An improved autoencoder for recommendation to alleviate the vanishing gradient problem
Knowledge-Based Systems(2023)
摘要
In the recommendation domain, user rating data has high sparsity and the number of interaction information from each user is very uneven, which brings great technical challenge to designing high-quality personalized recommendation schemes. Although autoencoder-based recommendation models have achieved some success in handling sparse data, they do not deal well with the uneven distribution of the amount of interaction information among different users, which further prevents the improvement of recommendation performance. In this paper, we find that the vanishing gradient problem occurs in the process of training model with the data from active users and theoretically analyze its root cause. Then, an improved autoencoder is proposed to alleviate this problem in recommendation domain. Furthermore, based on the improved autoencoder, two recommendation schemes are presented for rating prediction task and top-N ranking task, respectively. Finally, the numerical experimental results demonstrate that the proposed recommendation schemes can achieve about 5% and 3% improvements in the rating prediction and top-N ranking, respectively. Therefore, the improved model can well cope with the challenges brought by data sparsity and uneven distribution of rating data and achieve good recommendation performance.
更多查看译文
关键词
Recommendation system,Autoencoder,Collaborative filtering,Vanishing gradient problem
AI 理解论文
溯源树
样例
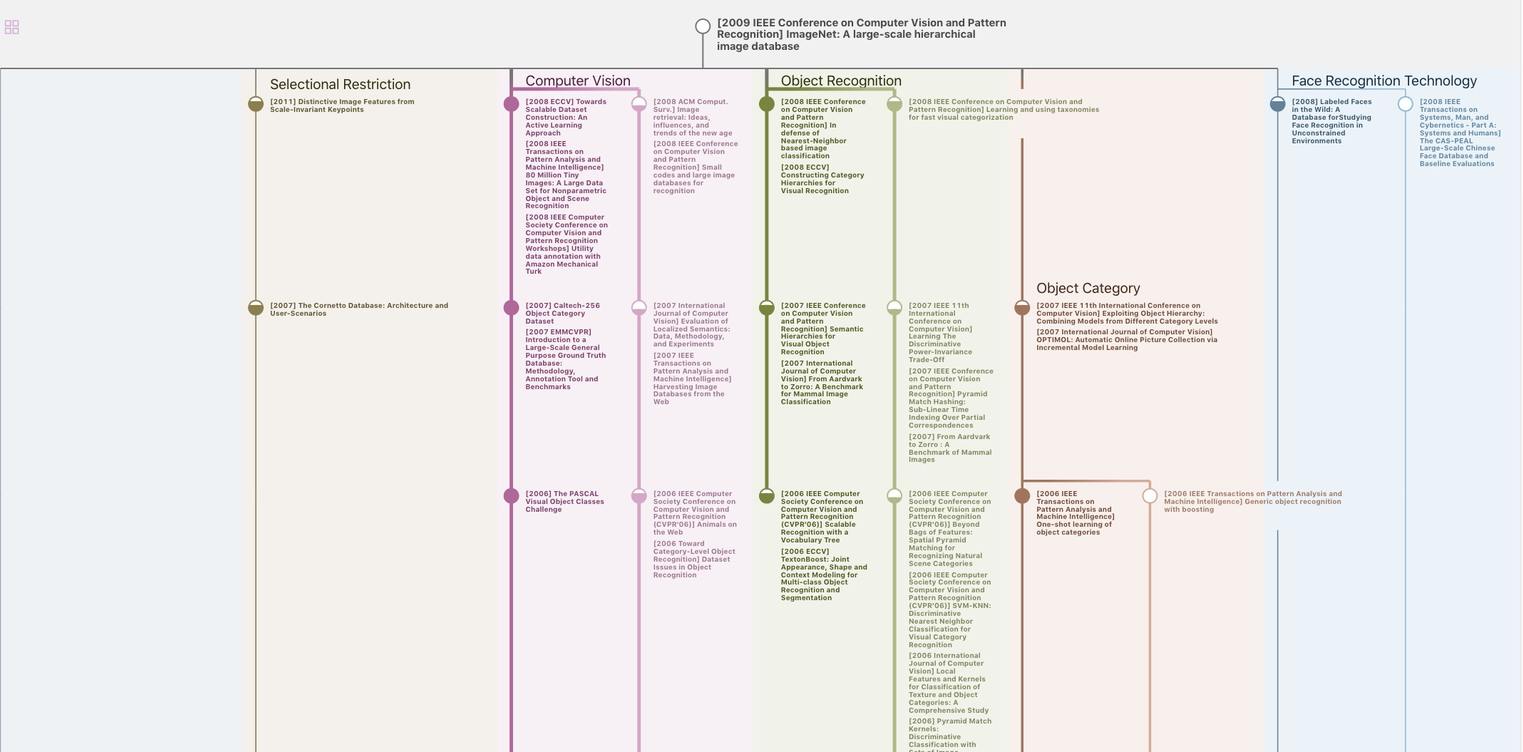
生成溯源树,研究论文发展脉络
Chat Paper
正在生成论文摘要