Design considerations for observational studies of drugs for advanced cancer.
Pharmacoepidemiology and drug safety(2023)
摘要
Post-authorisation studies complement the clinical development programme of a drug by studying larger, more diverse populations over a longer time than studies of the programme and can rely on secondary use of routinely collected patient data (i.e., real-world data [RWD]). Often, like the randomised controlled trials (RCTs) that may precede them, their aim is to estimate causal effects1 of a drug on safety and/or efficacy outcomes. Here, we present three challenges of RWD analyses of patients with advanced cancer and assess whether similar challenges occur in RCTs and how they are managed. Then we propose target trial emulation2 as an analytical framework for overcoming the challenges in post-authorisation studies. Treatment of patients with advanced cancer is characterised by three factors: (1) Patients often receive drugs in sequence as “lines of treatment” (LoTs), which consist of one or several drugs, continued until a pre-specified duration, tumour progression, toxicity, or a decision to stop treatment is reached. Sometimes drugs in a LoT are started at different times because of logistics or the need of additional diagnostics. For example, in patients with advanced colorectal cancer treated with first-line chemotherapy plus anti-epidermal growth factor receptor (EGFR) therapy, start of anti-EGFR is sometimes delayed because its indication needs to be verified by additional testing. (2) If clinically indicated, the patient often proceeds to a subsequent LoT when the previous LoT is no longer indicated (e.g., progression or toxicity). For example, several drugs are approved to treat metastatic castration-resistant prostate cancer – androgen receptor-targeted drugs, chemotherapy, radioactive agents, poly (ADP-ribose) polymerase inhibitors, and immunotherapy3 – which can be administered almost in any sequence as several LoTs. (3) Patients receiving later LoTs have a substantially worse prognosis than those receiving earlier LoTs because the LoT reflects the natural evolution of the disease (e.g., tumour can spread to distant sites and become resistant to treatments). The first challenge is studying a drug that can be a component of different LoTs. If the average causal effect across several LoTs is estimated and the distribution of LoTs differs between exposure groups, baseline confounding can occur (because of the prognostic nature of LoTs). Additionally, if the drug's effect is not the same in all LoTs (effect modification), the average causal effect across several LoTs may not be relevant for clinical practice – where therapeutic decisions are made on the basis of LoT – and may hinder transportability. Transportability is the process of extending inferences computed in one population to a second population with different characteristics.4 If the LoT is an effect modifier and the distribution of LoT differs between the population in which the effect was estimated and the target population(s), the estimated average causal effect across several LoTs is not transportable. Differences in LoT distribution can occur due, for example, to differences in reimbursement policies, time trends in drug prescription, or prescriber preferences. The design of RCTs commonly addresses this challenge by recruiting only patients eligible to receive the drug in a specific LoT. Such restriction avoids confounding by the LoT and generates useful information to estimate effects in other populations if the effect of the drug is not homogeneous across LoTs. The second challenge is that patients can receive additional LoTs after baseline, before the outcome of interest occurs, and such sequence of drugs may be determined by the efficacy and toxicity of previous LoTs. If we are interested in estimating the effect of a specific drug under complete adherence or if there are losses to follow-up, both baseline and post-baseline confounding can distort the identification of the causal effect. Whereas randomisation protects against baseline confounding in RCTs, it does not eliminate post-baseline