Using machine learning to identify early predictors of adolescent emotion regulation development.
Journal of research on adolescence : the official journal of the Society for Research on Adolescence(2023)
摘要
As 20% of adolescents develop emotion regulation difficulties, it is important to identify important early predictors thereof. Using the machine learning algorithm SEM-forests, we ranked the importance of (87) candidate variables assessed at age 13 in predicting quadratic latent trajectory models of emotion regulation development from age 14 to 18. Participants were 497 Dutch families. Results indicated that the most important predictors were individual differences (e.g., in personality), aspects of relationship quality and conflict behaviors with parents and peers, and internalizing and externalizing problems. Relatively less important were demographics, bullying, delinquency, substance use, and specific parenting practices-although negative parenting practices ranked higher than positive ones. We discuss implications for theory and interventions, and present an open source risk assessment tool, ERRATA.
更多查看译文
关键词
adolescence,emotion regulation,machine learning,random forests,theory formation
AI 理解论文
溯源树
样例
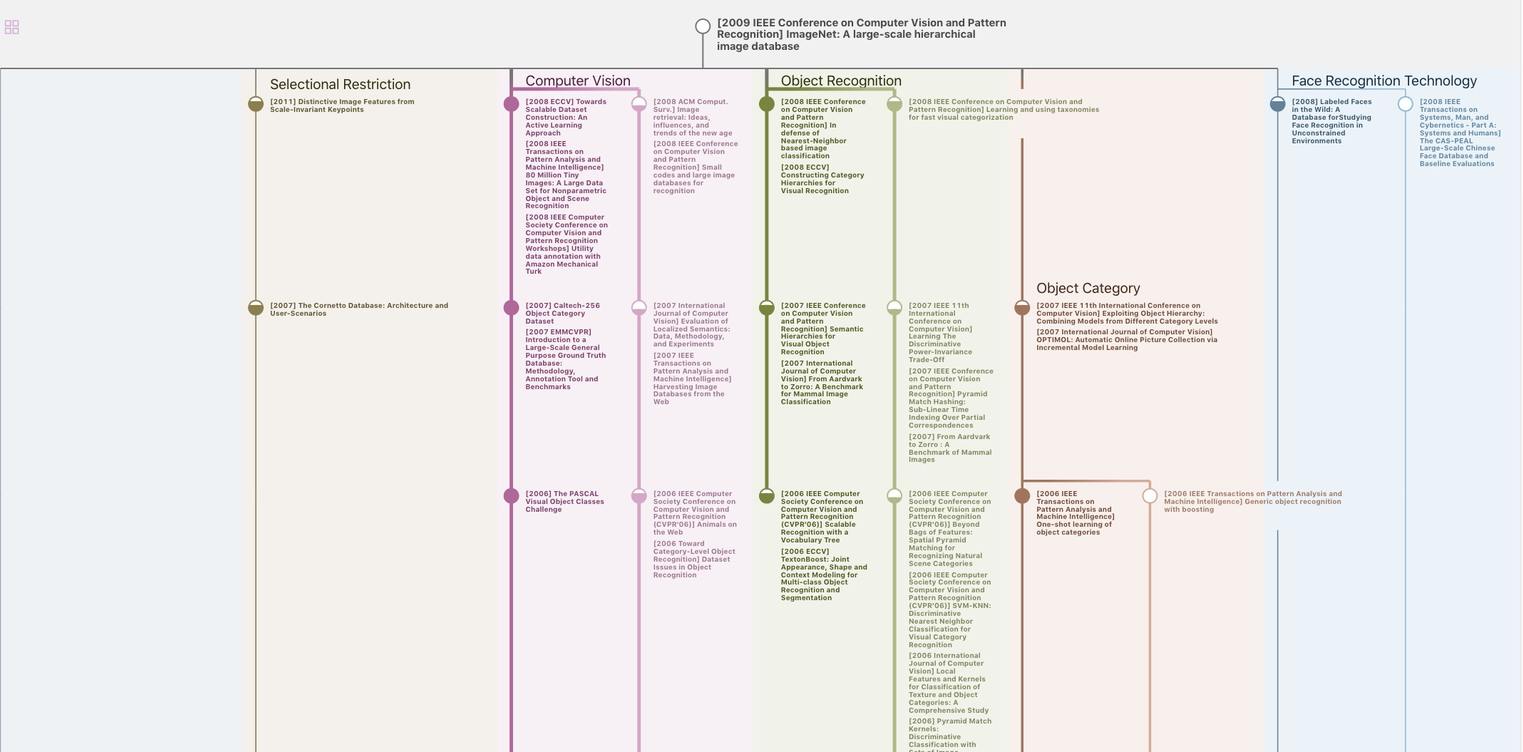
生成溯源树,研究论文发展脉络
Chat Paper
正在生成论文摘要