CoLT5: Faster Long-Range Transformers with Conditional Computation.
Conference on Empirical Methods in Natural Language Processing(2023)
Abstract
Many natural language processing tasks benefit from long inputs, but processing long documents with Transformers is expensive -- not only due to quadratic attention complexity but also from applying feedforward and projection layers to every token. However, not all tokens are equally important, especially for longer documents. We propose CoLT5, a long-input Transformer model that builds on this intuition by employing conditional computation, devoting more resources to important tokens in both feedforward and attention layers. We show that CoLT5 achieves stronger performance than LongT5 with much faster training and inference, achieving SOTA on the long-input SCROLLS benchmark. Moreover, CoLT5 can effectively and tractably make use of extremely long inputs, showing strong gains up to 64k input length.
MoreTranslated text
Key words
transformers,long-range long-range,conditional computation
AI Read Science
Must-Reading Tree
Example
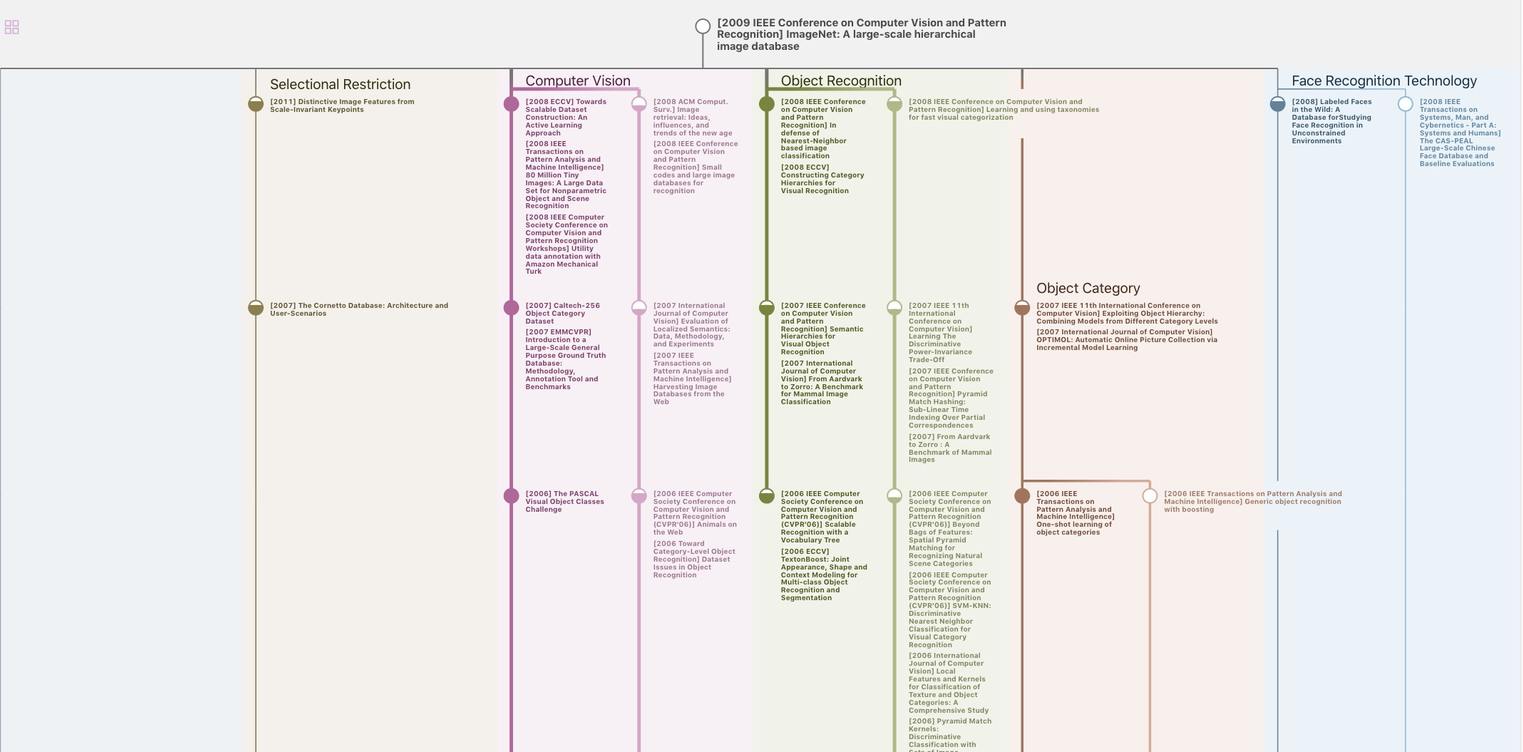
Generate MRT to find the research sequence of this paper
Chat Paper
Summary is being generated by the instructions you defined