DeltaScore: Evaluating Story Generation with Differentiating Perturbations
arxiv(2023)
摘要
Various evaluation metrics exist for natural language generation tasks, but they have limited utility for story generation since they generally do not correlate well with human judgments and do not measure fine-grained story aspects, such as fluency versus relatedness, as they are intended to assess overall generation quality. In this paper, we propose deltascore, an approach that utilizes perturbation to evaluate fine-grained story aspects. Our core idea is based on the hypothesis that the better the story performs in a specific aspect (e.g., fluency), the more it will be affected by a particular perturbation (e.g., introducing typos). To measure the impact, we calculate the likelihood difference between the pre- and post-perturbation stories using a language model. We evaluate deltascore against state-of-the-art model-based and traditional similarity-based metrics across multiple story domains, and investigate its correlation with human judgments on five fine-grained story aspects: fluency, coherence, relatedness, logicality, and interestingness. Our results demonstrate that deltascore performs impressively in evaluating fine-grained story aspects, and we discovered a striking outcome where a specific perturbation appears to be highly effective in measuring most aspects.
更多查看译文
AI 理解论文
溯源树
样例
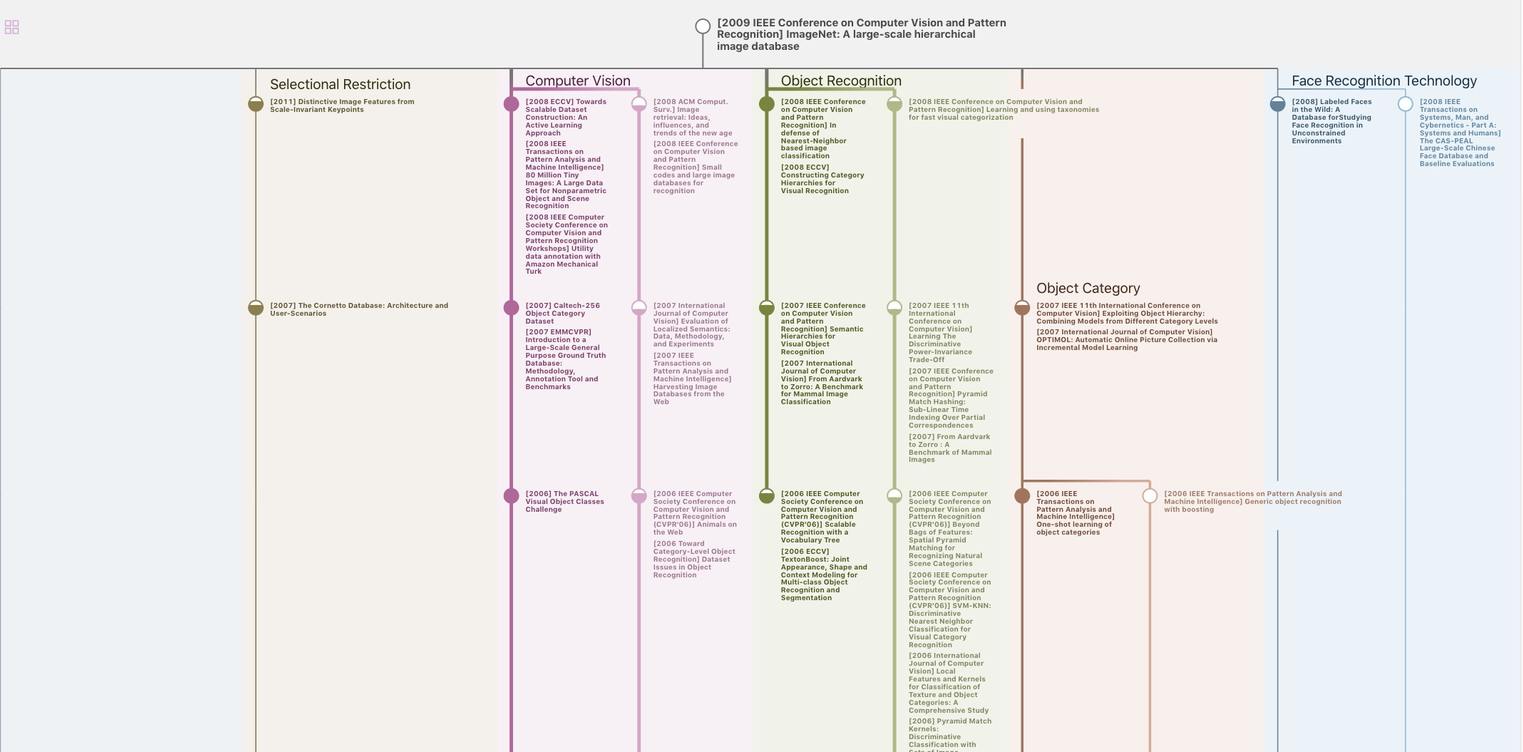
生成溯源树,研究论文发展脉络
Chat Paper
正在生成论文摘要