Intentionally seeding pores in additively manufactured alloy 718: Process parameters, microstructure, defects, and fatigue
Additive Manufacturing(2023)
摘要
Progress towards widespread adoption of additively manufactured (AM) components requires a strong understanding of the influence of pervasive pore defects on the material’s fatigue life. In this work, we describe a robust methodology to determine process parameters to intentionally seed three distinct types of pore defect structures (i.e., lack of fusion, keyhole/trapped gas, and planar/linearly aligned defects) in AM builds, alongside control specimens with minimal porosity, through a design of experiments (DOE) study for alloy 718 (also known as IN718). Normalized enthalpy and melt pool overlap were considered to adequately populate the DOE space by varying laser power, scan speed, and hatch spacing, and porosity as a function of laser energy density was compared to the literature. Builds were characterized using optical microscopy, electron backscatter diffraction (EBSD), two computed tomography (CT) techniques with an order of magnitude difference in resolution, and strain-controlled low cycle fatigue (LCF) experiments. EBSD and cyclic straining revealed that the process parameters identified to seed different pore defects did not significantly affect the microstructure. LCF tests revealed a fatigue life delineation between baseline (i.e., minimal porosity) and seeded defect samples as described above. Common porosity metrics such as volume fraction and maximum defect size did not strongly correlate to fatigue resistance but did reveal the need to closely consider the CT (or other non-destructive evaluation technique) resolution to adequately characterize pore defects. The methodology established in this work will be vital in future modeling efforts to assess potential debits to fatigue resistance in the presence of pore defects.
更多查看译文
关键词
Alloy 718,Process parameters,Microstructure,Seeded pore defects,Computed tomography,Low cycle fatigue
AI 理解论文
溯源树
样例
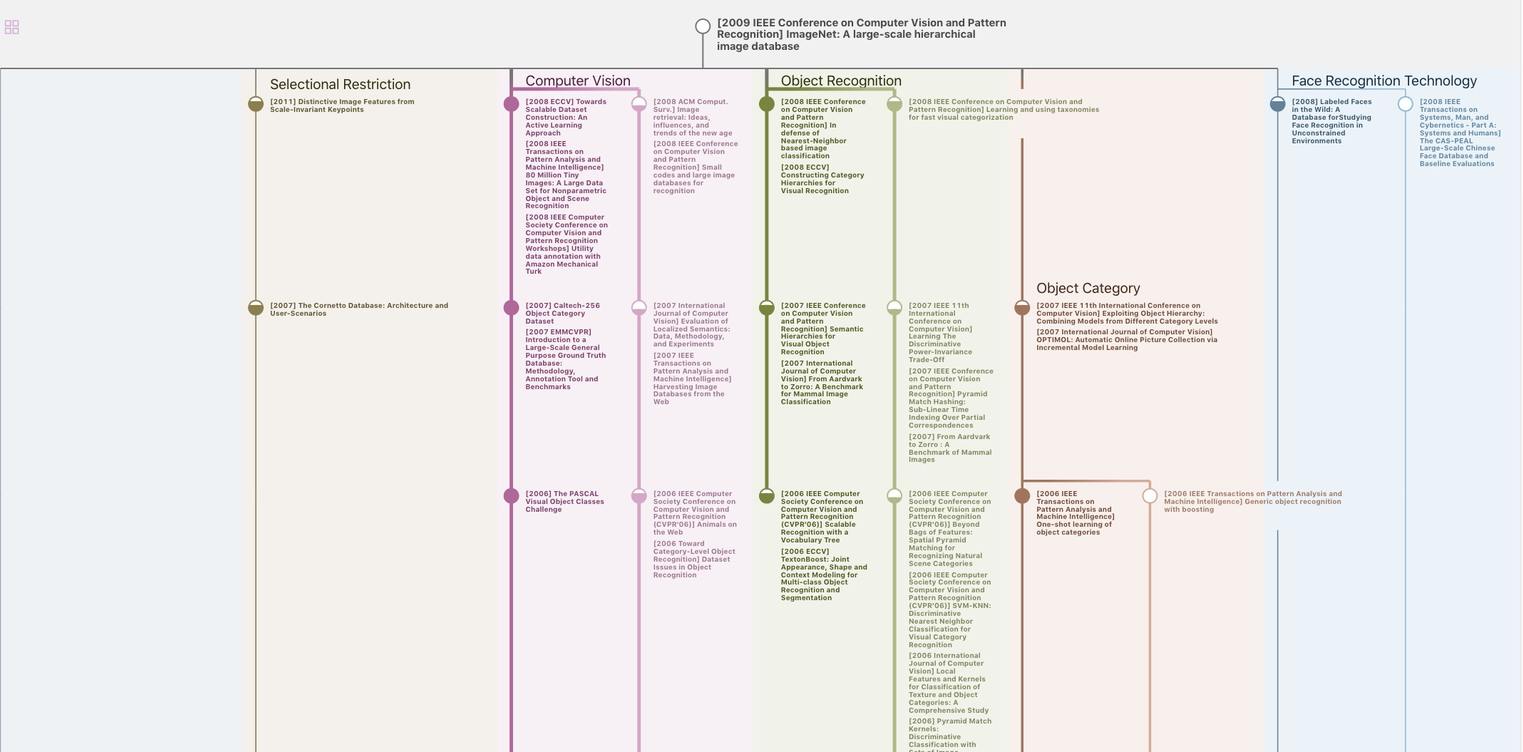
生成溯源树,研究论文发展脉络
Chat Paper
正在生成论文摘要