NRSTRNet: A Novel Network for Noise-Robust Scene Text Recognition
Int. J. Comput. Intell. Syst.(2023)
Abstract
Scene text recognition (STR) has been widely applied in industrial and commercial fields. However, existing methods still face challenges when processing text images with defects such as low contrast, blur, low resolution, and insufficient illumination. These defects are common in actual situations because of diverse text backgrounds in natural scenes and limitations in shooting conditions. To address these challenges, we propose a novel network for noise-robust scene text recognition (NRSTRNet), which comprehensively suppresses the noise in the three critical steps of STR. Specifically, in the text feature extraction stage, NRSTRNet enhances the text-related features through the channel and spatial dimensions and disregards some disturbances from the non-text area, reducing the noise and redundancy in the input image. In the context encoding stage, fine-grained feature coding is proposed to effectively reduce the influence of previous noisy temporal features on current temporal features while simultaneously reducing the impact of partial noise on the overall encoding by sharing contextual feature encoding parameters. In the decoding stage, a self-attention module is added to enhance the connections between different temporal features, thereby leveraging the global information to obtain noise-resistant features. Through these approaches, NRSTRNet can enhance the local semantic information while considering the global semantic information. Experimental results show that the proposed NRSTRNet can improve the ability to characterize text images, enhance stability under the influence of noise, and achieve superior accuracy in text recognition. As a result, our model outperforms SOTA STR models on irregular text recognition benchmarks by 2% on average, and it is exceptionally robust when applied to noisy images.
MoreTranslated text
Key words
Convolutional block attention module,Scene text recognition,Fine-grained feature,Self-attention mechanism
AI Read Science
Must-Reading Tree
Example
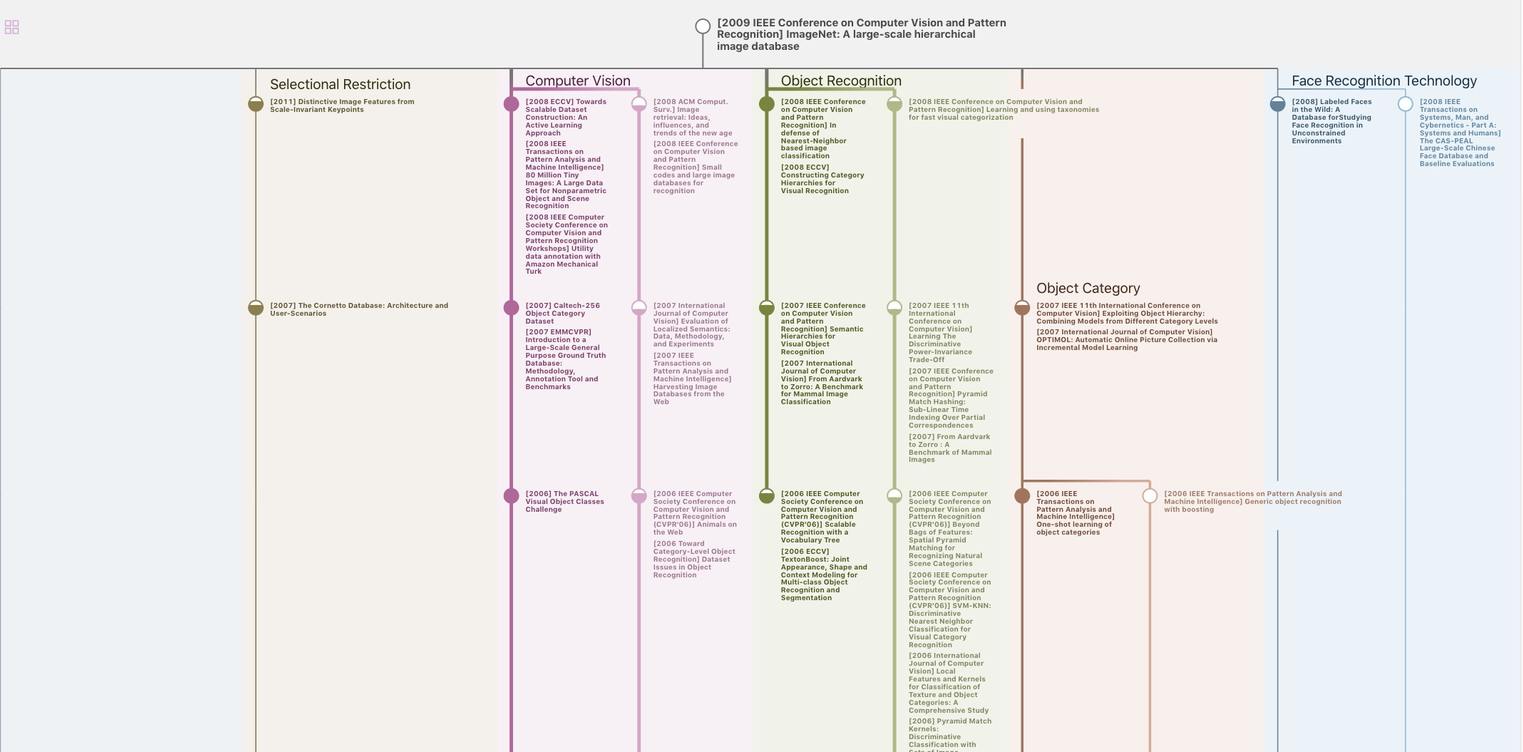
Generate MRT to find the research sequence of this paper
Chat Paper
Summary is being generated by the instructions you defined