NASDM: Nuclei-Aware Semantic Histopathology Image Generation Using Diffusion Models
MEDICAL IMAGE COMPUTING AND COMPUTER ASSISTED INTERVENTION, MICCAI 2023, PT VI(2023)
摘要
In recent years, computational pathology has seen tremendous progress driven by deep learning methods in segmentation and classification tasks aiding prognostic and diagnostic settings. Nuclei segmentation, for instance, is an important task for diagnosing different cancers. However, training deep learning models for nuclei segmentation requires large amounts of annotated data, which is expensive to collect and label. This necessitates explorations into generative modeling of histopathological images. In this work, we use recent advances in conditional diffusion modeling to formulate a first-of-its-kind nuclei-aware semantic tissue generation framework (NASDM) which can synthesize realistic tissue samples given a semantic instance mask of up to six different nuclei types, enabling pixel-perfect nuclei localization in generated samples. These synthetic images are useful in applications in pathology pedagogy, validation of models, and supplementation of existing nuclei segmentation datasets. We demonstrate that NASDM is able to synthesize high-quality histopathology images of the colon with superior quality and semantic controllability over existing generative methods. Implementation: https://github.com/4m4n5/NASDM.
更多查看译文
关键词
Generative Modeling,Histopathology,Diffusion Models
AI 理解论文
溯源树
样例
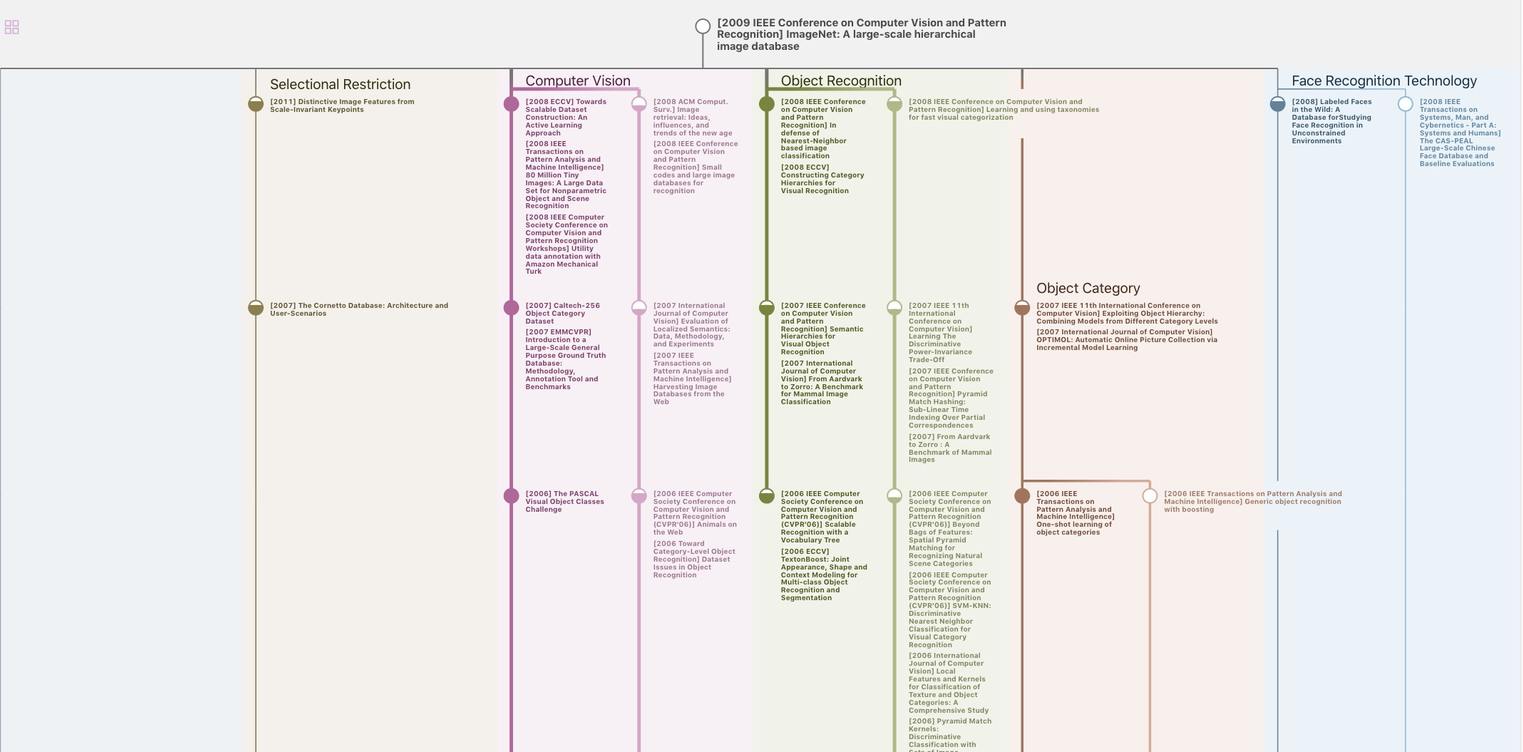
生成溯源树,研究论文发展脉络
Chat Paper
正在生成论文摘要