Deep Reinforcement Learning for Localizability-Enhanced Navigation in Dynamic Human Environments
arxiv(2023)
摘要
Reliable localization is crucial for autonomous robots to navigate efficiently and safely. Some navigation methods can plan paths with high localizability (which describes the capability of acquiring reliable localization). By following these paths, the robot can access the sensor streams that facilitate more accurate location estimation results by the localization algorithms. However, most of these methods require prior knowledge and struggle to adapt to unseen scenarios or dynamic changes. To overcome these limitations, we propose a novel approach for localizability-enhanced navigation via deep reinforcement learning in dynamic human environments. Our proposed planner automatically extracts geometric features from 2D laser data that are helpful for localization. The planner learns to assign different importance to the geometric features and encourages the robot to navigate through areas that are helpful for laser localization. To facilitate the learning of the planner, we suggest two techniques: (1) an augmented state representation that considers the dynamic changes and the confidence of the localization results, which provides more information and allows the robot to make better decisions, (2) a reward metric that is capable to offer both sparse and dense feedback on behaviors that affect localization accuracy. Our method exhibits significant improvements in lost rate and arrival rate when tested in previously unseen environments.
更多查看译文
关键词
deep reinforcement learning,dynamic human environments,localizability-enhanced
AI 理解论文
溯源树
样例
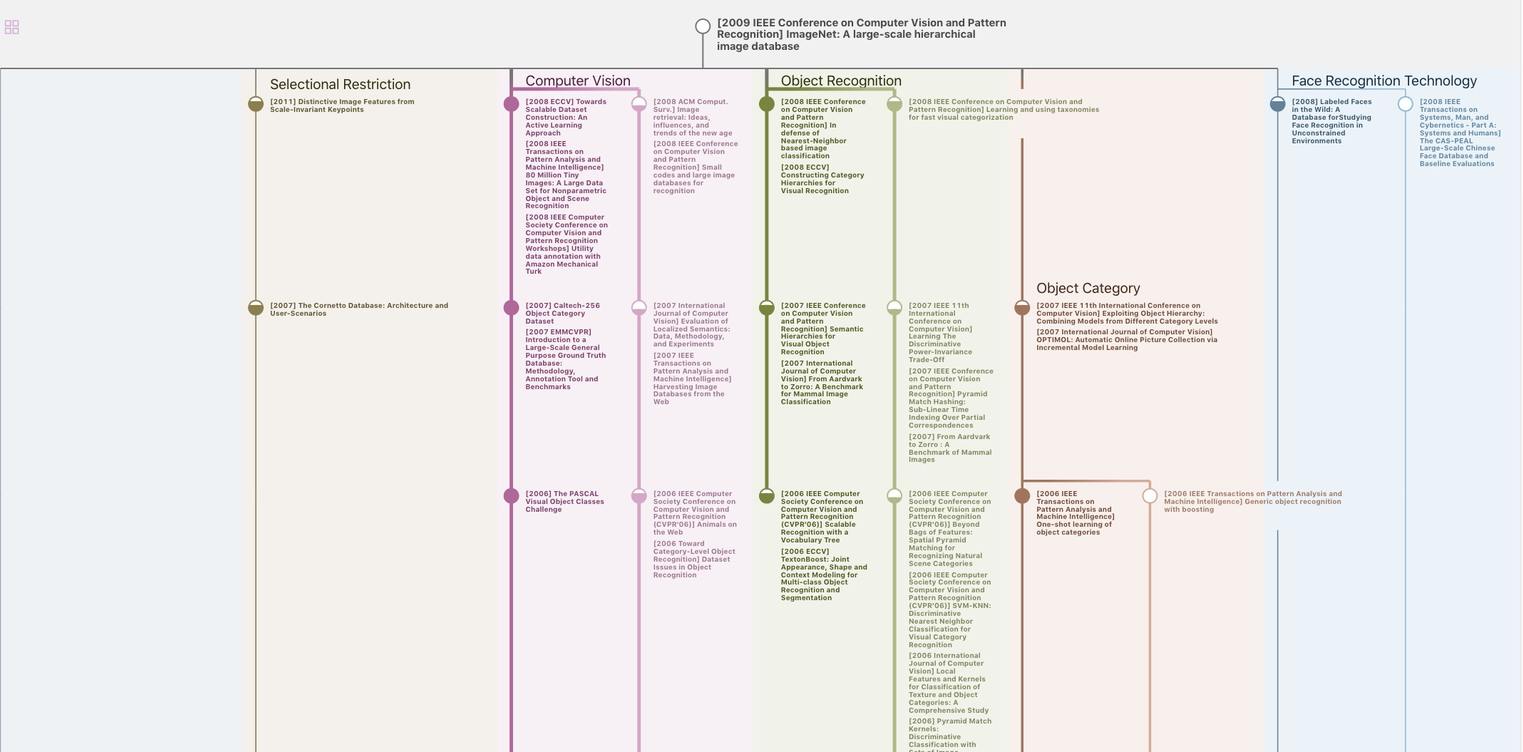
生成溯源树,研究论文发展脉络
Chat Paper
正在生成论文摘要