A new inexact gradient descent method with applications to nonsmooth convex optimization
OPTIMIZATION METHODS & SOFTWARE(2024)
摘要
The paper proposes and develops a novel inexact gradient method (IGD) for minimizing C-1-smooth functions with Lipschitzian gradients, i.e. for problems of $ {\mathcal {C}}<^>{1,1} $ C-1,C-1 optimization. We show that the sequence of gradients generated by IGD converges to zero. The convergence of iterates to stationary points is guaranteed under the Kurdyka-Lojasiewicz (KL) property of the objective function with convergence rates depending on the KL exponent. The newly developed IGD is applied to designing two novel gradient-based methods of nonsmooth convex optimization such as the inexact proximal point methods (GIPPM) and the inexact augmented Lagrangian method (GIALM) for convex programs with linear equality constraints. These two methods inherit global convergence properties from IGD and are confirmed by numerical experiments to have practical advantages over some well-known algorithms of nonsmooth convex optimization.
更多查看译文
关键词
Inexact gradient methods,inexact proximal point methods,inexact augmented Lagrangian methods,C-1,C-1 optimization,nonsmooth convex optimization
AI 理解论文
溯源树
样例
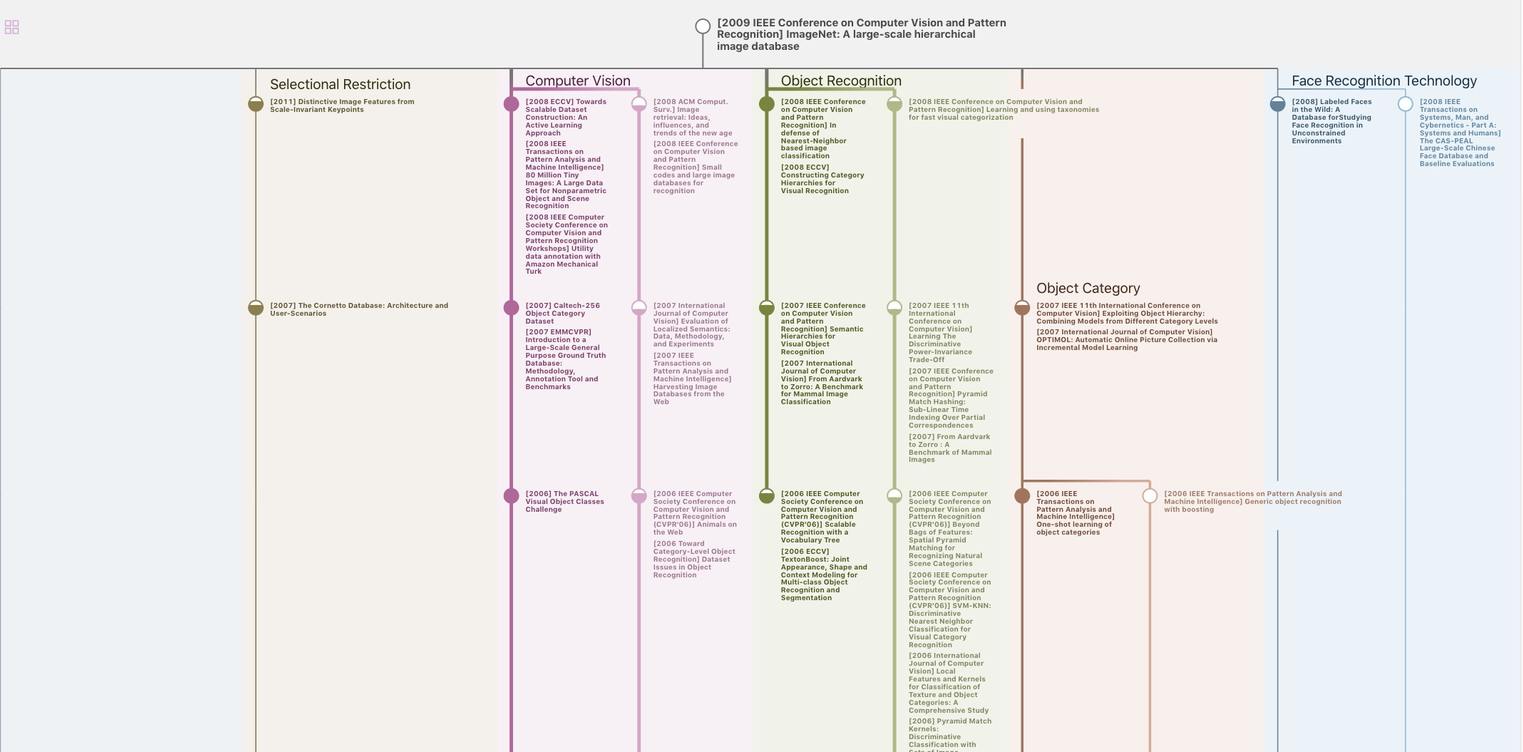
生成溯源树,研究论文发展脉络
Chat Paper
正在生成论文摘要