Analysis of UAS-LiDAR Ground Points Classification in Agricultural Fields Using Traditional Algorithms and PointCNN.
Remote. Sens.(2023)
摘要
Classifying bare earth (ground) points from Light Detection and Ranging (LiDAR) point clouds is well-established research in the forestry, topography, and urban domains using point clouds acquired by Airborne LiDAR System (ALS) at average point densities (approximate to 2 points per meter-square (pts/m(2))). The paradigm of point cloud collection has shifted with the advent of unmanned aerial systems (UAS) onboard affordable laser scanners with commercial utility (e.g., DJI Zenmuse L1 sensor) and unprecedented repeatability of UAS-LiDAR surveys. Therefore, there is an immediate need to investigate the existing methods, and to develop new ground classification methods, using UAS-LiDAR. In this paper, for the first time, traditional ground classification algorithms and modern machine learning methods were investigated to filter ground from point clouds of high-density UAS-LiDAR data (approximate to 900 pts/m(2)) over five agricultural fields in North Dakota, USA. To this end, we tested frequently used ground classification algorithms: Cloth Simulation Function (CSF), Progressive Morphological Filter (PMF), Multiscale Curvature Classification (MCC), and ArcGIS ground classification algorithms along with the PointCNN deep learning model were trained. We investigated two aspects of ground classification algorithms and PointCNN: (a) Classification accuracy of optimized ground classification algorithms (i.e., fine adjustment is user-defined parameters) and PointCNN over training site, and (b) transferability potential over four yet diverse test agricultural fields. The well-established evaluation metrics of omission error, commission error, and total error, along with kappa coefficients showed that deep learning outperforms the traditional ground classification algorithms in both aspects: (a) overall classification accuracy, and (b) transferability over diverse agricultural fields.
更多查看译文
关键词
UAS,LiDAR,point clouds,precision agriculture,PointCNN,CSF,PMF,MCC
AI 理解论文
溯源树
样例
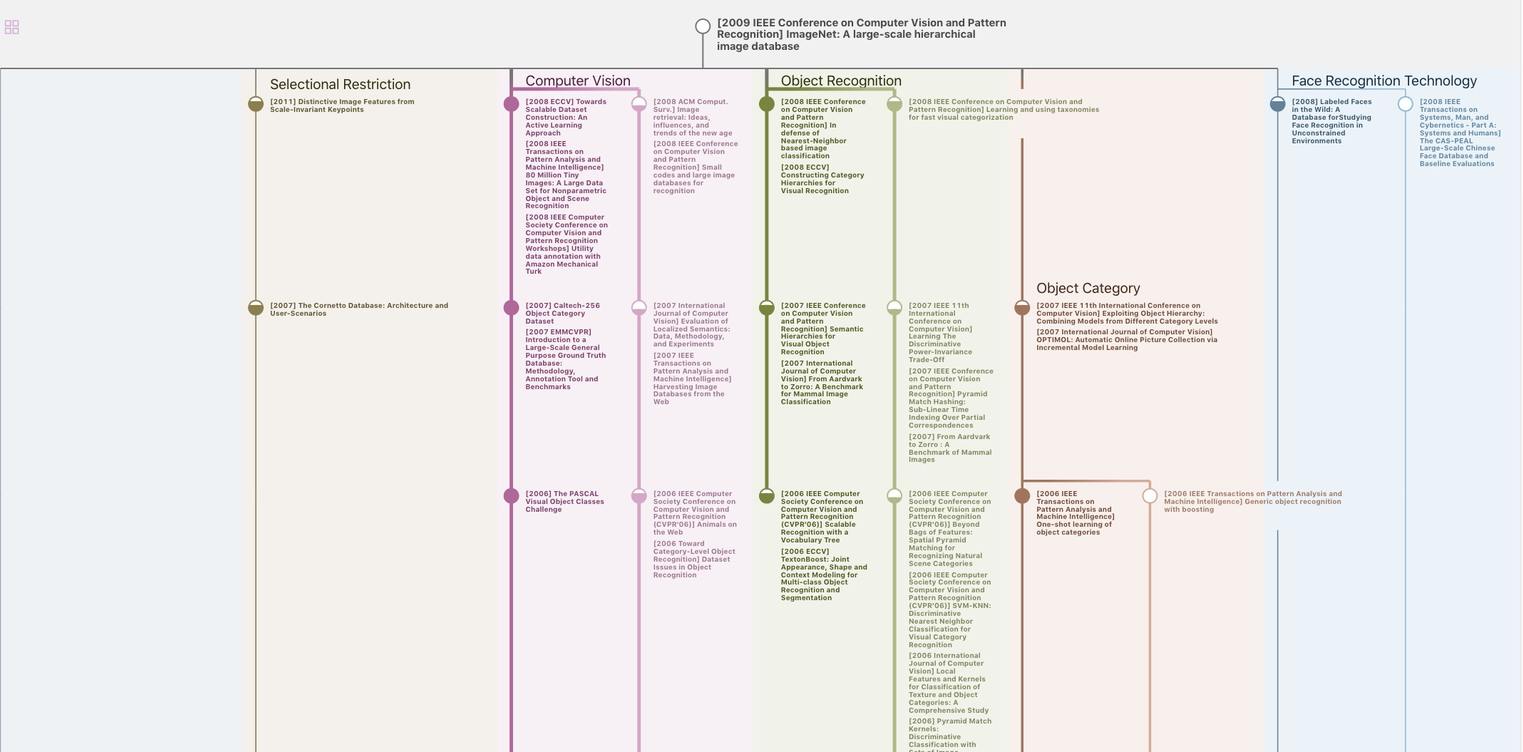
生成溯源树,研究论文发展脉络
Chat Paper
正在生成论文摘要