Fairness-aware Differentially Private Collaborative Filtering
COMPANION OF THE WORLD WIDE WEB CONFERENCE, WWW 2023(2023)
摘要
Recently, there has been an increasing adoption of differential privacy guided algorithms for privacy-preserving machine learning tasks. However, the use of such algorithms comes with trade-offs in terms of algorithmic fairness, which has been widely acknowledged. Specifically, we have empirically observed that the classical collaborative filtering method, trained by differentially private stochastic gradient descent (DP-SGD), results in a disparate impact on user groups with respect to different user engagement levels. This, in turn, causes the original unfair model to become even more biased against inactive users. To address the above issues, we propose DP-Fair, a two-stage framework for collaborative filtering based algorithms. Specifically, it combines differential privacy mechanisms with fairness constraints to protect user privacy while ensuring fair recommendations. The experimental results, based on Amazon datasets, and user history logs collected from Etsy, one of the largest e-commerce platforms, demonstrate that our proposed method exhibits superior performance in terms of both overall accuracy and user group fairness on both shallow and deep recommendation models compared to vanilla DP-SGD.
更多查看译文
关键词
Collaborative Filtering,Fairness,Differential Privacy
AI 理解论文
溯源树
样例
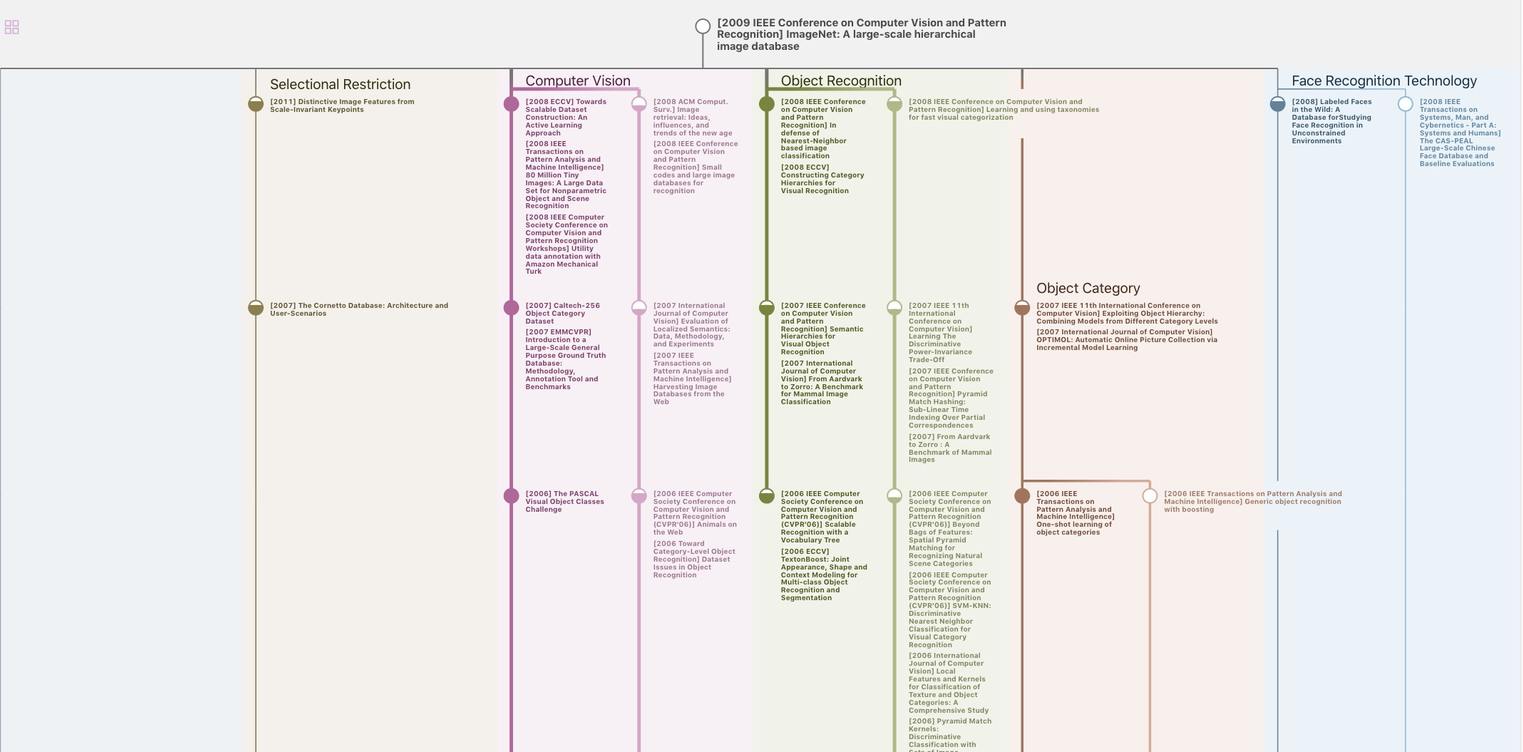
生成溯源树,研究论文发展脉络
Chat Paper
正在生成论文摘要