Multi-feature space similarity supplement for few-shot class incremental learning
Knowledge-Based Systems(2023)
摘要
With the continuous addition of new classes of data, incremental learning is widely followed and studied, especially for few-shot scenarios. Few-shot class incremental learning (FSCIL) aims to continually learn new knowledge with limited data, without forgetting old knowledge. Owing to limited new class samples and special incremental processes, FSCIL faces the problems of old knowledge catastrophic forgetting and new class adaption. We focus on a multi-feature space similarity supplement (MFS3) to alleviate the two dilemmas. We first train different feature spaces for different sessions, and use an inter-feature space similarity supplement (IFS3) to focus on boundary-sensitive sample points to improve the expression ability of every single session. To handle old knowledge catastrophic forgetting, we use the base feature space as the major and take multiple new feature spaces as supplements. For better adaption of new classes, we further design an outer-feature space similarity supplement (OFS3). OFS3 can utilize the supplement of base feature space with new feature space to rejudge the sample points, whose similarity results of base feature space are changed due to the addition of new classes. In general, IFS3 facilitates a single session, while OFS3 contributes a multi-feature space similarity supplement between different sessions in FSCIL. Sufficient experiments on three benchmark datasets, including CIFAR100, miniImageNet, and CUB200 demonstrate the advantage of our method. With the multi-feature space similarity supplement strategy, our method outperforms the state-of-the-art approaches by a large margin.
更多查看译文
关键词
Few-shot class incremental learning,Incremental learning,Prototype learning
AI 理解论文
溯源树
样例
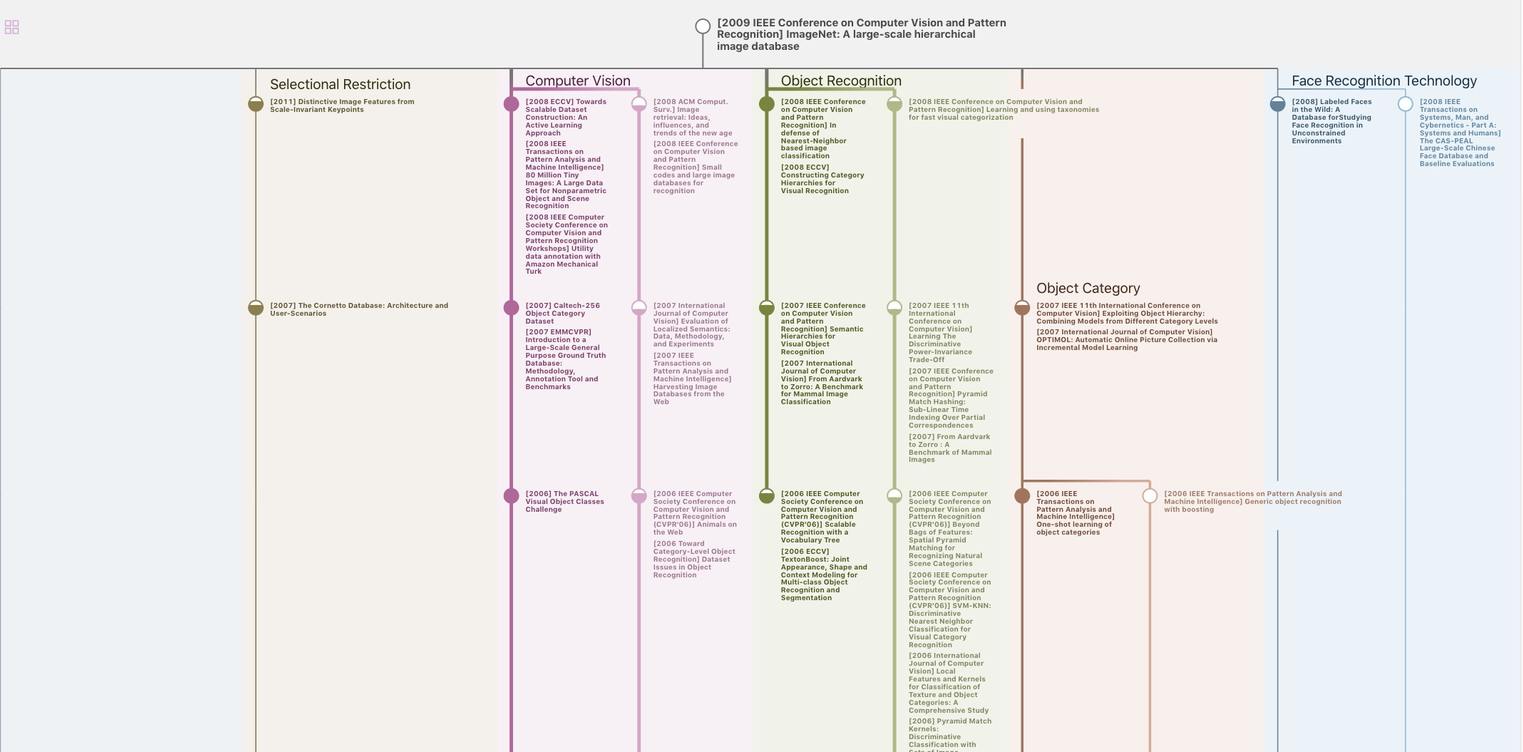
生成溯源树,研究论文发展脉络
Chat Paper
正在生成论文摘要