DiffusionAD: Denoising Diffusion for Anomaly Detection
arxiv(2023)
摘要
Anomaly detection is widely applied due to its remarkable effectiveness and efficiency in meeting the needs of real-world industrial manufacturing. We introduce a new pipeline, DiffusionAD, to anomaly detection. We frame anomaly detection as a ``noise-to-norm'' paradigm, in which anomalies are identified as inconsistencies between a query image and its flawless approximation. Our pipeline achieves this by restoring the anomalous regions from the noisy corrupted query image while keeping the normal regions unchanged. DiffusionAD includes a denoising sub-network and a segmentation sub-network, which work together to provide intuitive anomaly detection and localization in an end-to-end manner, without the need for complicated post-processing steps. Remarkably, during inference, this framework delivers satisfactory performance with just one diffusion reverse process step, which is tens to hundreds of times faster than general diffusion methods. Extensive evaluations on standard and challenging benchmarks including VisA and DAGM show that DiffusionAD outperforms current state-of-the-art paradigms, demonstrating the effectiveness and generalizability of the proposed pipeline.
更多查看译文
关键词
diffusionad,detection
AI 理解论文
溯源树
样例
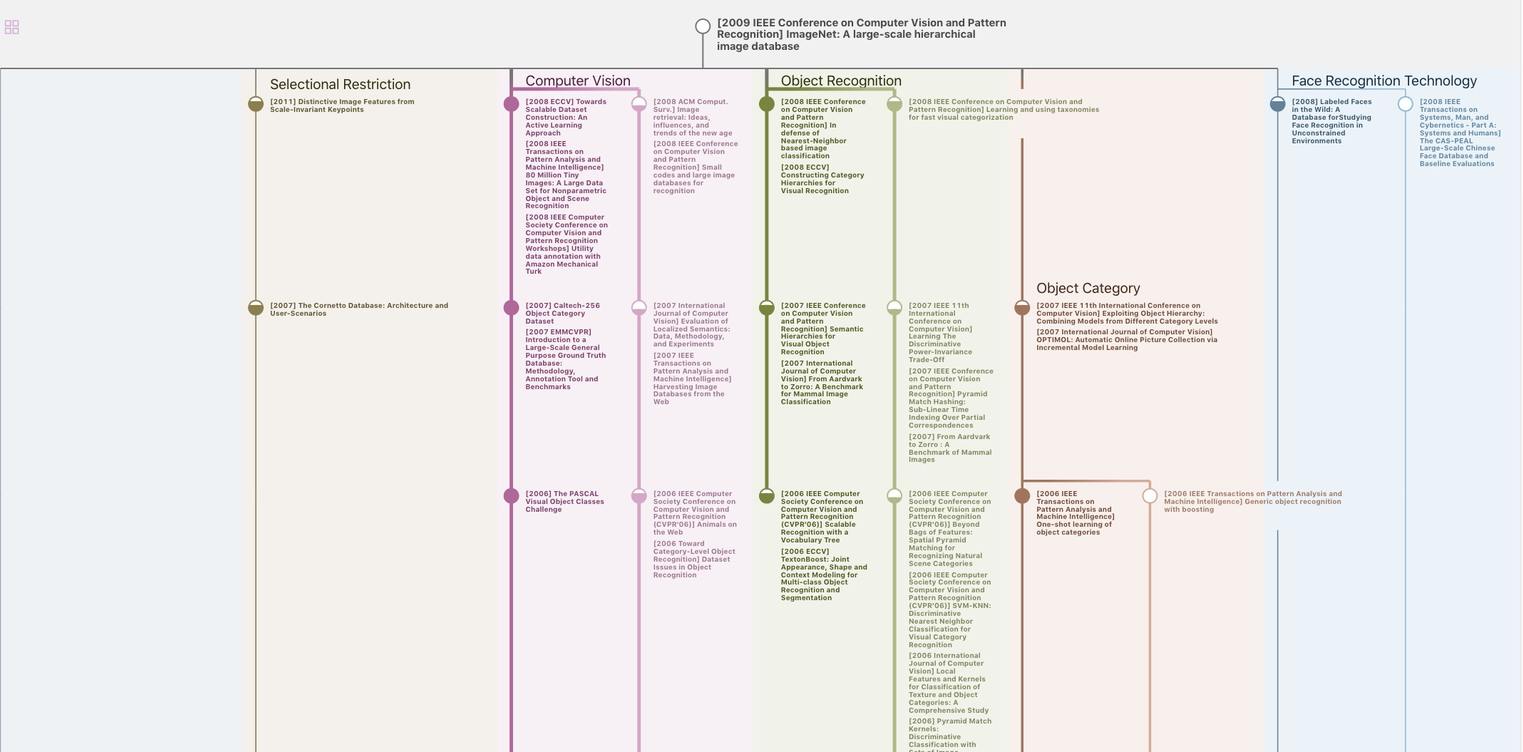
生成溯源树,研究论文发展脉络
Chat Paper
正在生成论文摘要