Discriminative Feature Extraction Network for Generalized Zero Shot Learning
2022 China Automation Congress (CAC)(2022)
摘要
Generalized zero shot learning (GZSL), which aims to recognize both the seen and unseen classes conditioned on shared semantic information, has achieved remarkable progress. Generative based methods have proved an effective way in addressing the data-imbalanced problem in GZSL via generating the unseen visual samples. Nevertheless, non- discriminative information caused by cross dataset bias issue still severely deteriorates the model performance. To address this problem, a novel method named discriminative feature extraction network (DFEN) for GZSL was proposed. DFEN incorporated a discriminative feature extraction (DFE) module in a unified generative model to extract the discriminative information of visual samples by visual to semantic mapping. Meanwhile, semantic consistency constraint was used to guide the DFE module to learn the semantically relevant representation. To further boost the discriminative feature, adversarial learning manner was adopted to encourage the mapped semantic representations are consistent with the actual semantic embeddings. Numerous experiments have implemented on four benchmark datasets, which demonstrates that proposed method performs better than the recent advanced methods significantly.
更多查看译文
关键词
generalized zero shot learning,generative based methods,semantic consistency constraint
AI 理解论文
溯源树
样例
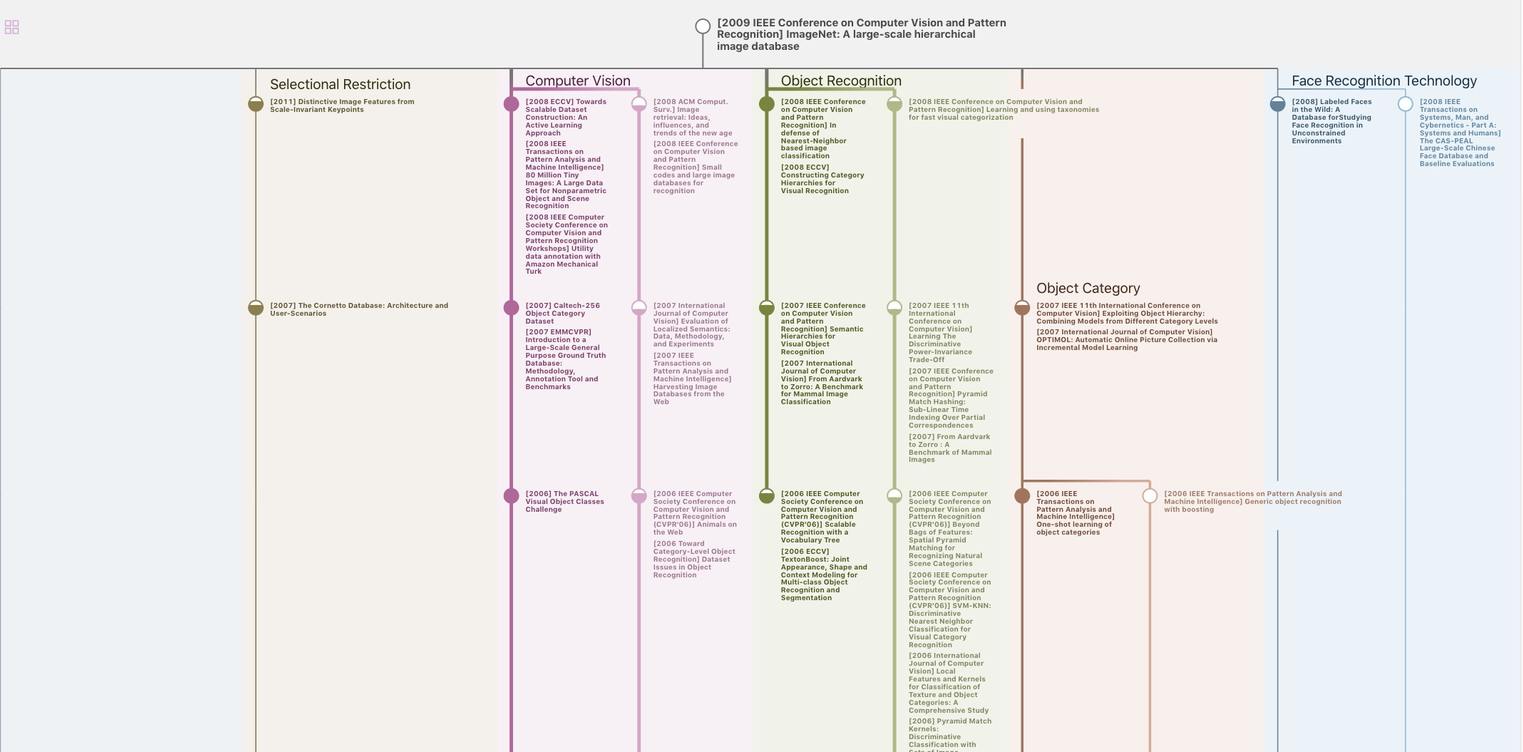
生成溯源树,研究论文发展脉络
Chat Paper
正在生成论文摘要