Promoting Monocular Depth Estimation by Multi-Scale Residual Laplacian Pyramid Fusion
IEEE Signal Processing Letters(2023)
摘要
Deep learning approach has achieved great success in monocular depth estimation. However, the learned deep network may produce a depth map with fewer details and incorrect global depth layout, especially when the learned network is applied to a high-resolution image. In order to generate a high-quality depth map with better global structure and richer details, we propose a multi-scale residual Laplacian pyramid fusion net (MS-RLap-FNet), to fuse the multi-scale depth maps estimated by the existing depth estimation models, for depth refinement. Our approach relies on a proposed multi-scale residual Laplacian pyramid decomposition of the multi-scale depth maps, and the fusion network modules to gradually refine the depth maps based on the decomposition from low to high resolution. Comprehensive experiments show that our method, by refining the depth maps based on three popular monocular depth estimation models (DPT, MiDas, SGR), outperforms the existing state-of-the-art methods both in quantity and quality on three public datasets with different image resolutions. The depth map refined by our method has better global depth layout with richer fine details.
更多查看译文
关键词
Depth refinement,Laplacian pyramid fusion,multi-scale residual
AI 理解论文
溯源树
样例
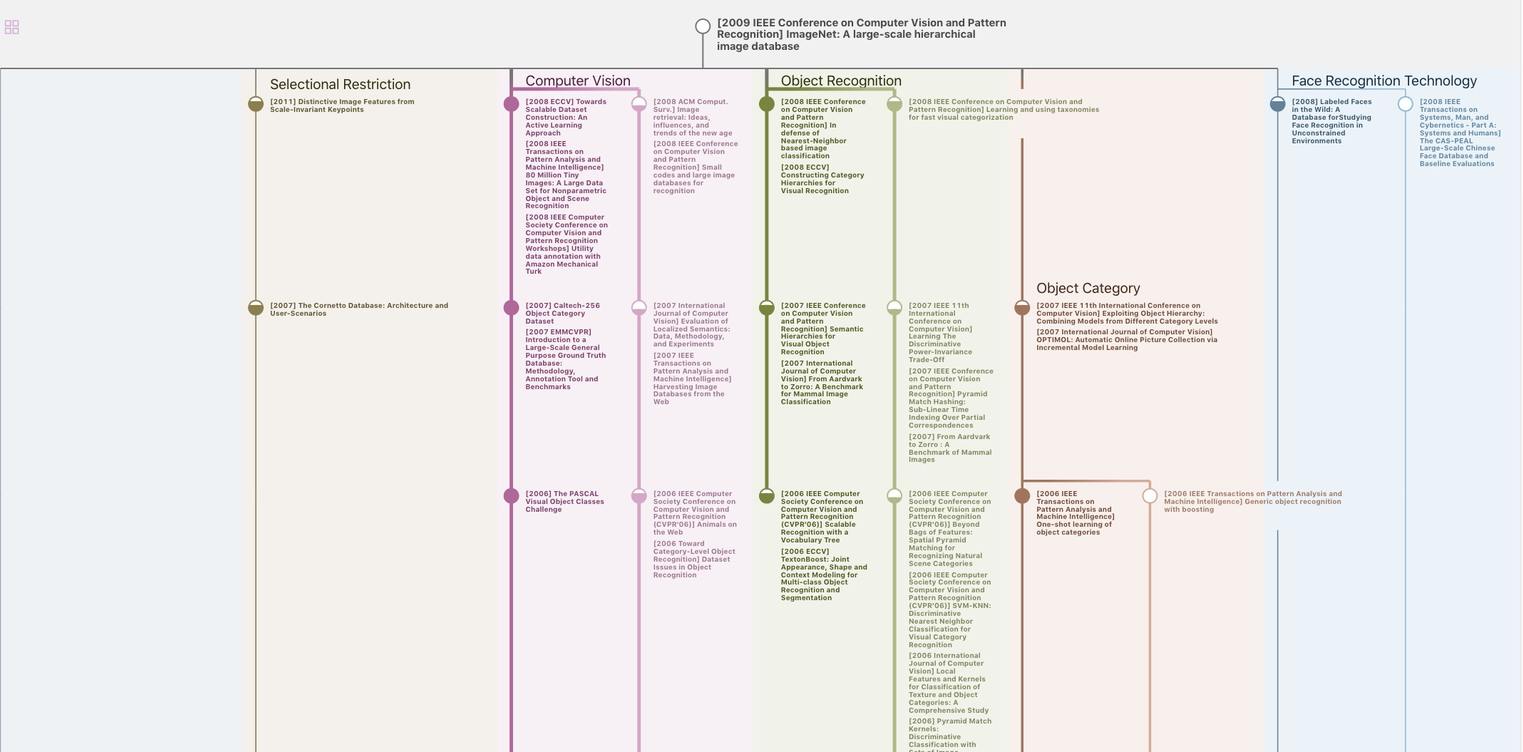
生成溯源树,研究论文发展脉络
Chat Paper
正在生成论文摘要