A Unified Framework of Policy Learning for Contextual Bandit with Confounding Bias and Missing Observations
CoRR(2023)
摘要
We study the offline contextual bandit problem, where we aim to acquire an optimal policy using observational data. However, this data usually contains two deficiencies: (i) some variables that confound actions are not observed, and (ii) missing observations exist in the collected data. Unobserved confounders lead to a confounding bias and missing observations cause bias and inefficiency problems. To overcome these challenges and learn the optimal policy from the observed dataset, we present a new algorithm called Causal-Adjusted Pessimistic (CAP) policy learning, which forms the reward function as the solution of an integral equation system, builds a confidence set, and greedily takes action with pessimism. With mild assumptions on the data, we develop an upper bound to the suboptimality of CAP for the offline contextual bandit problem.
更多查看译文
关键词
contextual bandit,policy learning,confounding bias
AI 理解论文
溯源树
样例
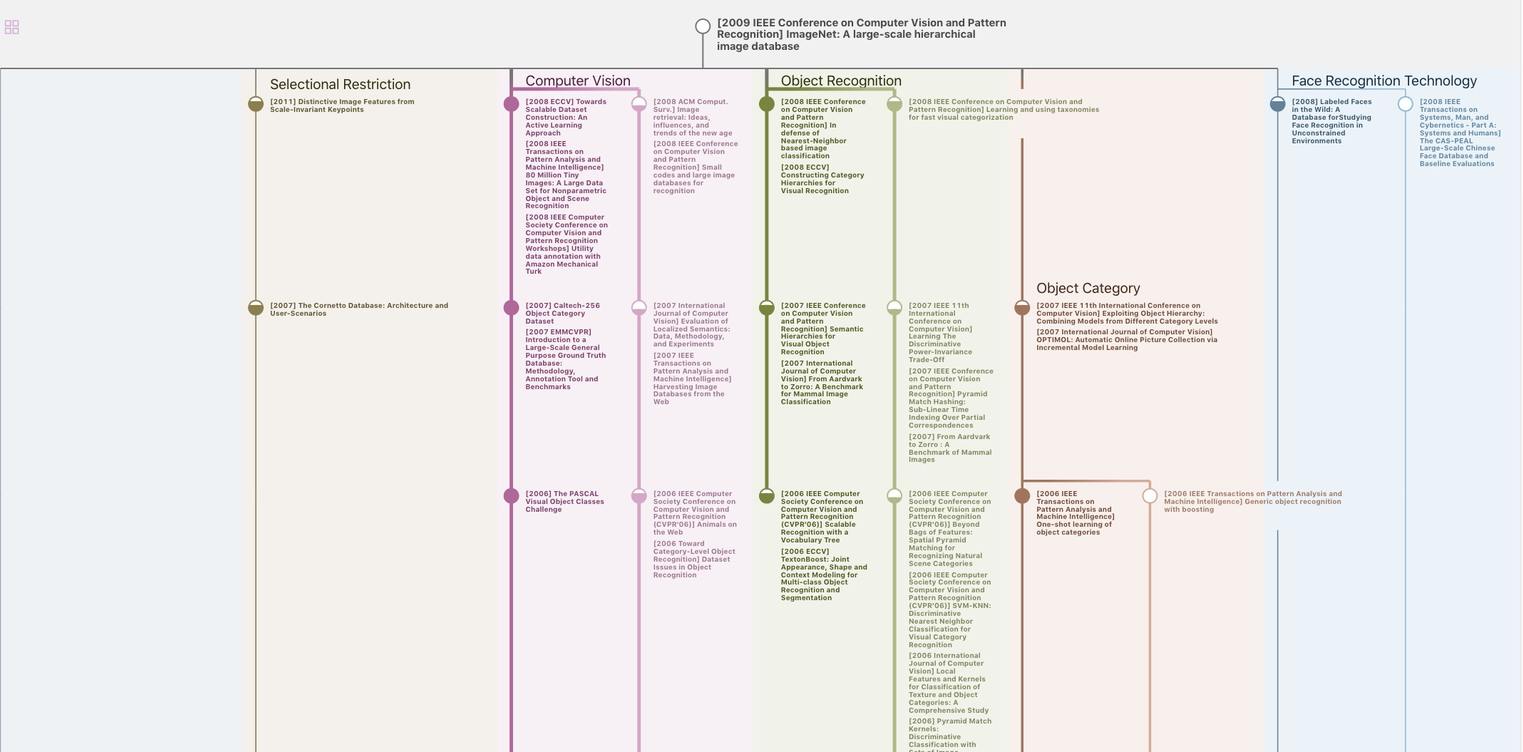
生成溯源树,研究论文发展脉络
Chat Paper
正在生成论文摘要