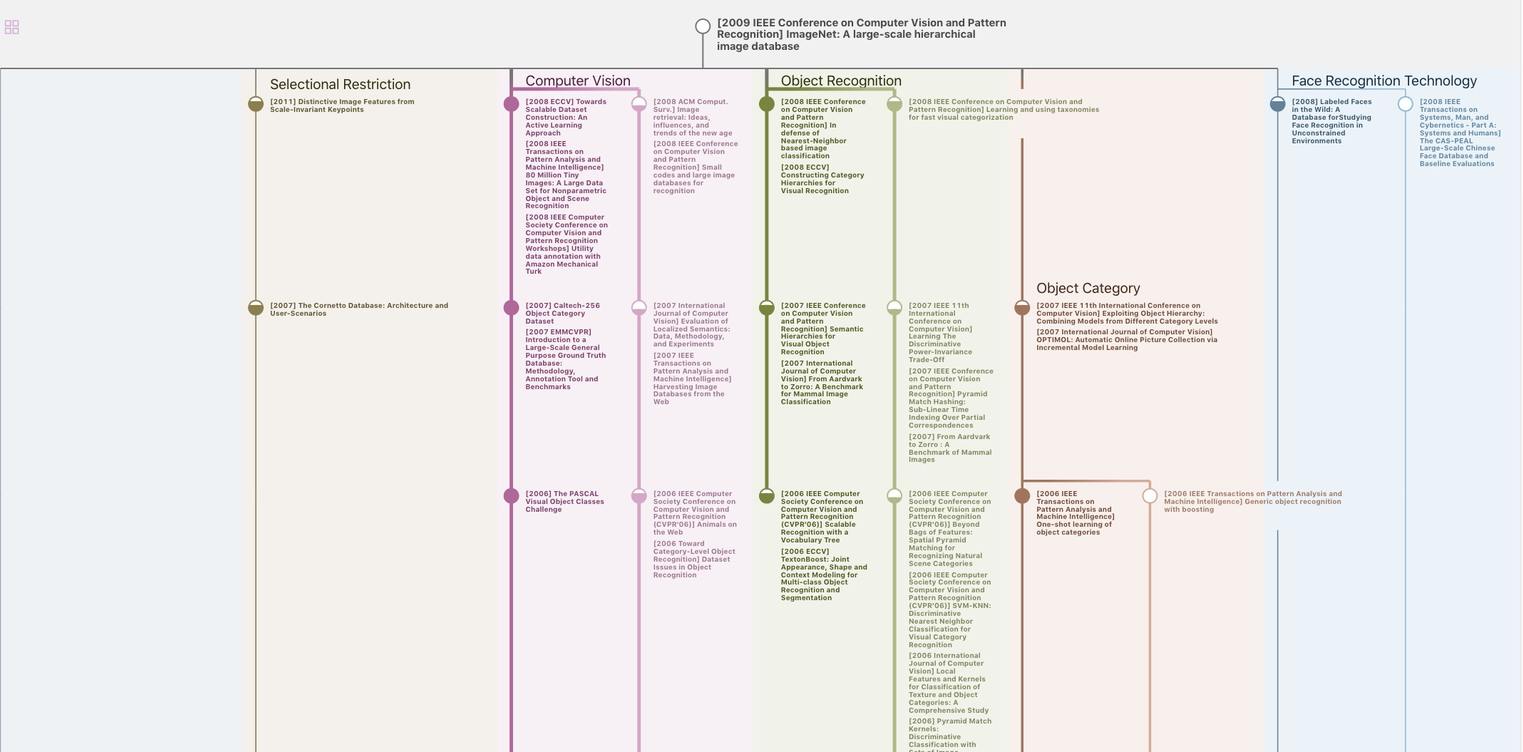
生成溯源树,研究论文发展脉络
Chat Paper
正在生成论文摘要