A Survey on Oversmoothing in Graph Neural Networks
arxiv(2023)
摘要
Node features of graph neural networks (GNNs) tend to become more similar with the increase of the network depth. This effect is known as over-smoothing, which we axiomatically define as the exponential convergence of suitable similarity measures on the node features. Our definition unifies previous approaches and gives rise to new quantitative measures of over-smoothing. Moreover, we empirically demonstrate this behavior for several over-smoothing measures on different graphs (small-, medium-, and large-scale). We also review several approaches for mitigating over-smoothing and empirically test their effectiveness on real-world graph datasets. Through illustrative examples, we demonstrate that mitigating over-smoothing is a necessary but not sufficient condition for building deep GNNs that are expressive on a wide range of graph learning tasks. Finally, we extend our definition of over-smoothing to the rapidly emerging field of continuous-time GNNs.
更多查看译文
关键词
graph,neural networks
AI 理解论文
溯源树
样例
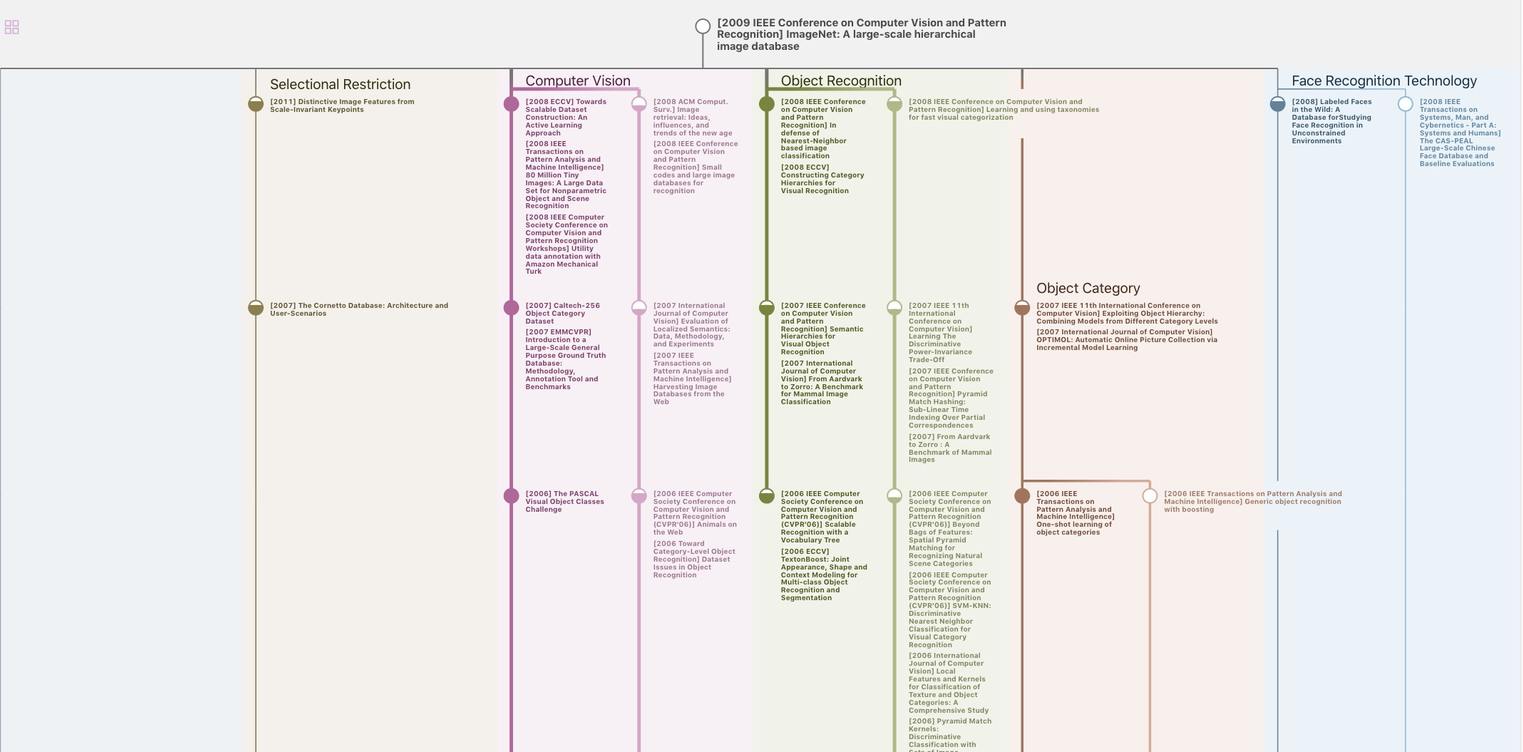
生成溯源树,研究论文发展脉络
Chat Paper
正在生成论文摘要