Pruning-Guided Curriculum Learning for Semi-Supervised Semantic Segmentation
WACV(2023)
摘要
This study focuses on improving the quality of pseudo-labeling in the context of semi-supervised semantic segmentation. Previous studies have adopted confidence thresholding to reduce erroneous predictions in pseudo-labeled data and to enhance their qualities. However, numerous pseudo-labels with high confidence scores exist in the early training stages even though their predictions are incorrect, and this ambiguity limits confidence thresholding substantially. In this paper, we present a novel method to resolve the ambiguity of confidence scores with the guidance of network pruning. A recent finding showed that network pruning severely impairs the network generalization ability on samples that are not yet well learned or represented. Inspired by this finding, we refine the confidence scores by reflecting the extent to which the predictions are affected by pruning. Furthermore, we adopted a curriculum learning strategy for the confidence score, which enables the network to learn gradually from easy to hard samples. This approach resolves the ambiguity by suppressing the learning of noisy pseudo-labels, the confidence scores of which are difficult to trust owing to insufficient training in the early stages. Extensive experiments on various benchmarks demonstrate the superiority of our framework over state-of-the-art alternatives.
更多查看译文
关键词
Algorithms: Image recognition and understanding (object detection,categorization,segmentation),Machine learning architectures,formulations,and algorithms (including transfer,low-shot,semi-,self-,and un-supervised learning)
AI 理解论文
溯源树
样例
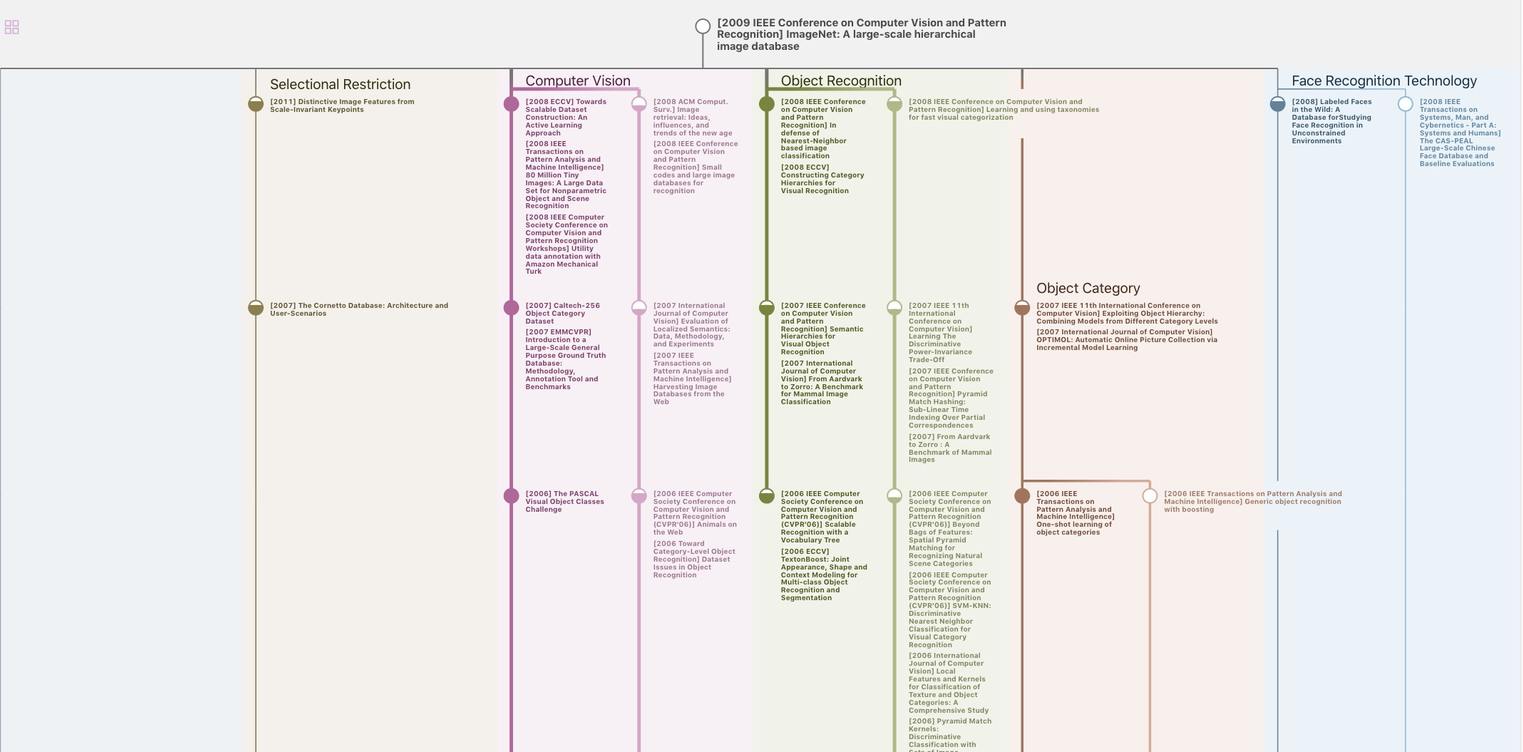
生成溯源树,研究论文发展脉络
Chat Paper
正在生成论文摘要