HRDFuse: Monocular 360 Depth Estimation by Collaboratively Learning Holistic-with-Regional Depth Distributions
arxiv(2023)
摘要
Depth estimation from a monocular 360 degrees image is a burgeoning problem owing to its holistic sensing of a scene. Recently, some methods, e.g., OmniFusion, have applied the tangent projection (TP) to represent a 360 degrees image and predicted depth values via patch-wise regressions, which are merged to get a depth map with equirectangular projection (ERP) format. However, these methods suffer from 1) non-trivial process of merging plenty of patches; 2) capturing less holistic-with-regional contextual information by directly regressing the depth value of each pixel. In this paper, we propose a novel framework, HRDFuse, that subtly combines the potential of convolutional neural networks (CNNs) and transformers by collaboratively learning the holistic contextual information from the ERP and the regional structural information from the TP. Firstly, we propose a spatial feature alignment (SFA) module that learns feature similarities between the TP and ERP to aggregate the TP features into a complete ERP feature map in a pixel-wise manner. Secondly, we propose a collaborative depth distribution classification (CDDC) module that learns the holistic-with-regional histograms capturing the ERP and TP depth distributions. As such, the final depth values can be predicted as a linear combination of histogram bin centers. Lastly, we adaptively combine the depth predictions from ERP and TP to obtain the final depth map. Extensive experiments show that our method predicts more smooth and accurate depth results while achieving favorably better results than the SOTA methods.
更多查看译文
关键词
depth estimation,learning,holistic-with-regional
AI 理解论文
溯源树
样例
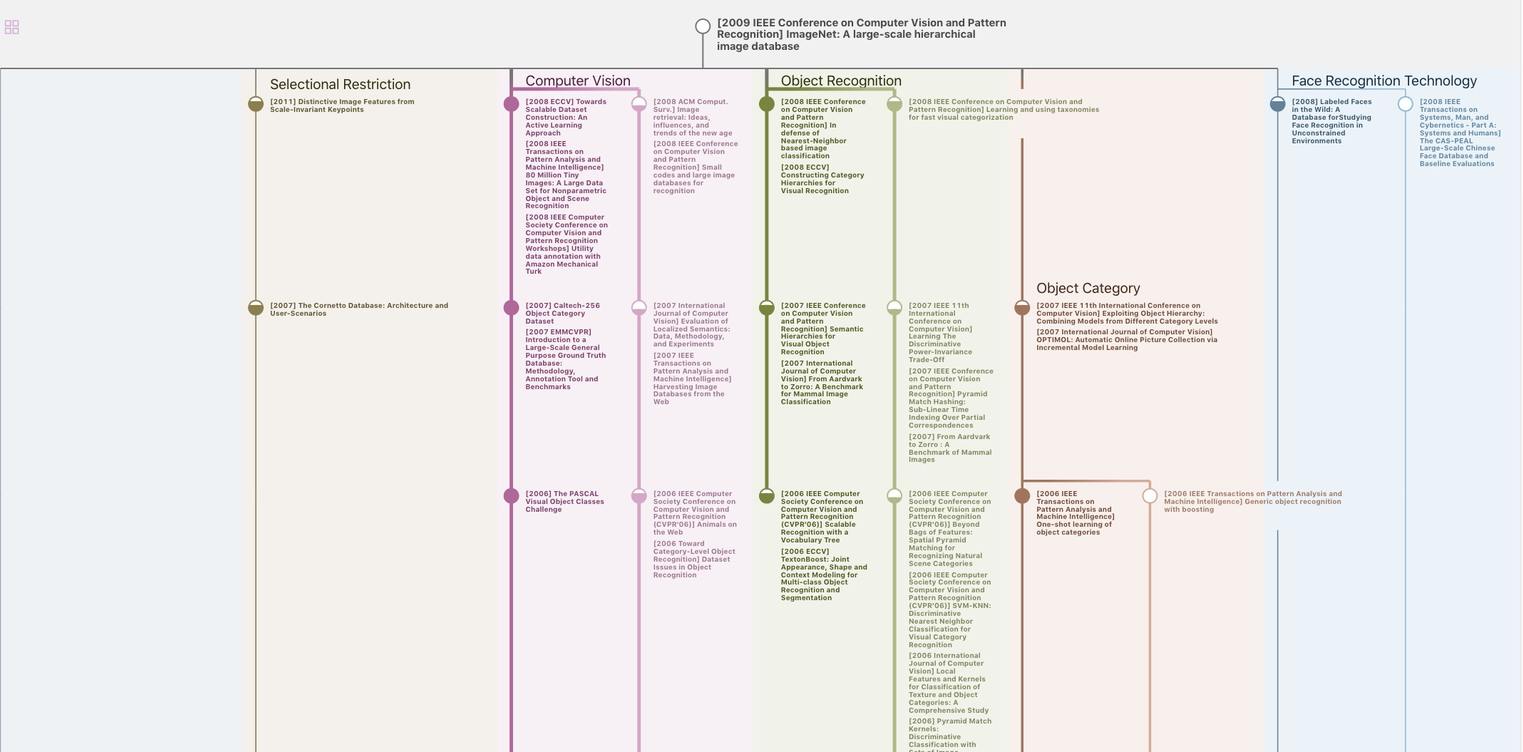
生成溯源树,研究论文发展脉络
Chat Paper
正在生成论文摘要