Time Series Classification Based on Multi-Dimensional Feature Fusion.
IEEE Access(2023)
摘要
Time series classification is a key problem in data mining, most of existing classification methods directly extract one-dimensional data from one-dimensional features, which cannot effectively express the inter-relation between different time points. Besides, some classification methods extract two-dimensional features through encoding raw one-dimensional data into two-dimensional images, and part of information is lost due to the difference of encoding methods. How to make full use of one-dimensional and two-dimensional features to extract valuable information and integrate them in an optimal fashion remains a promising challenge. In this paper, we propose a multi-scale convolutional network to extract one-dimensional features from time series for obtaining more feature information based on multi-scale convolution kernels. Two-dimensional features are constructed in terms of two-dimensional image coding based on Gramian angular field, Markov transition field and Recurrence plot (GMR) methods. We develop a multi-dimensional feature fusion approach leveraging Squeeze-and-Excitation (SE) and Self-Attention (SA) mechanism to effective fusing one-dimensional multi-scale features and two-dimensional image features in terms of weight setting. We conduct experimental verification based on 84 complete data traces from a typical UCR dataset in the field. Experimental results show that the accuracy of our proposed approach improves by 3.35% compared with existing benchmark methods. The Gradient-weighted Class Activation Mapping (Grad-CAM) visualization analysis method is adopted, where our proposed approach extracts more accurate features and effectively distinguishes different time series data categories.
更多查看译文
关键词
Feature extraction,Time series analysis,Data mining,Markov processes,Convolution,Image coding,Correlation,Time series classification,multi-scale convolution ResNet,two-dimensional image,feature fusion,accuracy
AI 理解论文
溯源树
样例
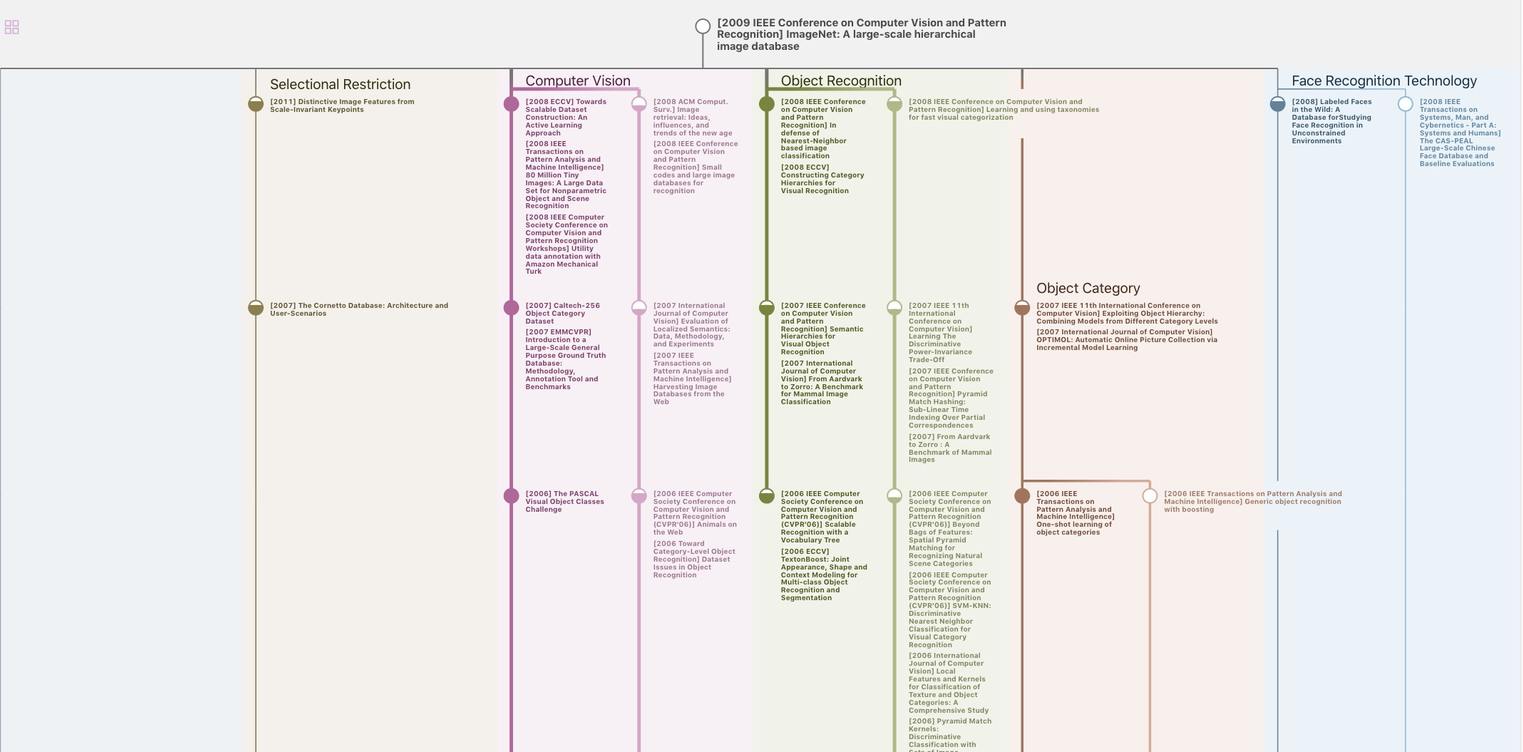
生成溯源树,研究论文发展脉络
Chat Paper
正在生成论文摘要