Implicit regularization of a deep augmented neural network model for human motion prediction
Applied Intelligence(2023)
摘要
Predicting human motion based on past observed motion is one of the challenging issues in computer vision and graphics. Existing research works are dealing with this issue by using discriminative models and showing the results for cases that follow a homogeneous distribution (in distribution) and not discussing the issues of the domain shift problem, where training and testing data follow a heterogeneous (out of distribution) problem, which is the reality when such models are used in practice. However, recent research proposed addressing domain shift issues by augmenting the discriminative model with a generative model and obtained better results. In the present investigation, we propose regularizing the extended network by inserting linear layers to minimize the rank of the latent space and train the entire end-to-end network. We regularize the network to strengthen the model to deal effectively with domain shift scenarios. Both training and testing data come from different distribution sets; to deal with this, we toughen our network by adding the extra linear layers to the network encoder. We tested our model with the benchmark datasets, CMU Motion Capture and Human3.6M, and proved that our model outperforms 14 OoD actions of H3.6M and 7 OoD actions of CMU MoCap in terms of the Euclidean distance calculated between predicted and ground truth joint angle values. Our average results of 14 OoD actions for short-term (80, 160, 320, 400) are 0.34, 0.6, 0.96, 1.07, and for CMU MoCap of 7 OoD actions for short-term and long term (80, 160, 320, 400, 1000) are 0.28, 0.45, 0.77, 0.89, 1.46. All these results are much better than the other state-of-the-art results.
更多查看译文
关键词
Linear layers,Human motion,Out-of-distribution,In-distribution,Encoder-decoder,Graph convolution network
AI 理解论文
溯源树
样例
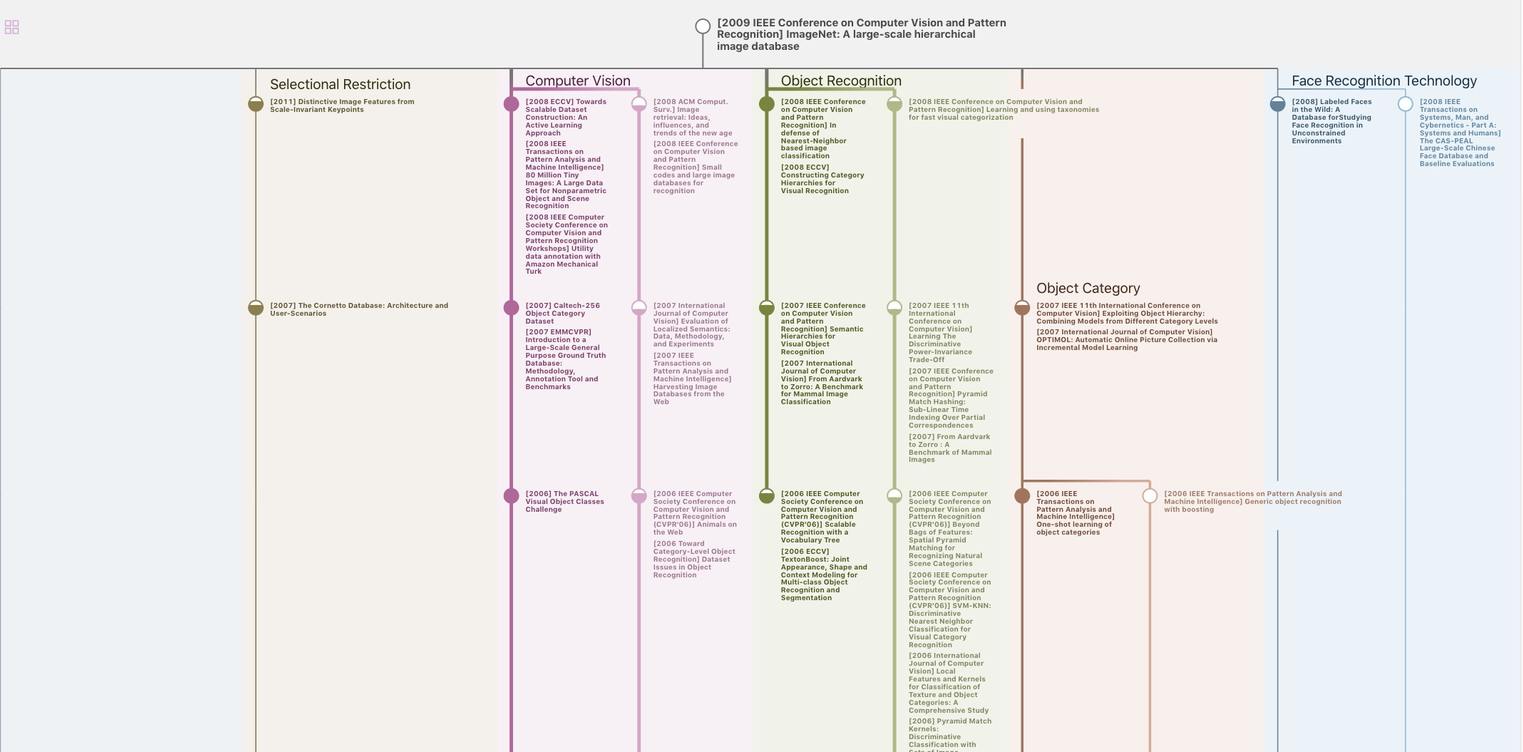
生成溯源树,研究论文发展脉络
Chat Paper
正在生成论文摘要