From Local Binary Patterns to Pixel Difference Networks for Efficient Visual Representation Learning
arxiv(2023)
摘要
LBP is a successful hand-crafted feature descriptor in computer vision. However, in the deep learning era, deep neural networks, especially convolutional neural networks (CNNs) can automatically learn powerful task-aware features that are more discriminative and of higher representational capacity. To some extent, such hand-crafted features can be safely ignored when designing deep computer vision models. Nevertheless, due to LBP's preferable properties in visual representation learning, an interesting topic has arisen to explore the value of LBP in enhancing modern deep models in terms of efficiency, memory consumption, and predictive performance. In this paper, we provide a comprehensive review on such efforts which aims to incorporate the LBP mechanism into the design of CNN modules to make deep models stronger. In retrospect of what has been achieved so far, the paper discusses open challenges and directions for future research.
更多查看译文
关键词
pixel difference networks,efficient visual representation learning,local binary patterns
AI 理解论文
溯源树
样例
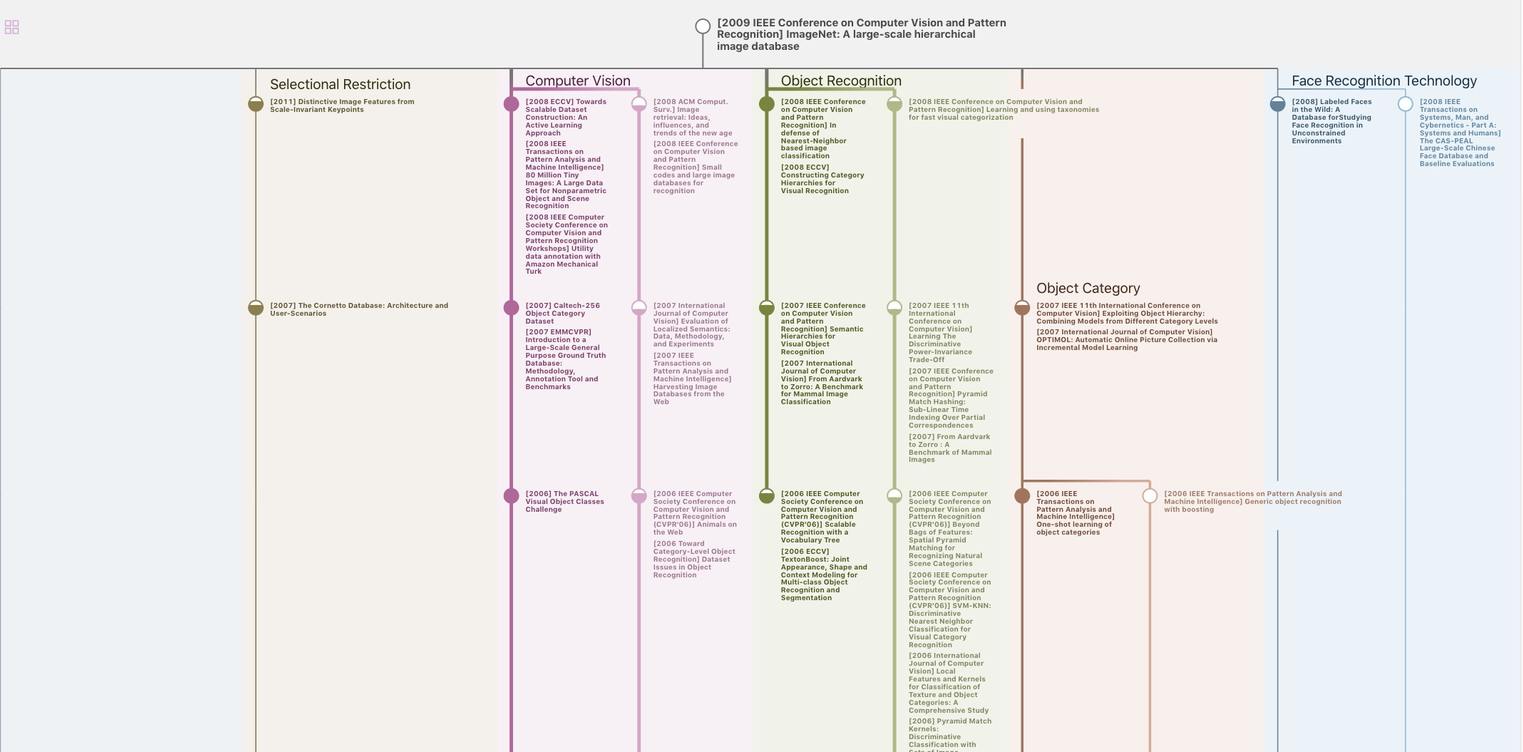
生成溯源树,研究论文发展脉络
Chat Paper
正在生成论文摘要