SSSD: Self-Supervised Self Distillation
WACV(2023)
摘要
With labeled data, self distillation (SD) has been proposed to develop compact but effective models without a complex teacher model available in advance. Such approaches need labeled data to guide the self distillation process. Inspired by self-supervised (SS) learning, we propose a self-supervised self distillation (SSSD) approach in this work. Based on an unlabeled image dataset, a model is constructed to learn visual representations in a self-supervised manner. This pre-trained model is then adopted to extract visual representations of the target dataset and generates pseudo labels via clustering. The pseudo labels guide the SD process, and thus enable SD to proceed in an unsupervised way (no data labels are required at all). We verify this idea based on evaluations on the CIFAR-10, CIFAR-100, and ImageNet-1K datasets, and demonstrate the effectiveness of this unsupervised SD approach. Performance out-performing similar frameworks is also shown.
更多查看译文
关键词
Algorithms: Machine learning architectures,formulations,and algorithms (including transfer),Image recognition and understanding (object detection,categorization,segmentation,scene modeling,visual reasoning)
AI 理解论文
溯源树
样例
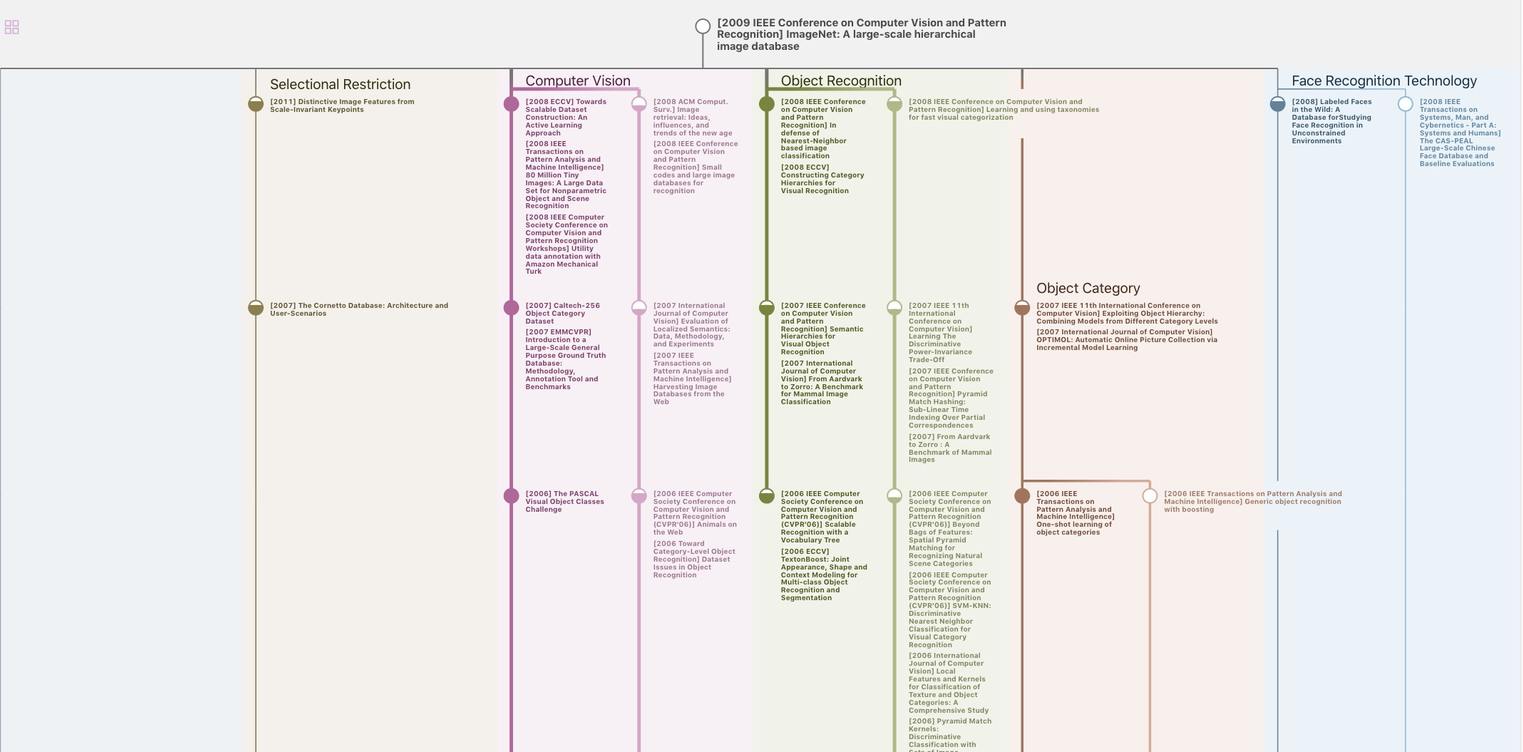
生成溯源树,研究论文发展脉络
Chat Paper
正在生成论文摘要