confounding. Post-baseline confounding can arise from non-adherence if post-randomization prognostic factors that affect treatment decisions are unequally distributed across arms. RCTs address this challenge by specifying the post-randomization events (events occurring after treatment initiation, such as subsequent treatments that affect the interpretation or the existence of the measurements associated with the clinical question) and then by integrating such events in the treatment strategies to construct an estimand. For example, an RCT protocol can specify the sequence of treatments allowed in each study arm (no trial would halt treatment after the randomised drug is stopped if the patient is fit to continue treatment with another LoT) and even force some control by design of subsequent treatments (e.g., by specifying whether crossing over to the experimental drug is allowed in the control group). Specifically, RCTs compare not only drugs but treatment strategies that can comprise 1 or more drugs administered concomitantly or in sequence, as well as rules to guide treatment, applied at specific decision points. A proposed approach to identify the effect under complete adherence and/or under complete follow-up consists of censoring data from individuals when they deviate from the treatment strategy (note that stopping treatment for clinical reasons is not a deviation) or from the follow-up strategy and then adjusting for pre-randomisation and post-randomisation factors that predict adherence. If baseline treatments can affect post-randomisation factors, an adjustment must be made with g-methods, which include inverse probability weighting, g-estimation of structural nested models, and the g-formula, rather than with conventional statistical methods.5 The third challenge is studying the effect of a drug (vs. not receiving it) that is part of a multicomponent LoT and is started later than the other components. Because mortality in patients with advanced cancer is high, immortal time bias6 (a form of selection7) can occur in an observational setting because only patients who survive long enough will be able to start to use that drug. RCTs address this issue by estimating the intention-to-treat effect, effectively avoiding such immortal time bias by aligning the start of follow-up with the date of treatment assignment (date of randomization), rather than with the date of treatment initiation. The target trial emulation framework starts by articulating the research question in the form of a hypothetical pragmatic randomised trial. This target trial should have pre-specified eligibility criteria, treatment strategies, treatment assignment, start and end of follow-up, outcomes, causal contrast, and a data analysis plan. Subsequently, the RWD analysis will emulate the components of the target trial protocol by finding eligible individuals, assigning them to treatment strategies that are compatible with their data at baseline, following them up from treatment assignment (time zero) until outcome or end of follow-up, and conducting the same analysis as the corresponding target trial. The only difference is that the emulation requires adjustment for baseline confounders in an attempt to emulate the randomization of the target trial. By providing a well-defined estimand, this approach helps to identify and address possible sources of bias,8 and by comparing well-defined courses of action, it helps decision-makers. Appropriate handling of start of follow-up (time zero) and appropriate handling of time-varying confounding are among the most useful aspects of this approach. We present solutions to the challenges above through two applications. The first two challenges were present in PRECISE, a post-authorisation study that, as the primary objective, estimated the effect of Radium-223 on the incidence of fractures compared with other standard of care in Swedish men with metastatic castration-resistant prostate cancer.9 As part of our target trial, we specified the treatment strategies considering baseline drugs and decision rules based on post-baseline events, which included observed drug toxicity, tumor progression