Deterministic Learning-Based Neural Control for Output-Constrained Strict-Feedback Nonlinear Systems
Science China Information Sciences(2024)
摘要
This study investigates the deterministic learning (DL)-based output-feedback neural control for a class of nonlinear sampled-data systems with prescribed performance (PP). Specifically, first, a sampled-data observer is employed to estimate the unavailable system states for the Euler discretization model of the transformed system dynamics. Then, based on the observations and backstepping method, a discrete neural network (NN) controller is constructed to ensure system stability and achieve the desired tracking performance. The noncausal problem encountered during the controller deduction process is resolved using a command filter. Moreover, the regression characteristics of the NN input signals are demonstrated with the observed states. This ensures that the radial basis function NN, based on DL theory, meets the partial persistent excitation condition. Subsequently, a class of discrete linear time-varying systems is proven to be exponentially stable, achieving partial convergence of neural weights to their optimal/actual values. Consequently, accurate modeling of unknown closed-loop dynamics is achieved along the system trajectory from the output-feedback control. Finally, a knowledge-based controller is developed using the modeling results. This controller not only enhances the control performance but also ensures the PP of the tracking error. The effectiveness of the scheme is illustrated through simulation results.
更多查看译文
关键词
deterministic learning,neural networks,adaptive neural control,predefined performance,nonlinear sampled-data systems
AI 理解论文
溯源树
样例
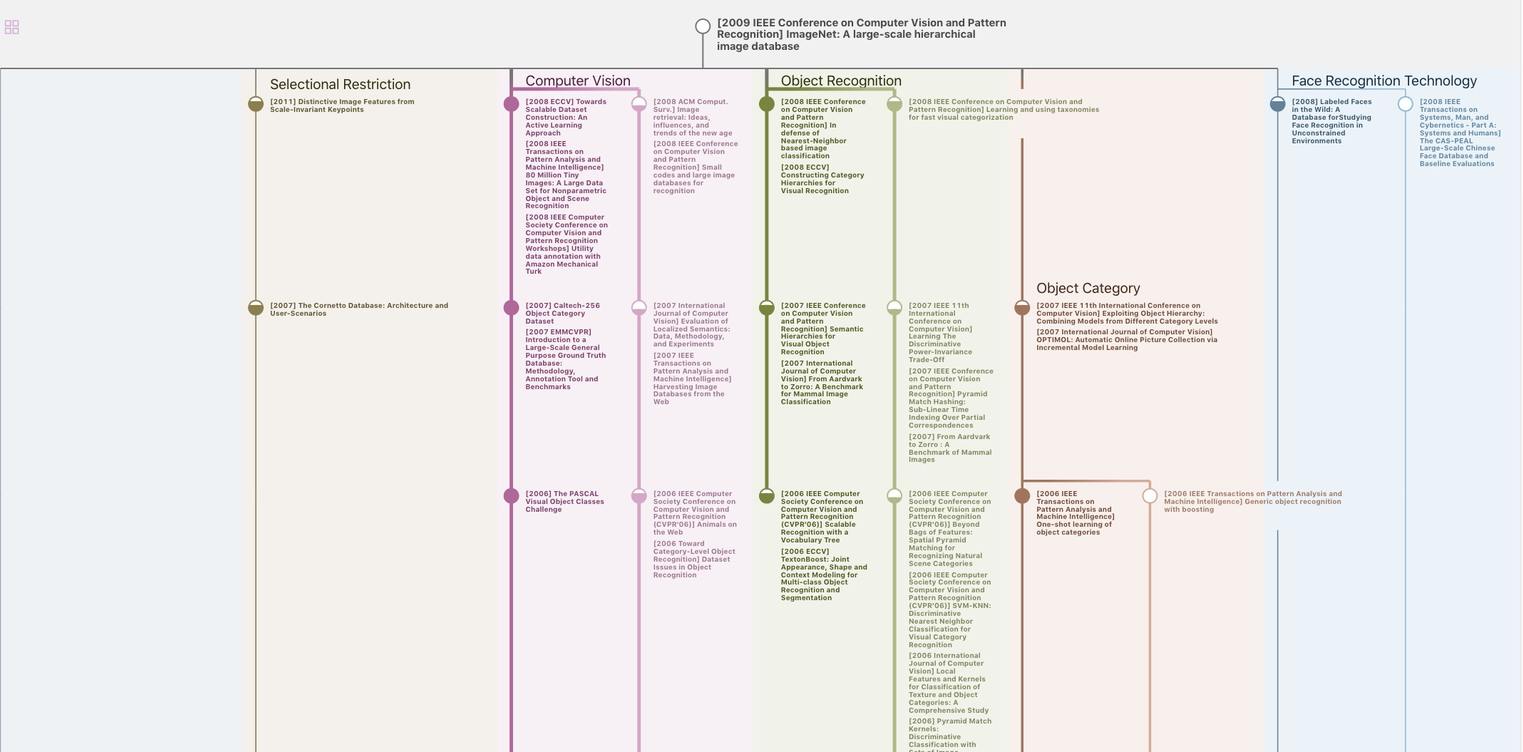
生成溯源树,研究论文发展脉络
Chat Paper
正在生成论文摘要