Monitoring Network Telescopes and Inferring Anomalous Traffic Through the Prediction of Probing Rates.
IEEE Trans. Netw. Serv. Manag.(2022)
摘要
Network reconnaissance is the first step preceding a cyber-attack. Hence, monitoring the probing activities is imperative to help security practitioners enhancing their awareness about Internet's large-scale events or peculiar events targeting their network. In this paper, we present a framework for an improved and efficient monitoring of the probing activities targeting network telescopes. Particularly, we model the probing rates which are a good indicator for measuring the cyber-security risk targeting network services. The approach consists of first inferring groups of network ports sharing similar probing characteristics through a new affinity metric capturing both temporal and semantic similarities between ports. Then, sequences of probing rates targeting similar ports are used as inputs to stacked Long Short-Term Memory (LSTM) neural networks to predict probing rates 1 hour and 1 day in advance. Finally, we describe two monitoring indicators that use the prediction models to infer anomalous probing traffic and to raise early threat warnings. We show that LSTM networks can accurately predict probing rates, outperforming the non-stationary autoregressive model, and we demonstrate that the monitoring indicators are efficient in assessing the cyber-security risk related to vulnerability disclosure.
更多查看译文
关键词
Monitoring, Predictive models, Telescopes, IP networks, Semantics, Security, Measurement, Network monitoring and measurements, network telescope, threat monitoring, security management, security situational awareness, artificial intelligence, machine learning, deep learning, unsupervised learning
AI 理解论文
溯源树
样例
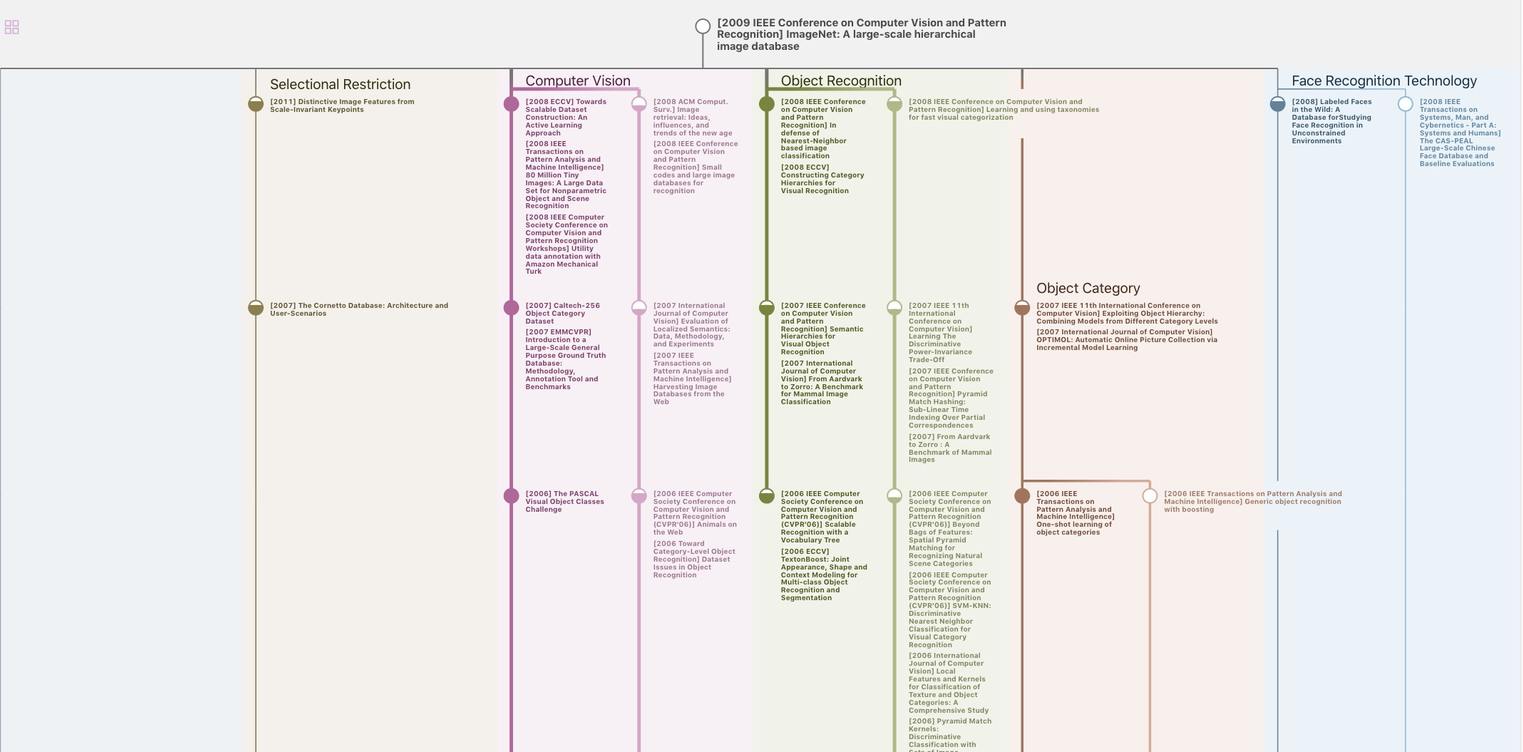
生成溯源树,研究论文发展脉络
Chat Paper
正在生成论文摘要