Temporal motif-based attentional graph convolutional network for dynamic link prediction.
Intell. Data Anal.(2023)
摘要
Dynamic link prediction is an important component of the dynamic network analysis with many real-world applications. Currently, most advancements focus on analyzing link-defined neighborhoods with graph convolutional networks (GCN), while ignoring the influence of higher-order structural and temporal interacting features on link formation. Therefore, based on recent progress in modeling temporal graphs, we propose a novel temporal motif-based attentional graph convolutional network model (TMAGCN) for dynamic link prediction. As dynamic graphs usually contain periodical patterns, we first propose a temporal motif matrix construction method to capture higher-order structural and temporal features, then introduce a spatial convolution operation following a temporal motif-attention mechanism to encode these features into node embeddings. Furthermore, we design two methods to combine multiple temporal motif-based attentions, a dynamic attention-based method and a reinforcement learning-based method, to allow each individual node to make the most of the relevant motif-based neighborhood to propagate and aggregate information in the graph convolutional layers. Experimental results on various real-world datasets demonstrate that the proposed model is superior to state-of-the-art baselines on the dynamic link prediction task. It also reveals that temporal motif can manifest the essential dynamic mechanism of the network.
更多查看译文
关键词
Dynamic link prediction,graph convolutional network,temporal motif
AI 理解论文
溯源树
样例
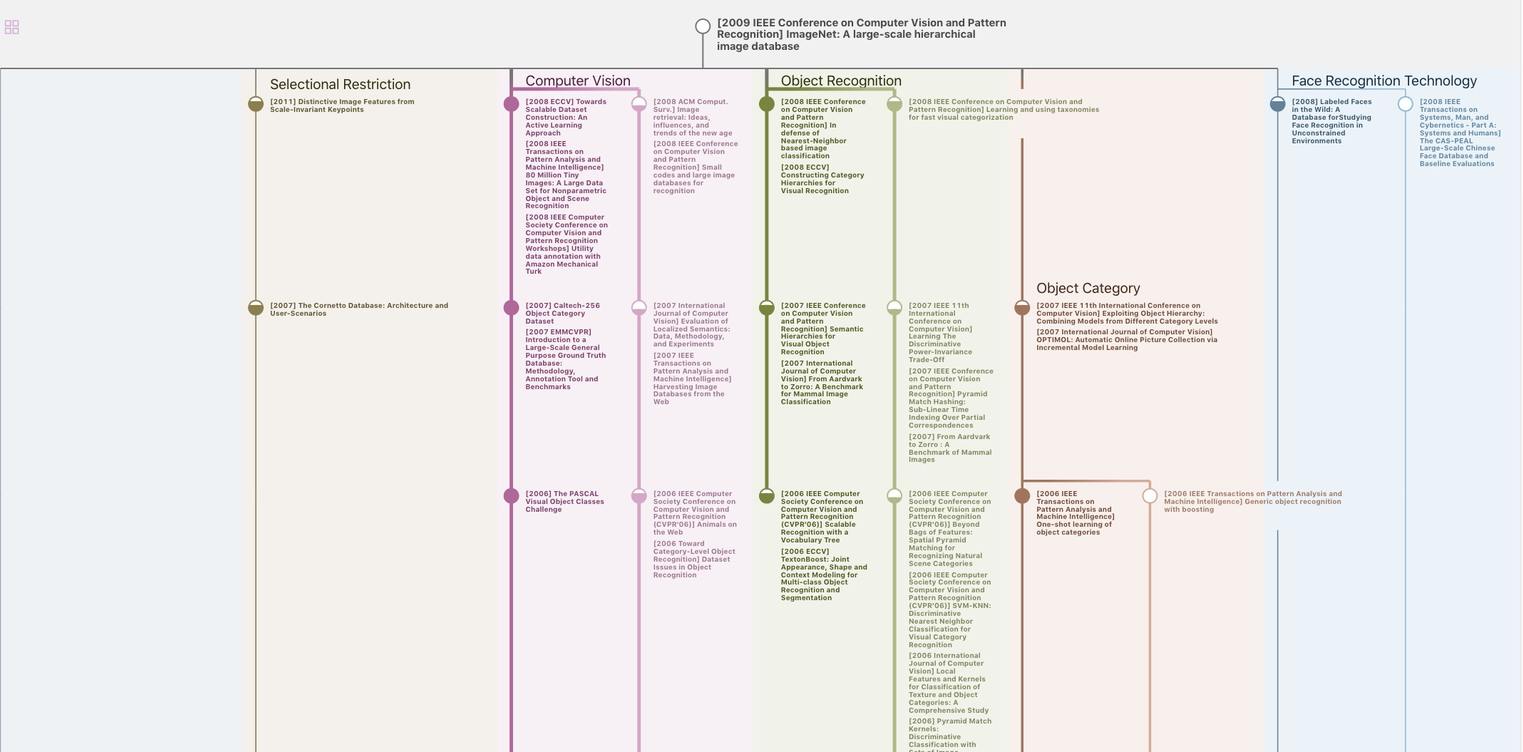
生成溯源树,研究论文发展脉络
Chat Paper
正在生成论文摘要