Daily Posture Behavior Patterns Derived From Multitime-Scale Topic Models Using Wearable Triaxial Acceleration for Assessment of Concern About Falling
IEEE Sensors Journal(2023)
摘要
Concern about falling is prevalent in older population. This condition would cause a series of adverse physical and psychological consequences for older adults’ health. Traditional assessment of concern about falling is relied on self-reported questionnaires and thus is too subjective. Therefore, we proposed a novel multitime-scale topic modeling approach to quantitatively evaluate concerns about falling by analyzing triaxial acceleration signals collected from a wearable pendent sensor. Different posture segments were first recognized to extract their corresponding feature subsets. Then, each selected feature related to concern about falling was clustered into discrete levels as feature letters of artificial words in different time scales. As a result, all older participants’ signal recordings were converted to a collection of artificial documents, which can be processed by natural language processing methodologies. The topic modeling technique was used to discover daily posture behavior patterns from these documents as discriminants between older adults with different levels of concern about falling. The results indicated that there were significant differences in distributions of posture topics between groups of older adults with different levels of concern about falling. In addition, the transitions of posture topics over daytime and nighttime revealed temporal regularities of posture behavior patterns of older adult’s active and inactive status, which were substantially different for older adults with different levels of concern about falling. Finally, the level of concern about falling was accurately determined with an accuracy of 71.2% based on the distributions of posture topics combined with the mobility performance metrics of walking behaviors and demographic information.
更多查看译文
关键词
Concern about falling,posture behavior pattern,telehealth,topic modeling,wearable accelerometer
AI 理解论文
溯源树
样例
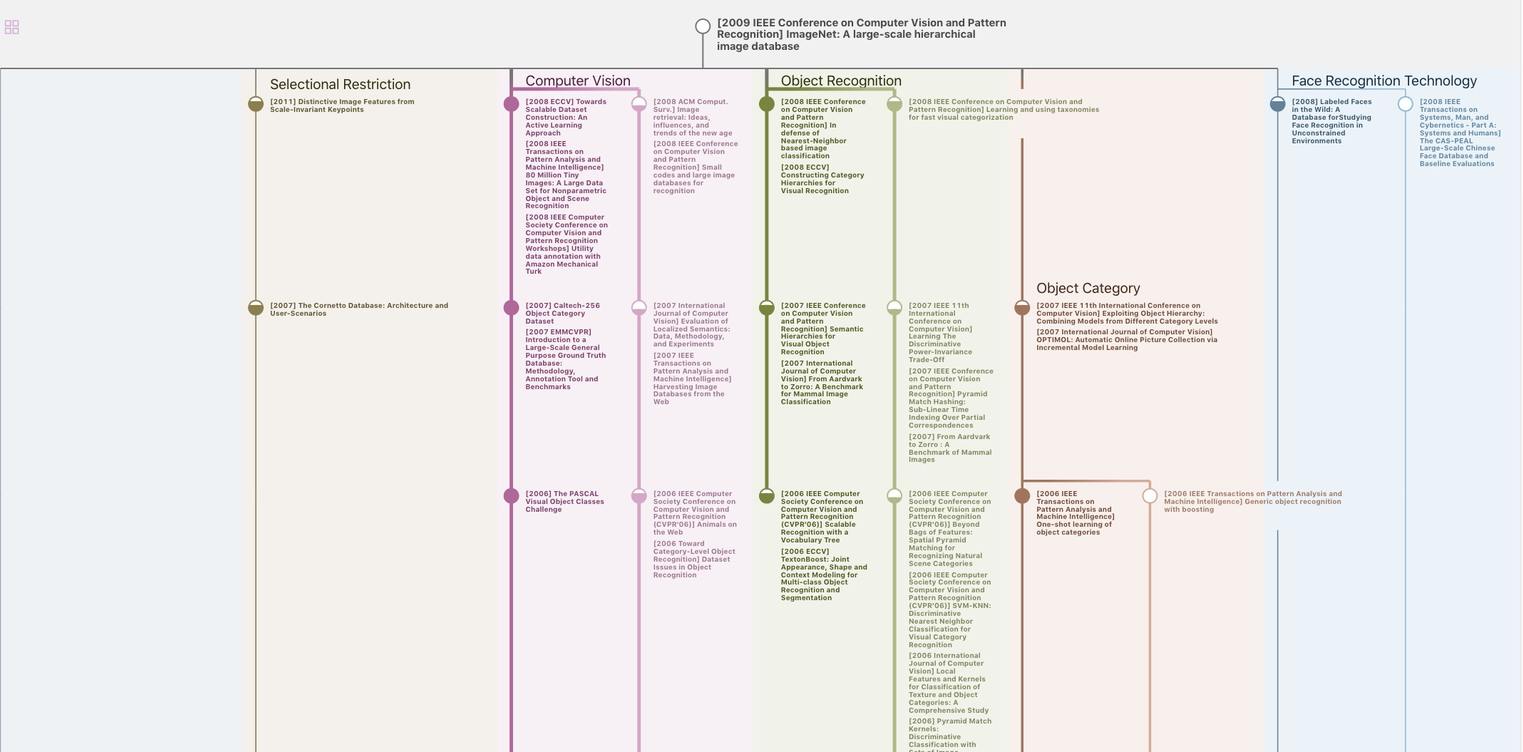
生成溯源树,研究论文发展脉络
Chat Paper
正在生成论文摘要