LiDARFormer: A Unified Transformer-Based Multi-Task Network for LiDAR Perception
ICRA 2024(2024)
摘要
There is a recent need in the LiDAR perception field for unifying multiple tasks in a single strong network with improved performance, as opposed to using separate networks for each task. In this paper, we introduce a new LiDAR multi-task learning paradigm based on the transformer. The proposed LiDARFormer utilizes cross-space global contextual feature information and exploits cross-task synergy to boost the performance of LiDAR perception tasks across multiple large-scale datasets and benchmarks. Our novel transformer-based framework includes a cross-space transformer module that learns attentive features between the 2D dense Bird's Eye View (BEV) and 3D sparse voxel feature maps. Additionally, we propose a transformer decoder for the segmentation task to dynamically adjust the learned features by leveraging the categorical feature representations. Furthermore, we combine the segmentation and detection features in a shared transformer decoder with cross-task attention layers to enhance and integrate the object-level and class-level features. LiDARFormer is evaluated on the large-scale nuScenes and the Waymo Open datasets for both 3D detection and semantic segmentation tasks, and it achieves state-of-the-art performance on both tasks.
更多查看译文
关键词
Deep Learning for Visual Perception,Object Detection, Segmentation and Categorization,Computer Vision for Transportation
AI 理解论文
溯源树
样例
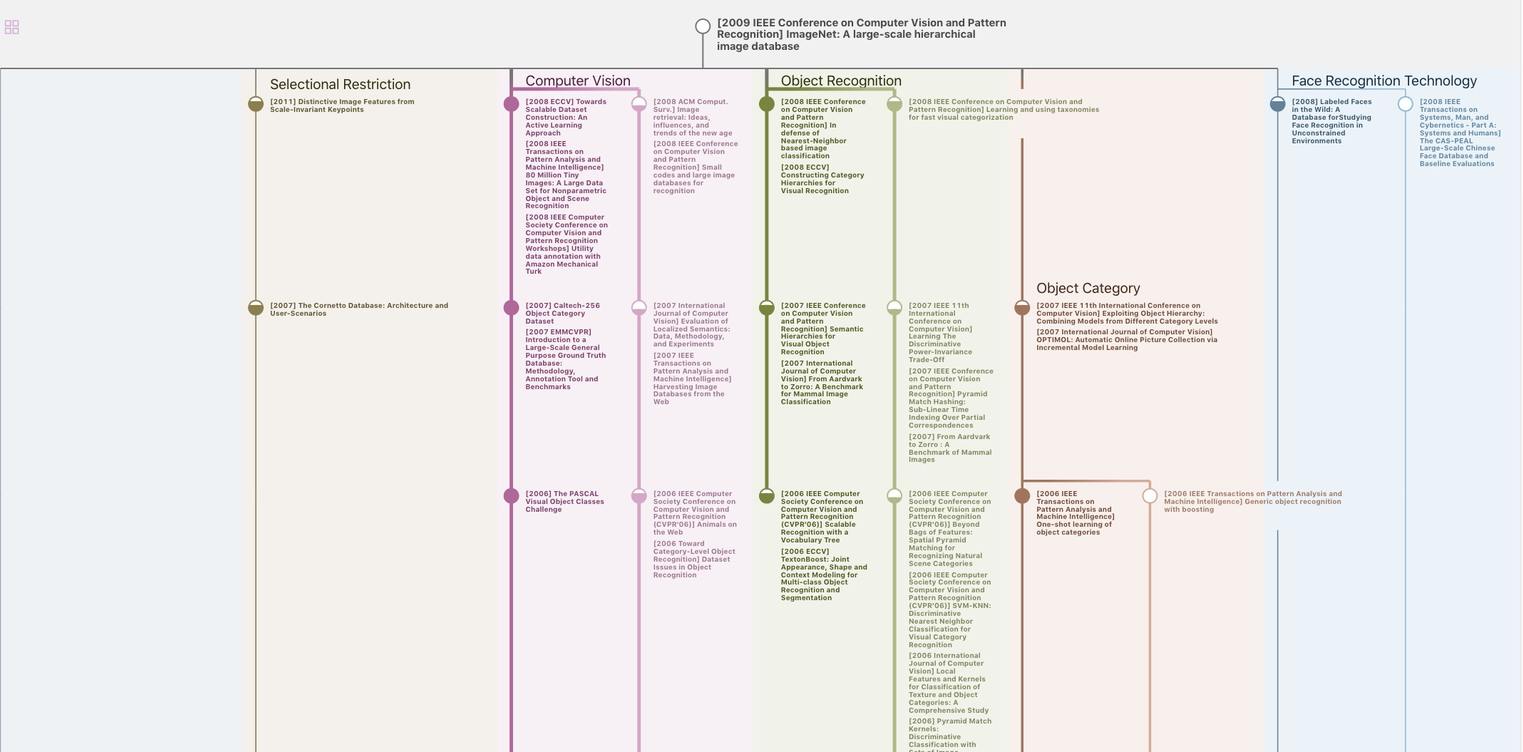
生成溯源树,研究论文发展脉络
Chat Paper
正在生成论文摘要