Self-Improving-Leaderboard(SIL): A Call for Real-World Centric Natural Language Processing Leaderboards
arxiv(2023)
摘要
Leaderboard systems allow researchers to objectively evaluate Natural Language Processing (NLP) models and are typically used to identify models that exhibit superior performance on a given task in a predetermined setting. However, we argue that evaluation on a given test dataset is just one of many performance indications of the model. In this paper, we claim leaderboard competitions should also aim to identify models that exhibit the best performance in a real-world setting. We highlight three issues with current leaderboard systems: (1) the use of a single, static test set, (2) discrepancy between testing and real-world application (3) the tendency for leaderboard-centric competition to be biased towards the test set. As a solution, we propose a new paradigm of leaderboard systems that addresses these issues of current leaderboard system. Through this study, we hope to induce a paradigm shift towards more real -world-centric leaderboard competitions.
更多查看译文
关键词
natural
AI 理解论文
溯源树
样例
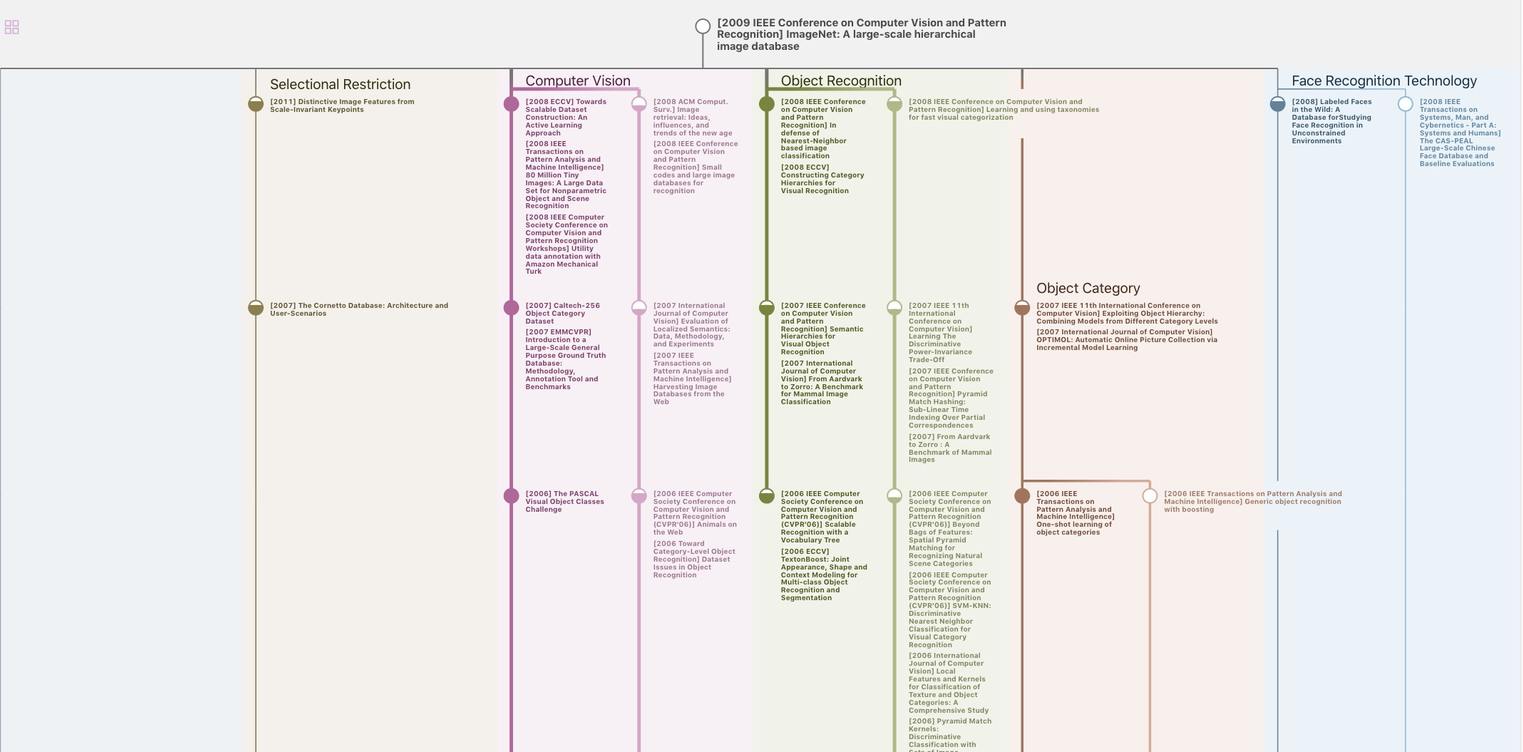
生成溯源树,研究论文发展脉络
Chat Paper
正在生成论文摘要