Performance Evaluation of Text Augmentation Methods with BERT on Small-sized, Imbalanced Datasets
2022 IEEE 4th International Conference on Cognitive Machine Intelligence (CogMI)(2022)
摘要
Recently deep learning methods have achieved great success in understanding and analyzing text messages. In real-world applications, however, labeled text data are often small-sized and imbalanced in classes due to the high cost of data collection and human annotation, limiting the performance of deep learning classifiers. Therefore, this study explores an understudied area—how sample sizes and imbalance ratios influence the performance of deep learning models and augmentation methods—and provides a solution to this problem. Specifically, this study examines the performance of BERT, Word2Vec, and WordNet augmentation methods with BERT fine-tuning on datasets of sizes 500, 1,000, and 2,000 and imbalance ratios of 4:1 and 9:1. Experimental results show that BERT augmentation improves the performance of BERT in detecting the minority class, and the improvement is most significantly (15.6–40.4% F1 increase compared to the base model and 2.8%–10.4% F1 increase compared to the model with the oversampling method) when the data size is small (e.g., 500 training documents) and highly imbalanced (e.g., 9:1). When the data size increases or the imbalance ratio decreases, the improvement generated by the BERT augmentation becomes smaller or insignificant. Moreover, BERT augmentation plus BERT fine-tuning achieves the best performance compared to other models and methods, demonstrating a promising solution for small-sized, highly imbalanced text classification tasks.
更多查看译文
关键词
text classification,NLP,imbalanced dataset,data augmentation,machine learning,deep learning
AI 理解论文
溯源树
样例
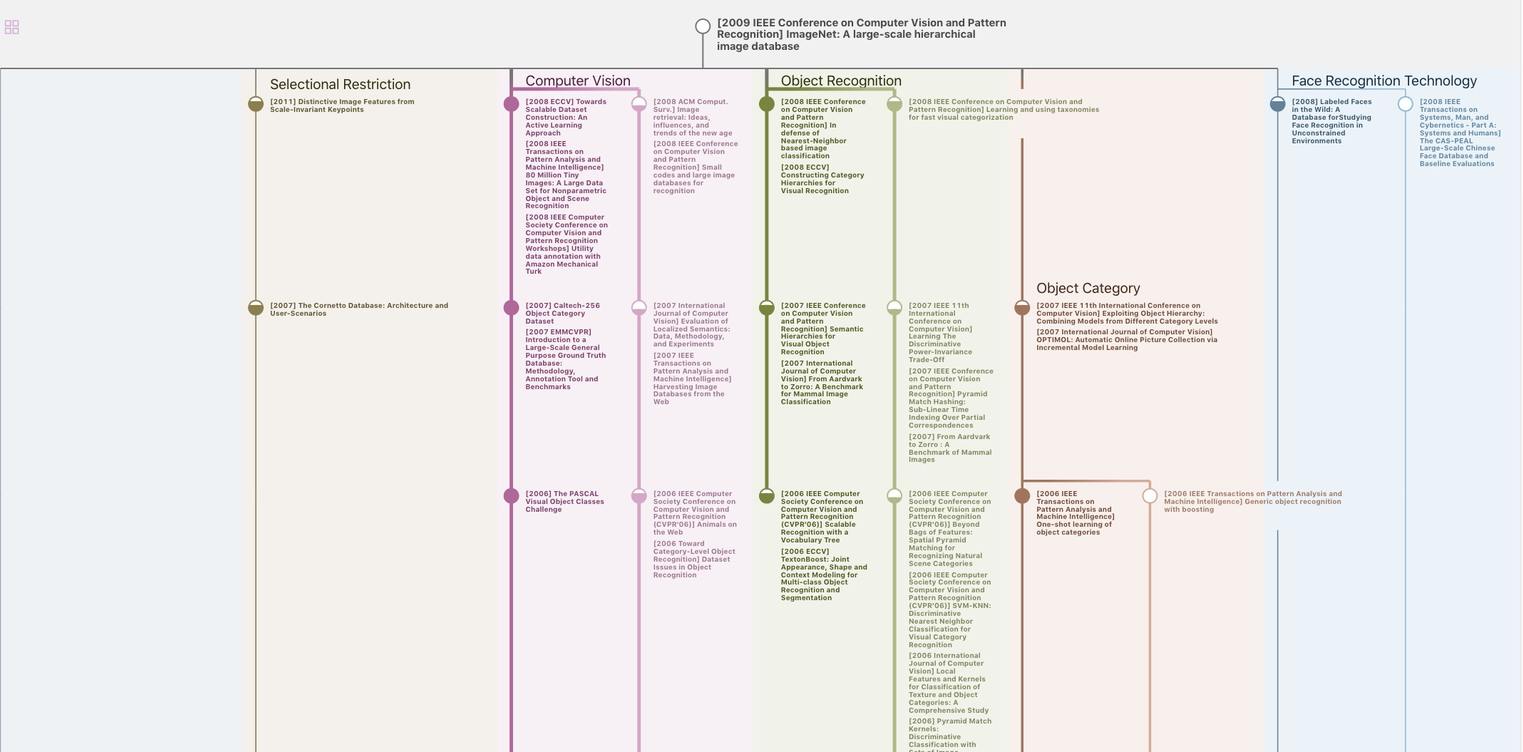
生成溯源树,研究论文发展脉络
Chat Paper
正在生成论文摘要