Muti-Agent Proximal Policy Optimization for Data Freshness in UAV-assisted Networks
2023 IEEE International Conference on Communications Workshops (ICC Workshops)(2023)
摘要
Unmanned aerial vehicles (UAVs) are seen as a promising technology to perform a wide range of tasks in wireless communication networks. In this work, we consider the deployment of a group of UAVs to collect the data generated by IoT devices. Specifically, we focus on the case where the collected data is time-sensitive, and it is critical to maintain its timeliness. Our objective is to optimally design the UAVs' trajectories and the subsets of visited IoT devices such as the global Age-of-Updates (AoU) is minimized. To this end, we formulate the studied problem as a mixed-integer nonlinear programming (MINLP) under time and quality of service constraints. To efficiently solve the resulting optimization problem, we investigate the cooperative Multi-Agent Reinforcement Learning (MARL) framework and propose an RL approach based on the popular on-policy Reinforcement Learning (RL) algorithm: Policy Proximal Optimization (PPO). Our approach leverages the centralized training decentralized execution (CTDE) framework where the UAVs learn their optimal policies while training a centralized value function. Our simulation results show that the proposed MAPPO approach reduces the global AoU by at least a factor of 1/2 compared to conventional off-policy reinforcement learning approaches.
更多查看译文
关键词
Actor-Critic,Age-of-Updates,MARL,Policy Gradient,PPO,UAV-assisted Network
AI 理解论文
溯源树
样例
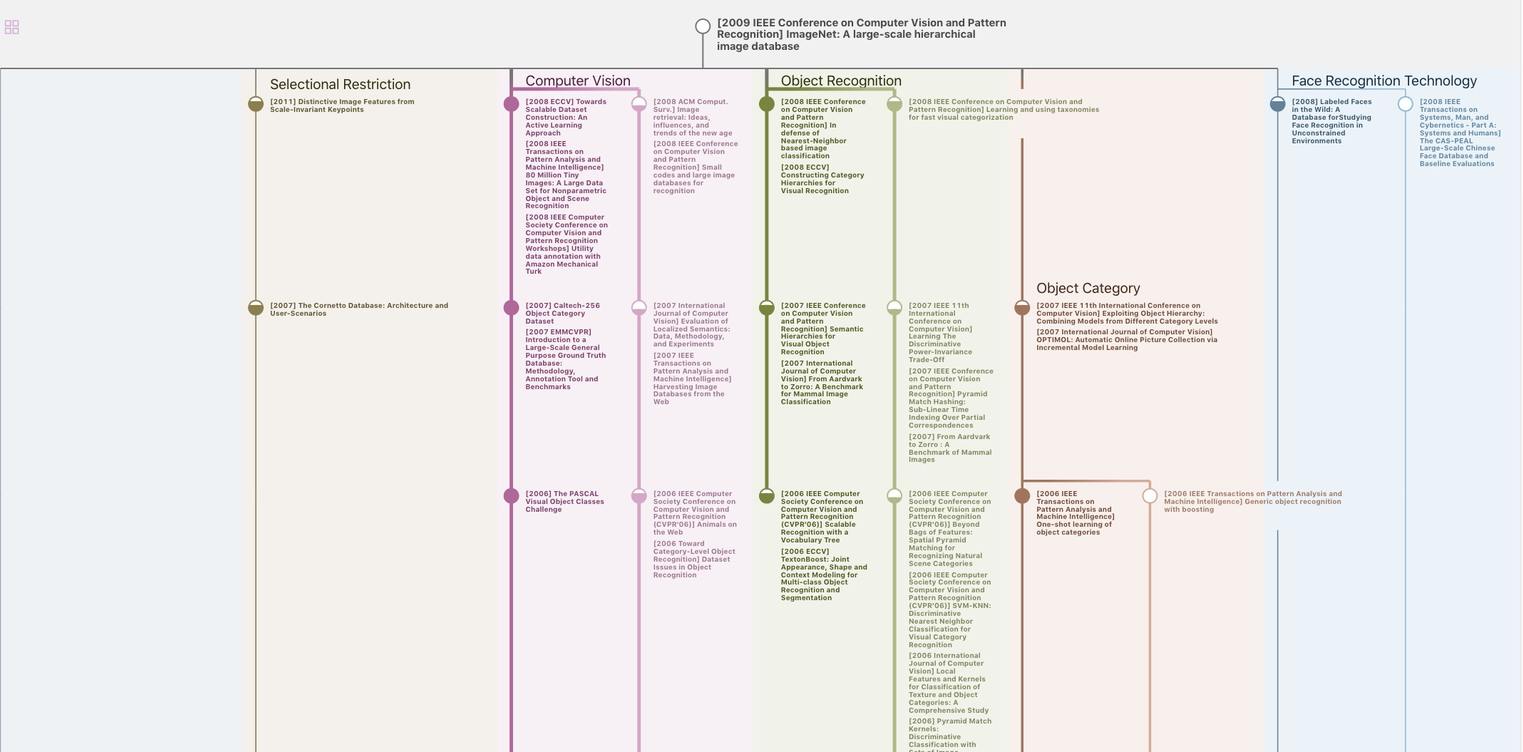
生成溯源树,研究论文发展脉络
Chat Paper
正在生成论文摘要