Self-Sufficient Framework for Continuous Sign Language Recognition
arxiv(2023)
摘要
The goal of this work is to develop self-sufficient framework for Continuous Sign Language Recognition (CSLR) that addresses key issues of sign language recognition. These include the need for complex multi-scale features such as hands, face, and mouth for understanding, and absence of frame-level annotations. To this end, we propose (1) Divide and Focus Convolution (DFConv) which extracts both manual and non-manual features without the need for additional networks or annotations, and (2) Dense Pseudo-Label Refinement (DPLR) which propagates non-spiky frame-level pseudo-labels by combining the ground truth gloss sequence labels with the predicted sequence. We demonstrate that our model achieves state-of-the-art performance among RGB-based methods on large-scale CSLR benchmarks, PHOENIX-2014 and PHOENIX-2014-T, while showing comparable results with better efficiency when compared to other approaches that use multi-modality or extra annotations.
更多查看译文
关键词
complex multiscale features,Continuous Sign Language Recognition,CSLR,dense pseudolabel refinement,DFConv,divide and focus convolution,DPLR,frame-level annotations,ground truth gloss sequence labels,large-scale CSLR benchmarks,manual feature extraction,nonmanual feature extraction,nonspiky frame-level pseudolabels,PHOENIX-2014,PHOENIX-2014-T,RGB-based methods,self-sufficient framework,sign language recognition
AI 理解论文
溯源树
样例
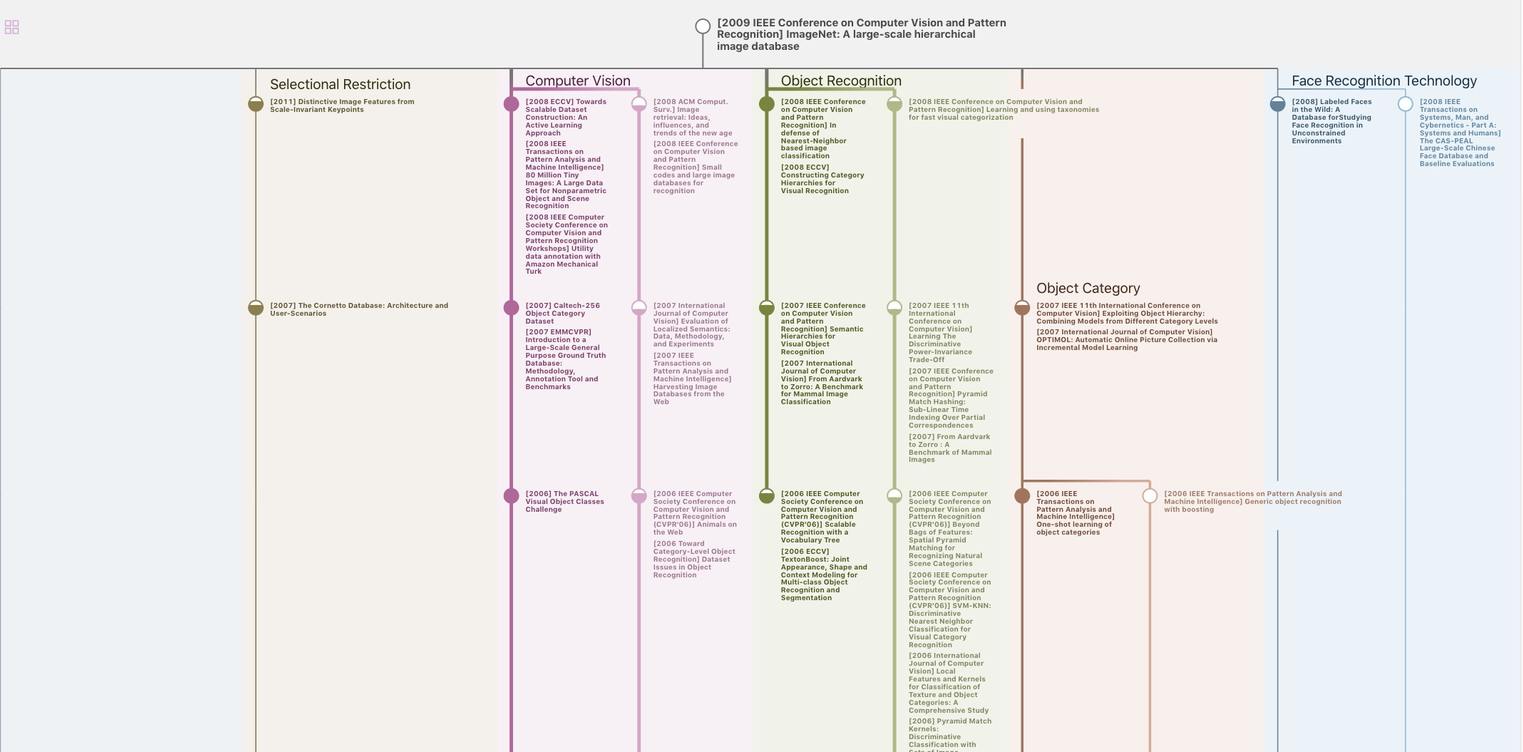
生成溯源树,研究论文发展脉络
Chat Paper
正在生成论文摘要