Enhanced Deep Learning Super-Resolution for Bathymetry Data
2022 IEEE/ACM International Conference on Big Data Computing, Applications and Technologies (BDCAT)(2022)
摘要
Spatial resolution is critical for observing and monitoring environmental phenomena. Acquiring high-resolution bathymetry data directly from satellites is not always feasible due to limitations on equipment, so spatial data scientists and researchers turn to single image super-resolution (SISR) methods that utilize deep learning techniques as an alternative method to increase pixel density. While super resolution residual networks (e.g., SR-ResNet) are promising for this purpose, several challenges still need to be addressed: (1) Earth data such as bathymetry is expensive to obtain and relatively limited in its data record amount; (2) certain domain knowledge needs to be complied with during model training; (3) certain areas of interest require more accurate measurements than other areas. To address these challenges, following the transfer learning principle, we study how to leverage an existing pre-trained super-resolution deep learning model, namely SR-ResNet, for high-resolution bathymetry data generation. We further enhance the SR-ResNet model to add corresponding loss functions based on domain knowledge. To let the model perform better for certain spatial areas, we add additional loss functions to increase the penalty of the areas of interest. Our experiments show our approaches achieve higher accuracy than most baseline models when evaluating using metrics including MSE, PSNR, and SSIM.
更多查看译文
关键词
deep learning,super-resolution,bathymetry data,transfer learning
AI 理解论文
溯源树
样例
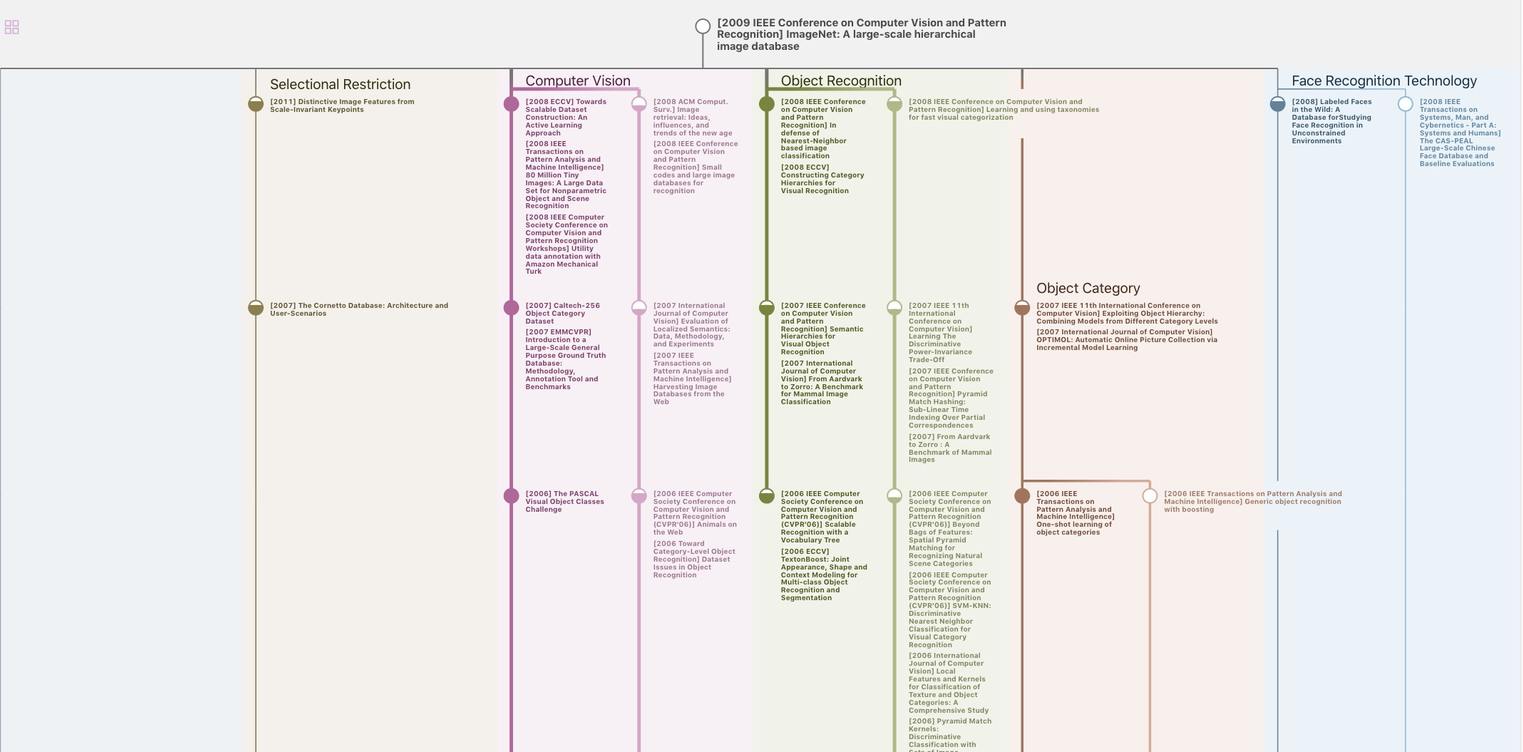
生成溯源树,研究论文发展脉络
Chat Paper
正在生成论文摘要