and overall health status (Table 1). Since safety was the focus of the study, the causal contrast (or estimand) of interest was the effect under complete adherence to such strategies.10 To diminish confounding by LoT and improve transportability of our estimates, we created a cohort of patients eligible for a first LoT (containing Ra-223 or other standard of care). Because Ra-223 could be used as any LoT during the study period, we also created cohorts of patients eligible for a second, third, and fourth LoT. In each LoT, we aligned time zero, eligibility assessment, and exposure assignment.11 Because patients in our database could be eligible for more than 1 LoT, we could let them contribute to 1 specific LoT or to all LoTs.2 For efficiency, we let patients contribute to all LoT-specific cohorts that they were eligible for. The observational analogue of the per-protocol effect was implemented by selecting the person-time compliant with each of the exposure strategies and using inverse probability weighting to adjust for potential baseline and post-baseline confounding. Because the effect of Ra-223 on the risk of fractures across LoTs was considered homogeneous, cohorts were pooled.9 Group 1: Initiate Ra-223. Patients can stop Ra-223 after 6 cycles or earlier in the event of toxicity, cancer progression, or worsening of the overall health status. Patients can start other systemic drugs for mCRPC after the initiation of Ra-223, when clinically indicated, but they can never be used while taking Ra-223. ADT with first-generation antiandrogens can be used at any time Group 2: Initiate other standard of care (docetaxel, cabazitaxel, enzalutamide, abiraterone, others). Patients are allowed to stop the standard of care and continue with other LoTs, with the exception of Ra-223, when clinically indicated. ADT with first-generation antiandrogens can be used at any time. Group 1. Initiate gemcitabine as first-line treatment. Initiate erlotinib (any dose) within the grace period: up to 12 weeks after gemcitabine initiation. Group 2. Initiate gemcitabine as first-line treatment within the grace period. Do not initiate erlotinib. Under both strategies, the decision to discontinue gemcitabine or erlotinib, as well as to initiate any additional therapies, is left to the patient's and physician's discretion. The third challenge was present in Petito et al.,12 who estimated the effect of adding erlotinib to gemcitabine on survival in patients with advanced pancreatic cancer. In routine care, erlotinib may be initiated a few weeks later than gemcitabine, and advanced pancreatic cancer is highly lethal. To avoid the potential for immortal time bias, Petito et al.12 defined time zero as the time when patients initiated gemcitabine and met the eligibility criteria. The exposure strategies allowed erlotinib to be started up to 12 weeks after time zero (Table 1). Time zero, treatment assignment, and eligibility were aligned by classifying patients into treatment strategies that their baseline data were compatible with, effectively creating two copies (clones) of each eligible individual and by assigning one copy to each exposure strategy.13 Then, the person-time compliant with the assigned strategy was selected, and inverse probability weighting was used to adjust for potential baseline and post-baseline confounding, which is the same approach used in PRECISE.12 In conclusion, we recommend the following target trial emulation framework in RWD studies of drugs for advanced cancer: create LoT-specific cohorts; align the beginning of follow-up (time zero), eligibility criteria, and exposure assignment; and use g-methods to estimate effects under complete adherence and under complete follow-up, especially in safety-focused studies, like PASs. In the absence of unmeasured confounding, measurement error, and model misspecification (including models used to handle missing data), this approach will help identify the causal effect of well-specified treatment strategies.14
更多查看译文
关键词
causal inference,real world evidence,target trial emulation
AI 理解论文
溯源树
样例
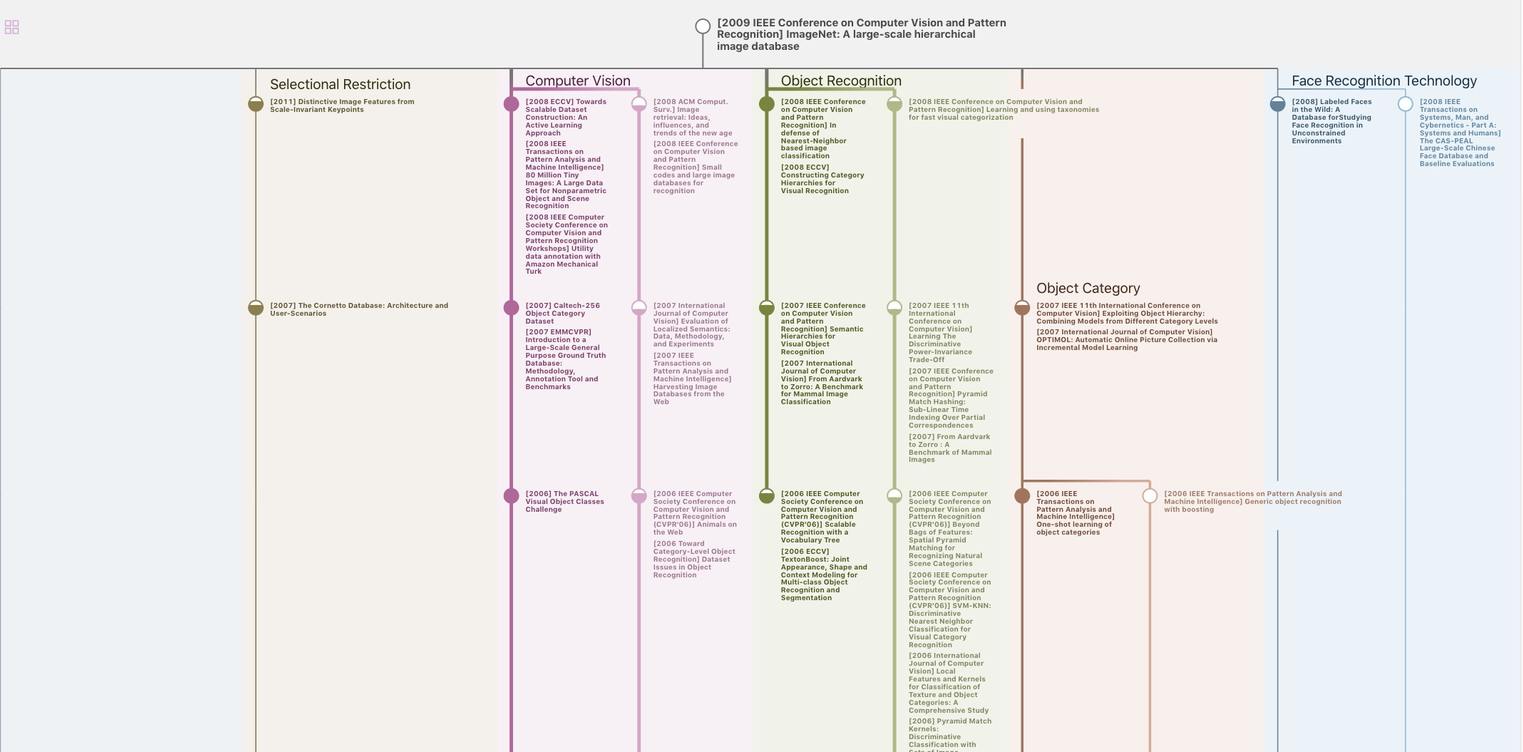
生成溯源树,研究论文发展脉络
Chat Paper
正在生成论文摘要