Quantization Robust Pruning With Knowledge Distillation
IEEE Access(2023)
摘要
To resolve the problem that deep neural networks (DNN) require a large number of network parameters, many researchers have sought to compress the network. Network pruning, quantization and knowledge distillation have been studied for this purpose. Considering realistic scenarios such as deploying DNN on the resource constraint device where the network uploaded in the device performs wells in various bit-widths without re-training and the network with reasonable performance, we propose quantization robust pruning with knowledge distillation (QRPK) method. In QRPK, model weights are divided into essential weigths and inessential weights based on their magnitude value. Then, QRPK trains the quantization robustness model with a high pruning ratio by making the distribution of essential weights as a quantization friendly distribution. We conducted experiments on CIFAR-10 and CIFAR-100 to verify the effectiveness of QRPK and a QRPK trained model performs well in various bit-width, as it designed by pruning, quantization robustness and knowledge distillation.
更多查看译文
关键词
Convolutional neural networks,network quantization,knowledge distillation,network pruning
AI 理解论文
溯源树
样例
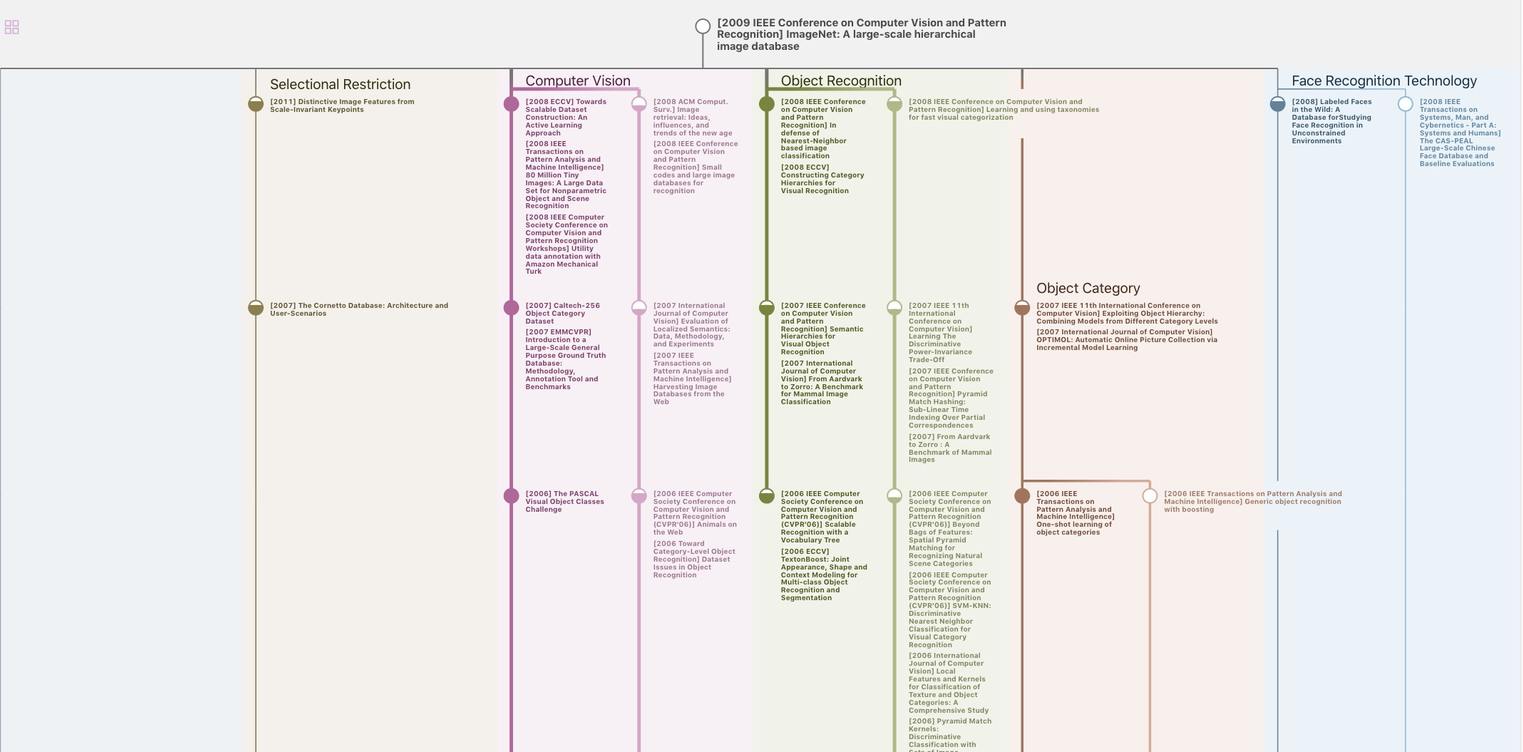
生成溯源树,研究论文发展脉络
Chat Paper
正在生成论文摘要