Resting-state SEEG-based brain network analysis for the detection of epileptic area.
Journal of neuroscience methods(2023)
摘要
BACKGROUND:Most epilepsy research is based on interictal or ictal functional connectivity. However, prolonged electrode implantation may affect patients' health and the accuracy of epileptic zone identification. Brief resting-state SEEG recordings reduce the observation of epileptic discharges by reducing electrode implantation and other seizure-inducing interventions.
NEW METHOD:The location coordinates of SEEG in the brain were identified using CT and MRI. Based on undirected brain network connectivity, five functional connectivity measures and data feature vector centrality were calculated. Network connectivity was calculated from multiple perspectives of linear correlation, information theory, phase, and frequency, and the relative influence of nodes on network connectivity was considered. We investigated the potential value of resting-state SEEG for epileptic zone identification by comparing the differences between epileptic and non-epileptic zones, as well as the differences between patients with different surgical outcomes.
RESULTS:By comparing the centrality of brain network connectivity between epileptic and non-epileptic zones, we found significant differences in the distribution of brain networks between the two zones. There was a significant difference in brain network between patients with good surgical outcomes and those with poor surgical outcomes (p < 0.01). By combining support vector machines with static node importance, we predicted an AUC of 0.94 ± 0.08 for the epilepsy zone.
CONCLUSIONS AND SIGNIFICANCE:The results illustrated that nodes in epileptic zones are distinct from those in non-epileptic zones. Analysis of resting-state SEEG data and the importance of nodes in the brain network may contribute to identifying the epileptic zone and predicting the outcome.
更多查看译文
AI 理解论文
溯源树
样例
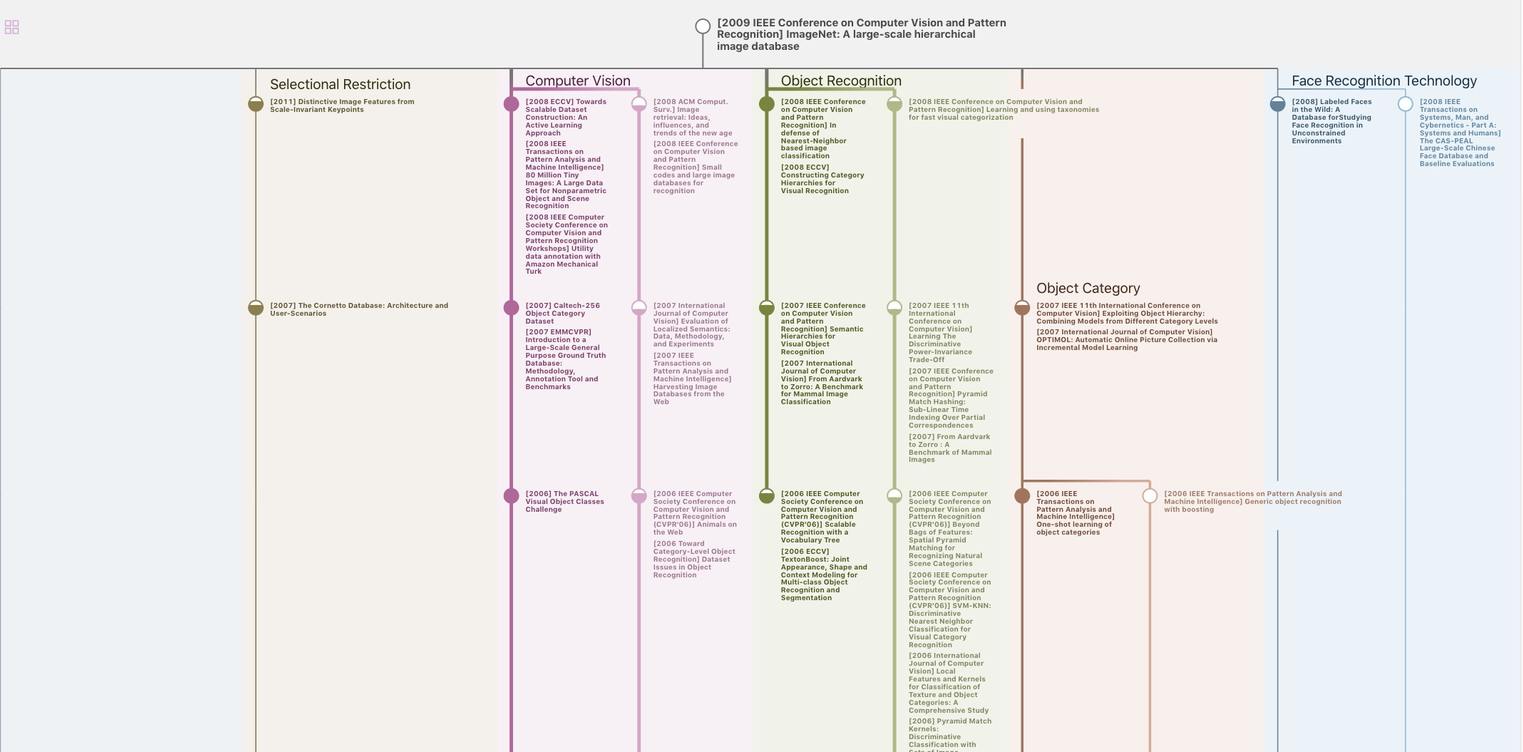
生成溯源树,研究论文发展脉络
Chat Paper
正在生成论文摘要