Measurement Optimization under Uncertainty using Deep Reinforcement Learning
arxiv(2023)
摘要
Optimal sensor placement enhances the efficiency of a variety of applications for monitoring dynamical systems. It has been established that deterministic solutions to the sensor placement problem are insufficient due to the many uncertainties in system input and parameters that affect system response sensor measurements. Accounting for the uncertainties in this typically expensive optimization is challenging due to computational intractability. This study proposes a stochastic environment in the form of a Markov Decision Process and a sensor placement agent that aims to maximize the information gain from placing a particular number of sensors of different types within the system. The agent is trained to maximize its reward based on an information-theoretic reward function. To verify the efficacy, the approach is applied to place a set of heterogeneous sensors in a shear building model. This methodology can be used to accommodate uncertainties in the sensor placement problem in real-world systems.
更多查看译文
关键词
deep reinforcement,uncertainty,optimization,measurement,learning
AI 理解论文
溯源树
样例
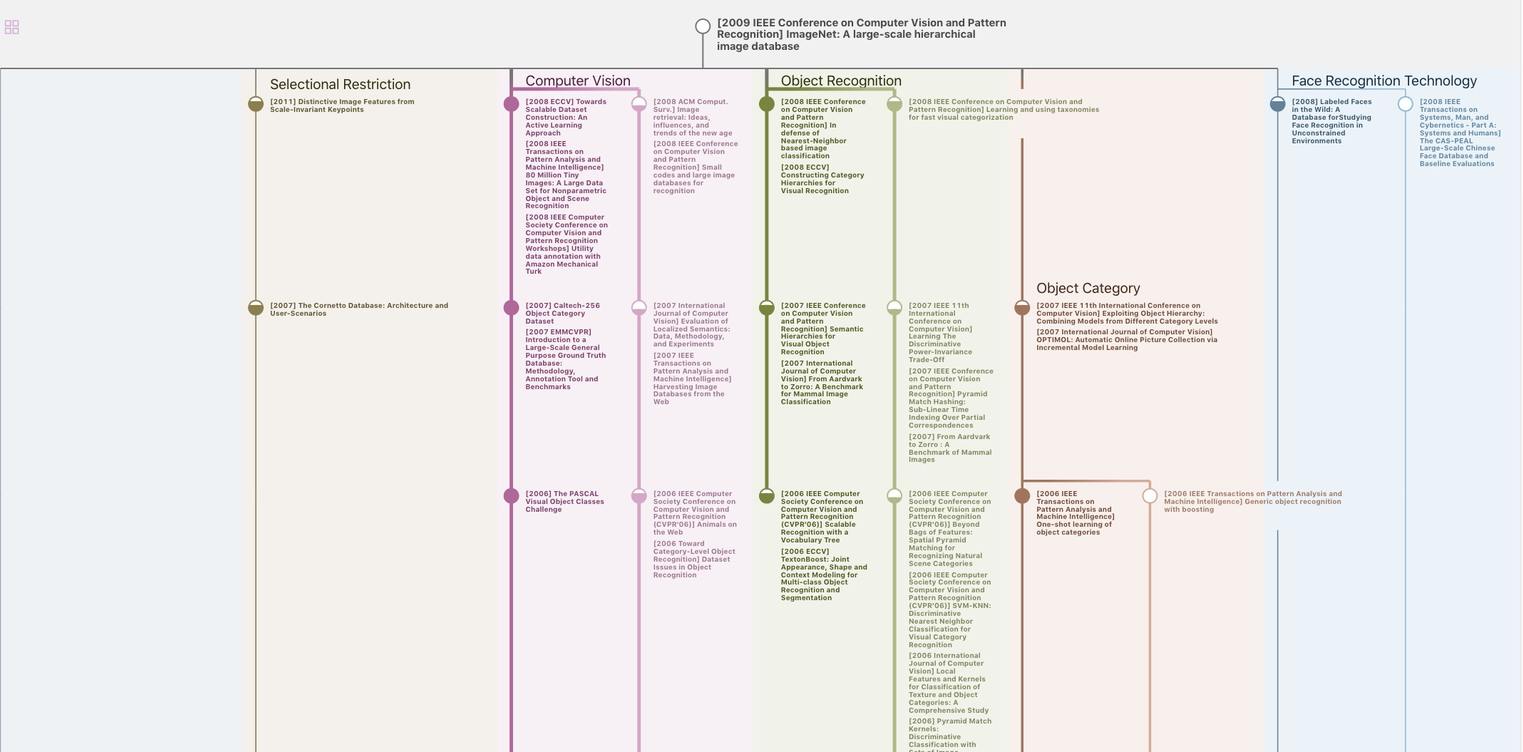
生成溯源树,研究论文发展脉络
Chat Paper
正在生成论文摘要