Block Mirror Stochastic Gradient Method For Stochastic Optimization
Journal of Scientific Computing(2023)
摘要
In this paper, a block mirror stochastic gradient method is developed to solve stochastic optimization problems involving convex and nonconvex cases, where the feasible set and the variables are treated as multiple blocks. The proposed method combines the features of the classic mirror descent stochastic method and the block coordinate gradient descent method. Acquiring the stochastic gradient information by stochastic oracles, our method updates all the blocks of variables in the Gauss–Seidel type. We establish the convergence for both convex and nonconvex cases. The analysis of our method is challenging because the typical unbiasedness assumption of stochastic gradient fails to hold in the Gauss–Seidel renewal type and requires more specific assumptions. The proposed algorithm is tested on the conditional value-at-risk problem and the stochastic LASSO problem to demonstrate the efficiency of our algorithm.
更多查看译文
关键词
Convex optimization, Nonconvex optimization, Stochastic optimization, Stochastic gradient, Block coordinate descent
AI 理解论文
溯源树
样例
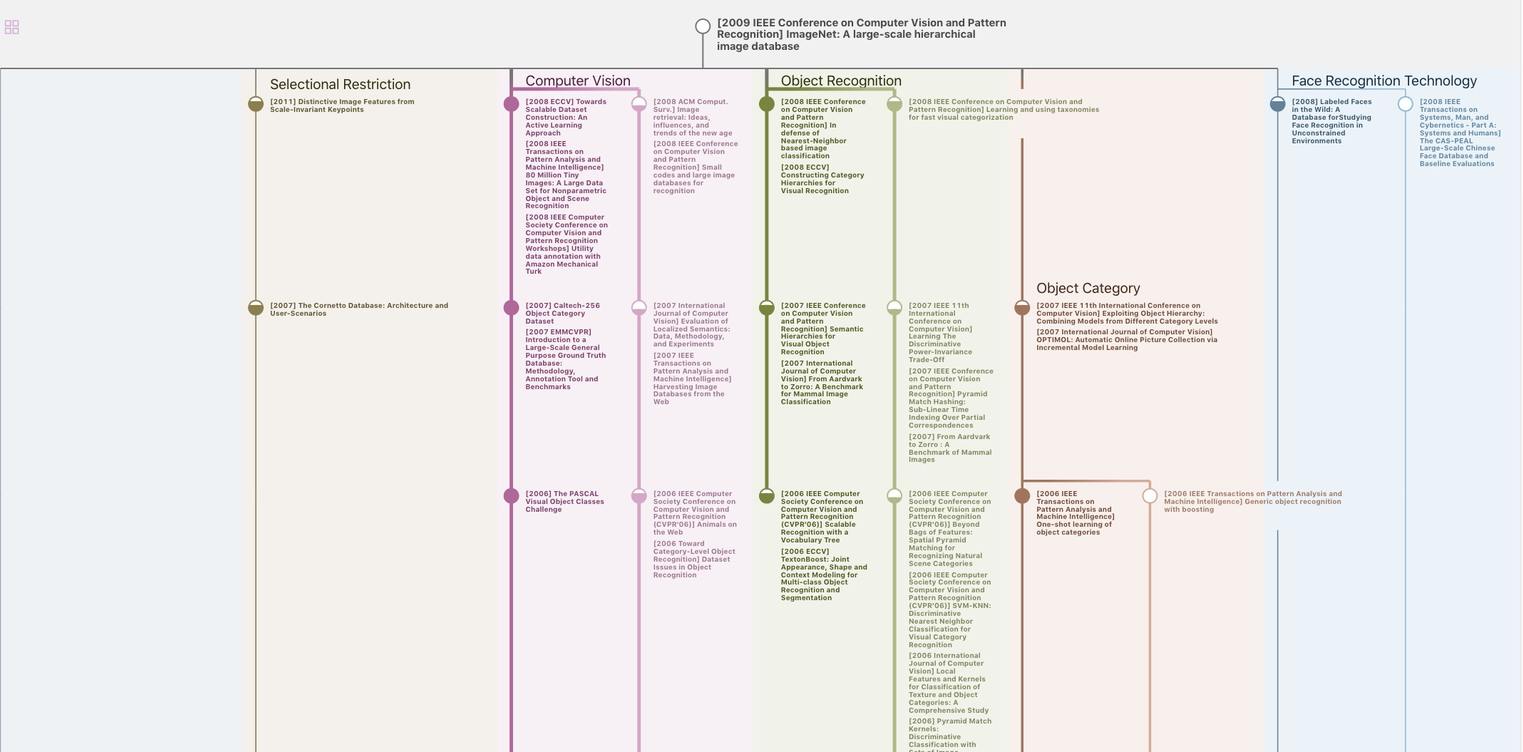
生成溯源树,研究论文发展脉络
Chat Paper
正在生成论文摘要