Multi-perspective enhanced representation for effective session-based recommendation
Knowledge-Based Systems(2023)
摘要
Session-based recommendation uses the click history of anonymous users to recommend the next possible click item for a given point in time. GNN-based models have returned promising results. However, these models still have some limitations. First, when GNN encodes the session data into session graphs, the order of the items is ignored; second, the GNN layer limit makes it impossible to capture some long-term dependencies in the session; third, noise items in the final generated session embedding will interfere with the model’s predictions. We propose a new multiperspective enhanced session representation recommendation model (MPER) to address the above problems. First, we introduce learnable position embedding to record the sequential relationships of the items, and propose the SLD-encoder to capture richer global information. Second, we propose an adaptive sparse target attention mechanism to alleviate the effect of the noise items in the traditional target embeddings. Third, a linear integration layer is added to the model to ensure that the session and item embeddings are in the same representation space. We conduct experiments on three real datasets, and the experimental results show that our model outperforms the SOTA baseline studies.
更多查看译文
关键词
Session-based recommendation,Transformer,Graph neural networks
AI 理解论文
溯源树
样例
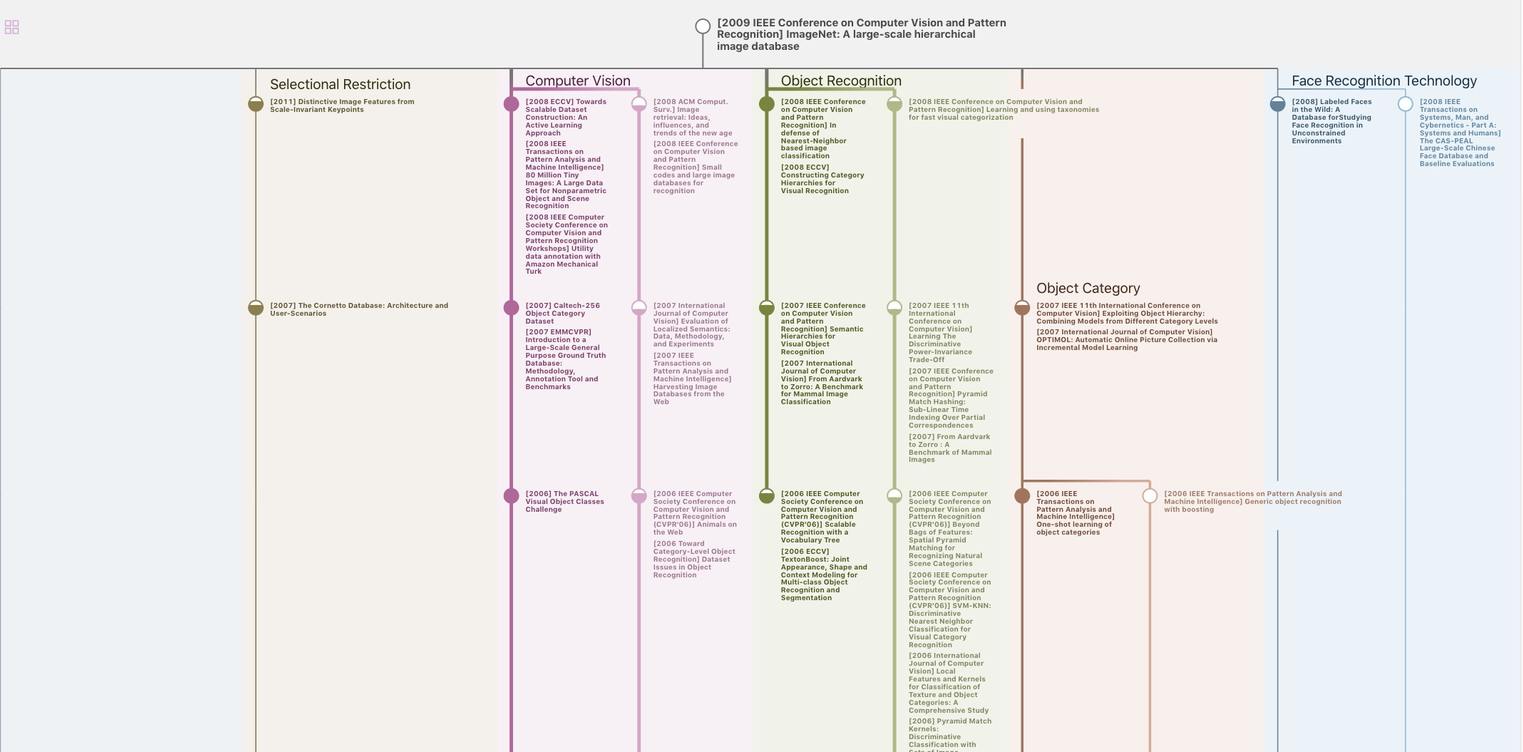
生成溯源树,研究论文发展脉络
Chat Paper
正在生成论文摘要