Low Dose of Contrast Agent and Low Radiation Liver Computed Tomography with Deep-Learning-based Contrast Boosting Model in Participants at High-Risk for Hepatocellular Carcinoma: Prospective, Randomized, Double-Blind Study.
European radiology(2023)
Abstract
To investigate the image quality and lesion conspicuity of a deep-learning-based contrast-boosting (DL-CB) algorithm on double-low-dose (DLD) CT of simultaneous reduction of radiation and contrast doses in participants at high-risk for hepatocellular carcinoma (HCC). Participants were recruited and underwent four-phase dynamic CT (NCT04722120). They were randomly assigned to either standard-dose (SD) or DLD protocol. All CT images were initially reconstructed using iterative reconstruction, and the images of the DLD protocol were further processed using the DL-CB algorithm (DLD-DL). The primary endpoint was the contrast-to-noise ratio (CNR), the secondary endpoint was qualitative image quality (noise, hepatic lesion, and vessel conspicuity), and the tertiary endpoint was lesion detection rate. The t-test or repeated measures analysis of variance was used for analysis. Sixty-eight participants with 57 focal liver lesions were enrolled (20 with HCC and 37 with benign findings). The DLD protocol had a 19.8 • Deep-learning-based contrast-boosting algorithm on double-low-dose CT provided an improved contrast-to-noise ratio compared to standard-dose CT. • The detection rate of focal liver lesions was not significantly differed between standard-dose CT and a deep-learning-based contrast-boosting algorithm on double-low-dose CT. • Double-low-dose CT without a deep-learning algorithm presented lower CNR and worse image quality.
MoreTranslated text
Key words
Tomography,X-ray computed,Deep learning,Contrast media,Hepatocellular carcinoma,Prospective study
AI Read Science
Must-Reading Tree
Example
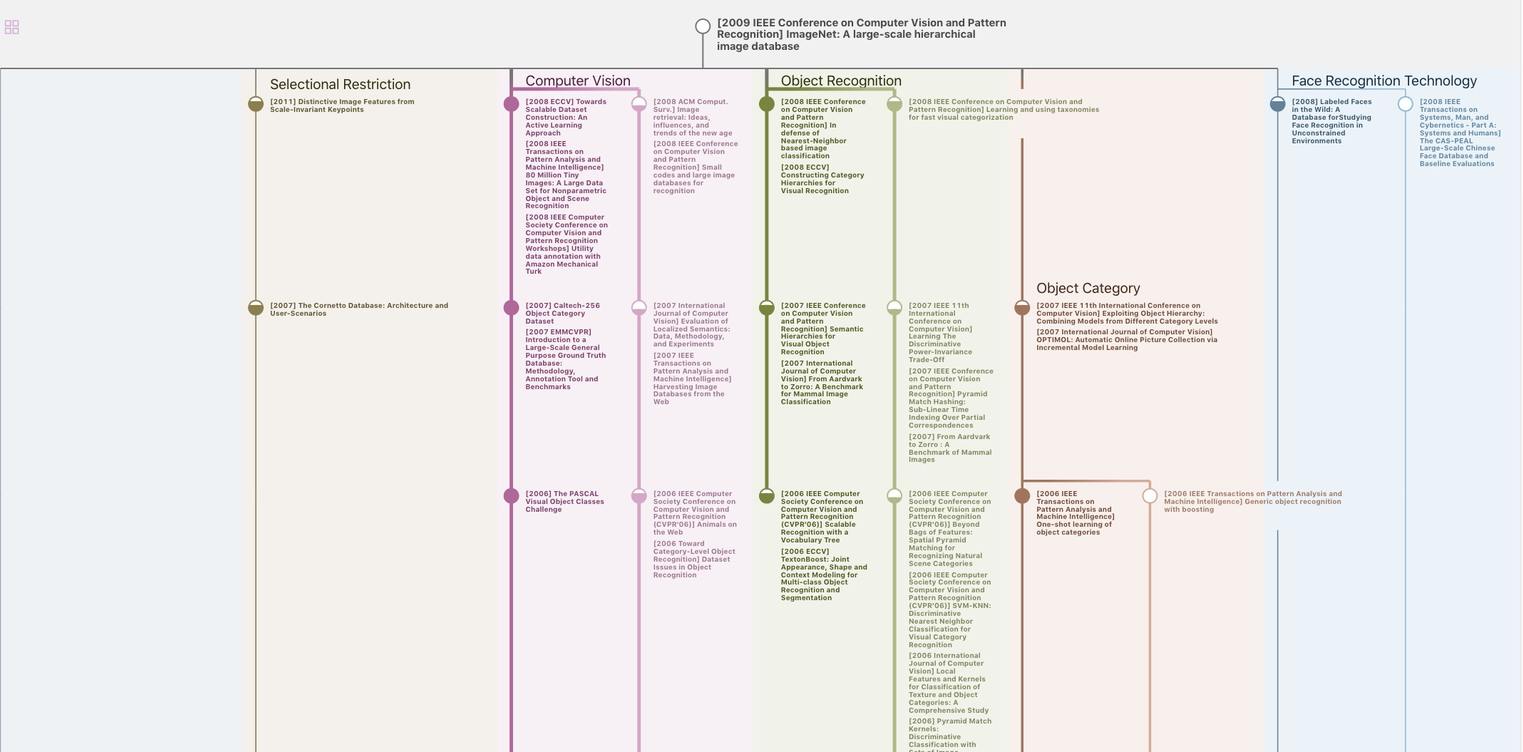
Generate MRT to find the research sequence of this paper
Chat Paper
Summary is being generated by the instructions you defined